Long-term Characterization of Political Communications on Social Media
WI/IAT(2021)
摘要
Social media play an important role in shaping political discourse, creating a public sphere that enables discussions, debates, and deliberations. Aware of this importance, politicians use social media for self-promotion and as a means of influencing people and votes. As an example of this assertion, in 2018, Brazilians democratically elected for president the far-right candidate Jair Bolsonaro. One of the most surprising feats of this outcome is that his party, PSL, had almost no television time. His victory was only possible because of his supporters’ engagement and activism on social media platforms, such as Twitter, Facebook, and WhatsApp. In this context, politicians need to decide how to communicate with their voters to build their reputations. While some politicians only share professional communications about their political agenda and activities, others prefer a more non-political and informal approach, sharing communications about the most varied subjects, such as religion, sports, and their families. Others, however, misuse platforms by spreading political messages that violate policies and circumvent electoral laws. Aware of these problems, I propose the LOCPOC a methodology to characterize the communication of Brazilian politicians over years in terms of the amount of political and non-political messages they post. The methodology is robust to concept drifts over time, requiring few new labeled messages each year. From the classified messages, I was able to characterize the communication of politicians over time and identified new findings: (i) Brazilian congresspeople changed their communication behavior over time; (ii) concept drifts occurred during important events in Brazilian politics; (iii) the explosive rise of the right seen just before the 2018 elections; (iv) a broader and more evenly distributed right-wing participation than the left-wing, and, finally, (v) the increase of public engagement over time.
更多查看译文
关键词
political communication, text classification, concept-drift, neural networks
AI 理解论文
溯源树
样例
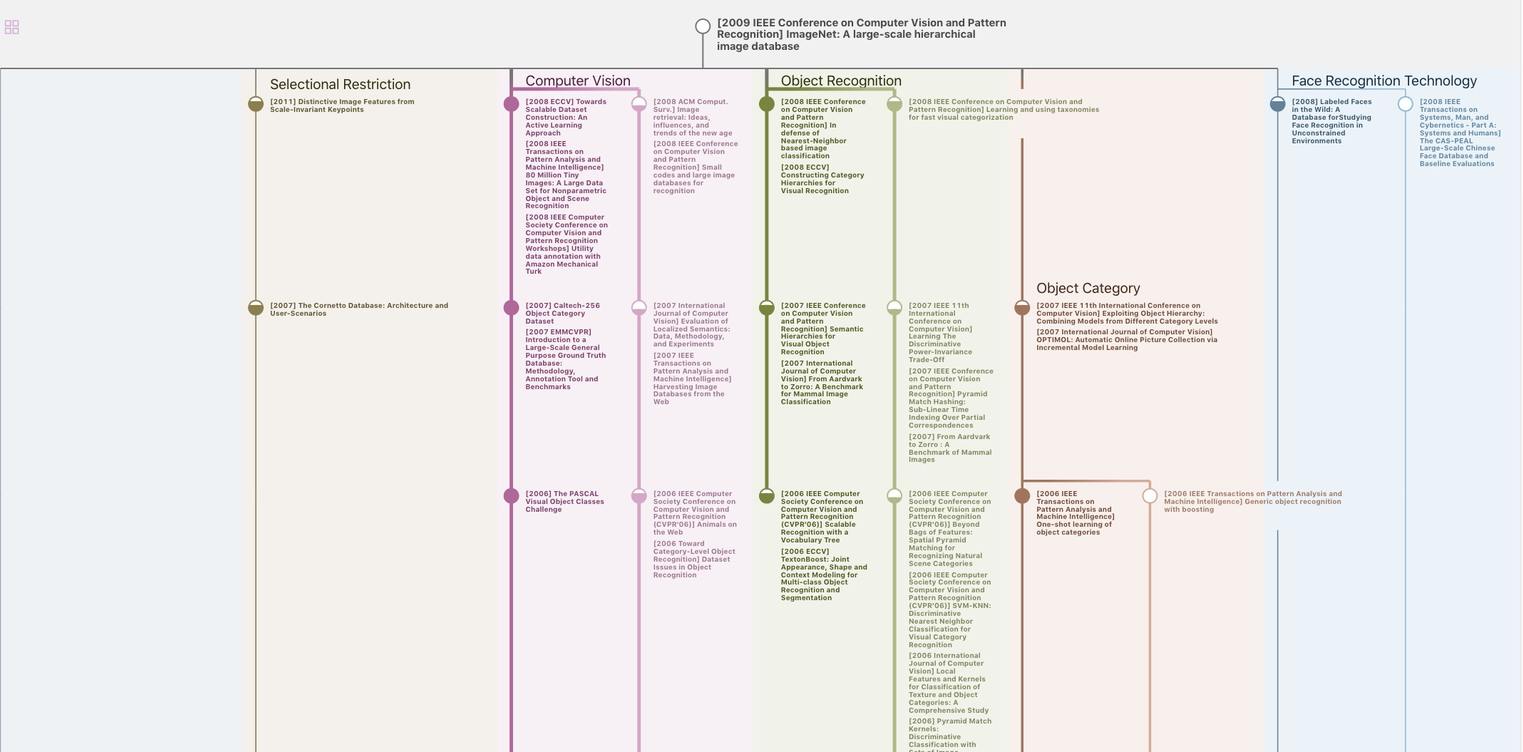
生成溯源树,研究论文发展脉络
Chat Paper
正在生成论文摘要