Evaluation of Effective Class-Balancing Techniques for CNN-Based Assessment of Aphanomyces Root Rot Resistance in Pea (Pisum sativum L.)
SENSORS(2022)
摘要
Aphanomyces root rot (ARR) is a devastating disease that affects the production of pea. The plants are prone to infection at any growth stage, and there are no chemical or cultural controls. Thus, the development of resistant pea cultivars is important. Phenomics technologies to support the selection of resistant cultivars through phenotyping can be valuable. One such approach is to couple imaging technologies with deep learning algorithms that are considered efficient for the assessment of disease resistance across a large number of plant genotypes. In this study, the resistance to ARR was evaluated through a CNN-based assessment of pea root images. The proposed model, DeepARRNet, was designed to classify the pea root images into three classes based on ARR severity scores, namely, resistant, intermediate, and susceptible classes. The dataset consisted of 1581 pea root images with a skewed distribution. Hence, three effective data-balancing techniques were identified to solve the prevalent problem of unbalanced datasets. Random oversampling with image transformations, generative adversarial network (GAN)-based image synthesis, and loss function with class-weighted ratio were implemented during the training process. The result indicated that the classification F1-score was 0.92 +/- 0.03 when GAN-synthesized images were added, 0.91 +/- 0.04 for random resampling, and 0.88 +/- 0.05 when class-weighted loss function was implemented, which was higher than when an unbalanced dataset without these techniques were used (0.83 +/- 0.03). The systematic approaches evaluated in this study can be applied to other image-based phenotyping datasets, which can aid the development of deep-learning models with improved performance.
更多查看译文
关键词
plant breeding, disease identification, deep learning, generative adversarial networks
AI 理解论文
溯源树
样例
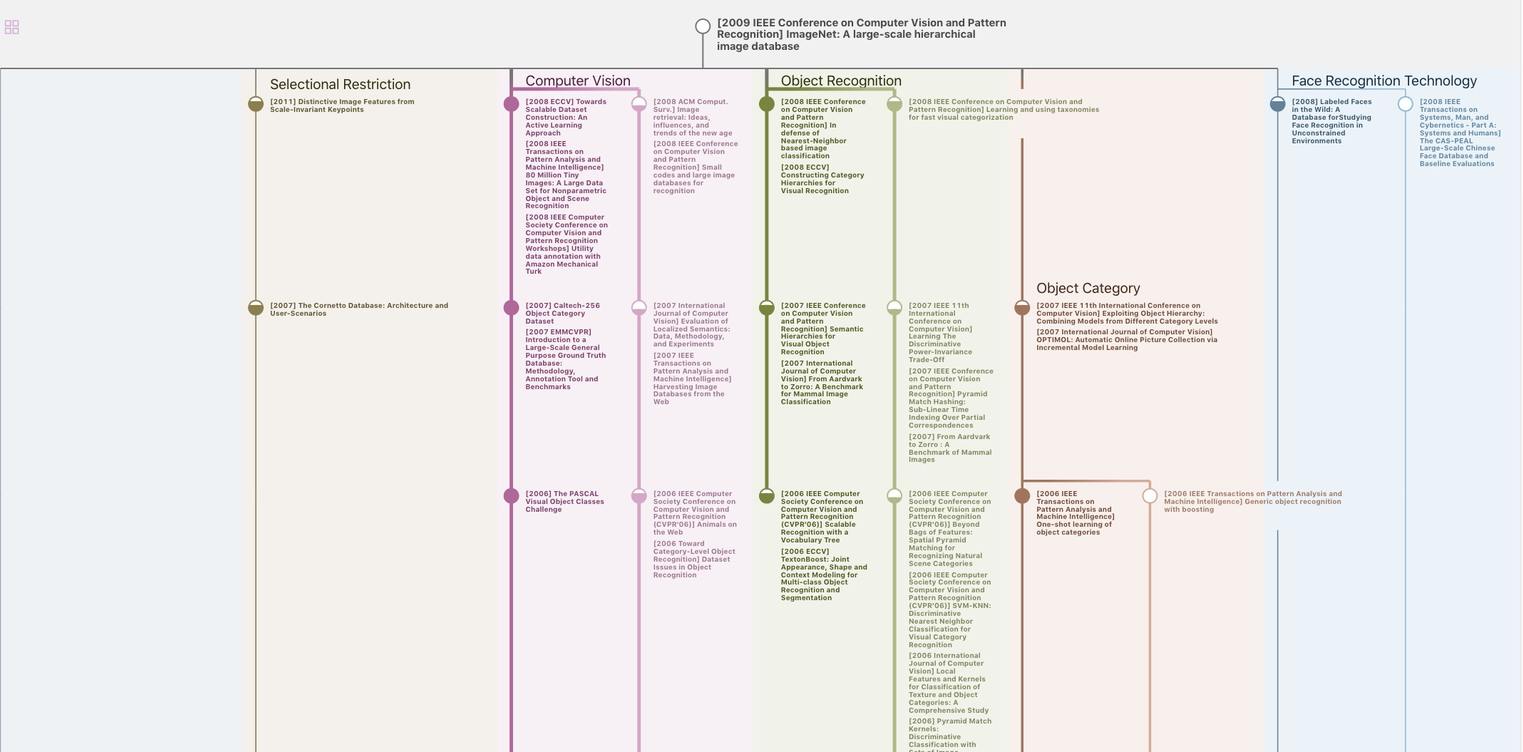
生成溯源树,研究论文发展脉络
Chat Paper
正在生成论文摘要