Remote Sensing Image Fusion Based on Morphological Convolutional Neural Networks with Information Entropy for Optimal Scale
SENSORS(2022)
摘要
Remote sensing image fusion is a fundamental issue in the field of remote sensing. In this paper, we propose a remote sensing image fusion method based on optimal scale morphological convolutional neural networks (CNN) using the principle of entropy from information theory. We use an attentional CNN to fuse the optimal cartoon and texture components of the original images to obtain a high-resolution multispectral image. We obtain the cartoon and texture components using sparse decomposition-morphological component analysis (MCA) with an optimal threshold value determined by calculating the information entropy of the fused image. In the sparse decomposition process, the local discrete cosine transform dictionary and the curvelet transform dictionary compose the MCA dictionary. We sparsely decompose the original remote sensing images into a texture component and a cartoon component at an optimal scale using the information entropy to control the dictionary parameter. Experimental results show that the remote sensing image fusion method proposed in this paper can effectively retain the information of the original image, improve the spatial resolution and spectral fidelity, and provide a new idea for image fusion from the perspective of multi-morphological deep learning.
更多查看译文
关键词
remote sensing image fusion, morphological component analysis, information entropy, deep learning, multi-scale
AI 理解论文
溯源树
样例
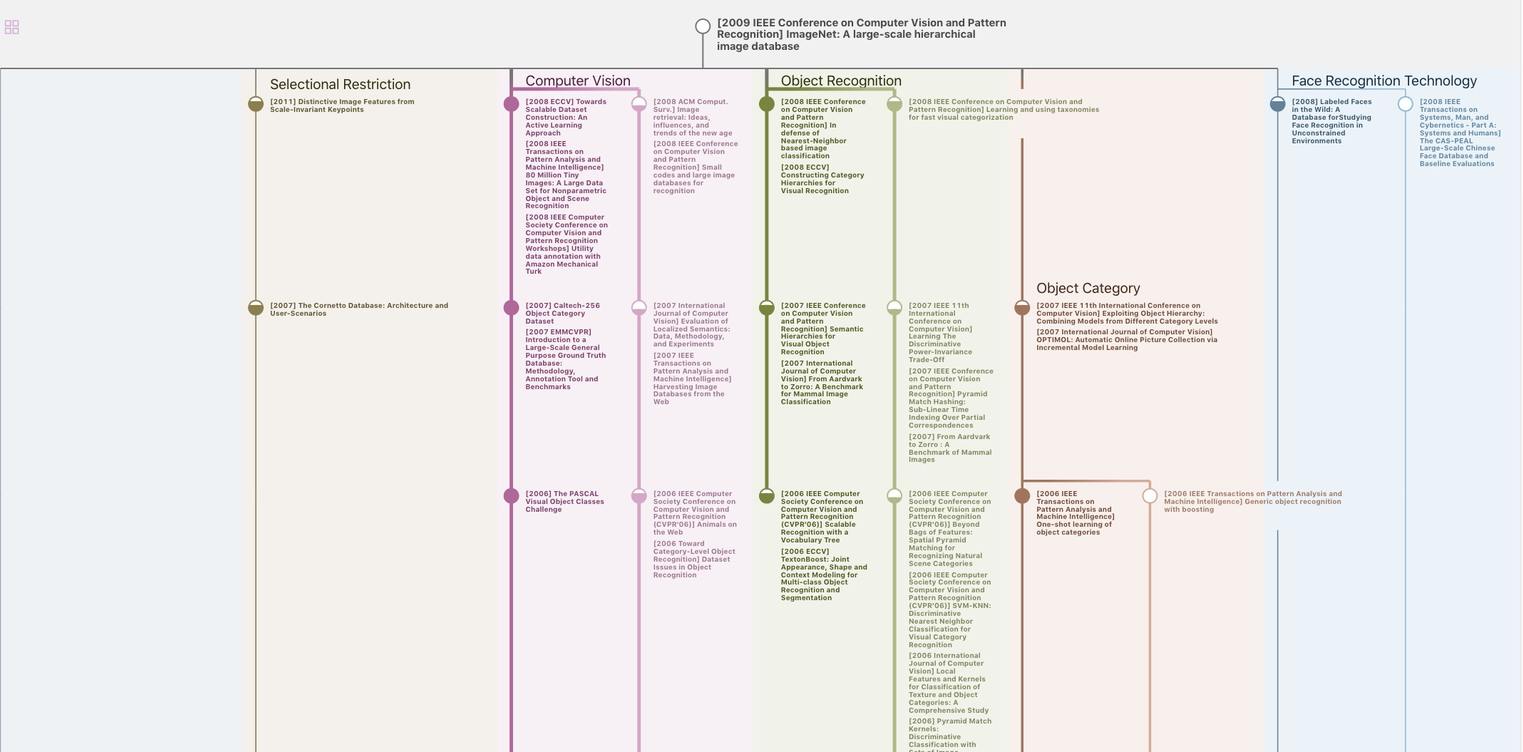
生成溯源树,研究论文发展脉络
Chat Paper
正在生成论文摘要