Unsupervised Tree Extraction in Embedding Spaces for Taxonomy Induction
WI/IAT(2021)
摘要
Exposing latent structure (graph, tree...) of data is a major challenge to deal with the web of data. Today's embedding techniques incorporate any data source (noisy graphs, item similarities, plain text) into continuous vector spaces that are typically used as input to classifier. In this work, we are dealing with the opposite task: finding structures (taxonomies) from embedded data. We provide an original unsupervised methodology for taxonomy induction by directly searching for graph structures preserving pairwise distances between items. Contrary to the state-of-the-art (SOTA), our approach does not require to train classifiers; it is also more versatile as it can be applied to any embedding (eg. word embedding, similarity embedding like space-time local embedding...). On standard benchmarks and metrics, our approach yields SOTA performance. As another contribution, we propose better evaluation metrics for taxonomy induction, leveraging graph kernel similarities and edit distance, showing that the structures of our predicted taxonomies are significantly closer to the ground-truth than SOTA solutions.
更多查看译文
关键词
Unstructured/Structured Data, Hyperbolic Embedding, Tree extraction, Taxonomy Induction
AI 理解论文
溯源树
样例
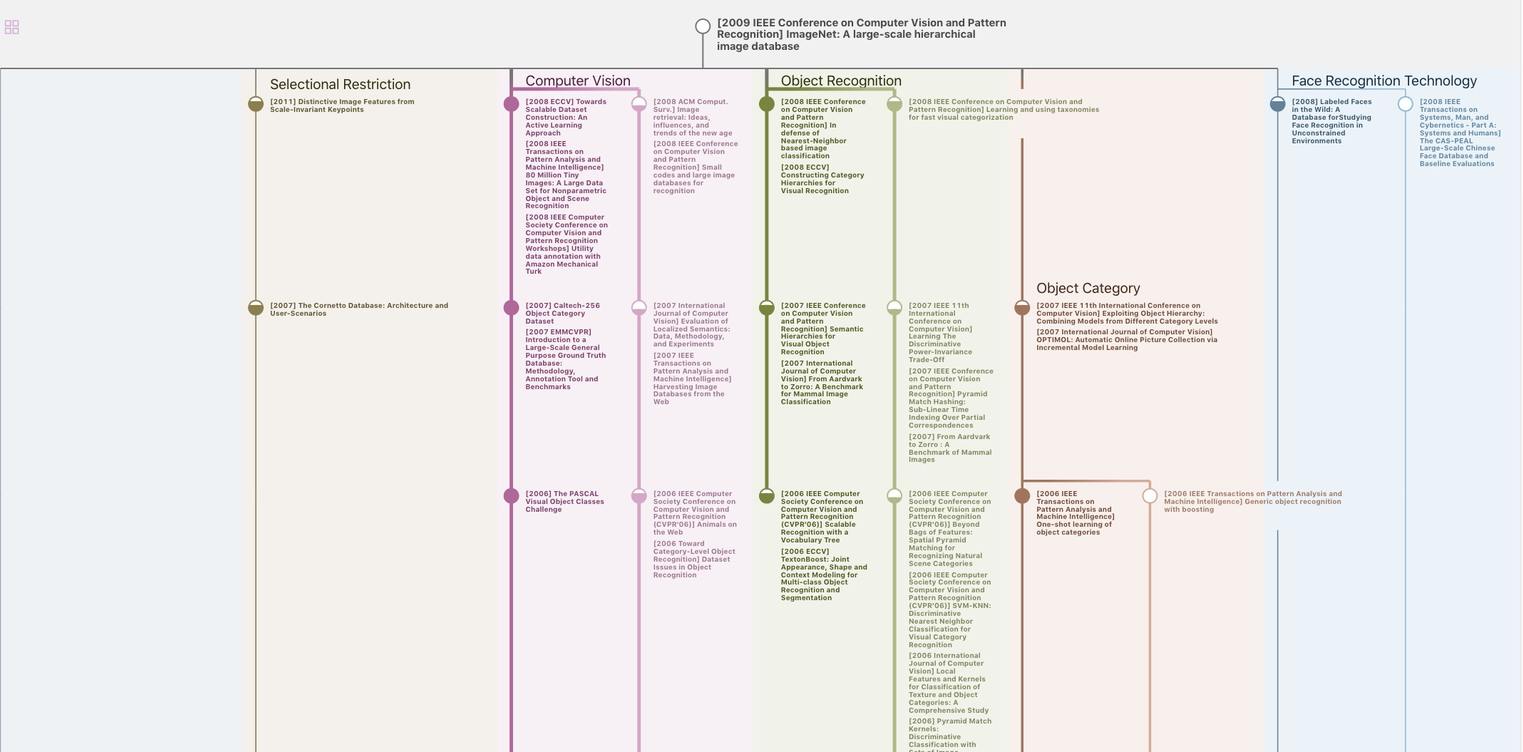
生成溯源树,研究论文发展脉络
Chat Paper
正在生成论文摘要