Few-shot learning for modeling cyber physical systems in non-stationary environments
Neural Comput. Appl.(2022)
摘要
This paper proposes a modeling scheme for cyber physical systems operating in non-stationary, small data environments. Unlike the traditional modeling logic, we introduce the few-shot learning paradigm, the operation of which is based on quantifying both similarities and dissimilarities. As such, we designed a suitable change detection mechanism able to reveal previously unknown operational states, which are incorporated in the dictionary online. We elaborate on spectrograms extracted from high-resolution ultrasound depth sensor timeseries, while the backbone of the proposed method is a Siamese Neural Network. The experimental scenario considers data representing liquid containers for fuel/water when the following five operational states are present: normal , accident , breakdown , sabotage , and cyber-attack . Thorough experiments were carried out assessing every aspect of the present framework and demonstrating its efficacy even when very few samples per class are available. In addition, we propose a probabilistic data selection scheme facilitating one-shot learning. Last but not least, responding to the wide requirement for interpretable AI, we explain the obtained predictions by examining the layer-wise activation maps.
更多查看译文
关键词
Cyber-physical systems,Cybersecurity,Few-shot learning,Deep learning,Online learning,Fault diagnosis,Cyber-attacks
AI 理解论文
溯源树
样例
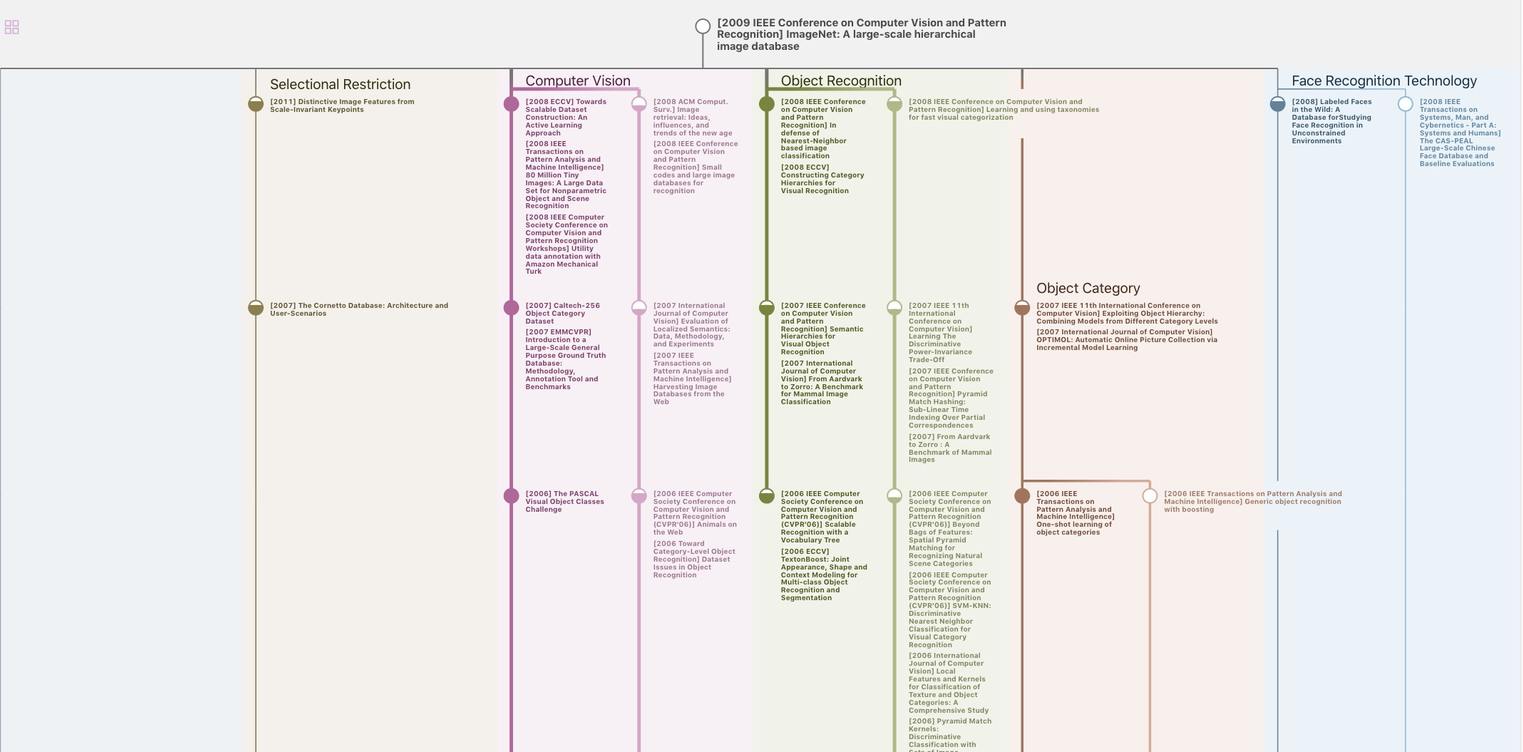
生成溯源树,研究论文发展脉络
Chat Paper
正在生成论文摘要