Co-consensus semi-supervised multi-view learning with orthogonal non-negative matrix factorization
Information Processing & Management(2022)
摘要
Semi-supervised multi-view learning has recently achieved appealing performance with the consensus relation between samples. However, in addition to the relation between samples, the relation between samples and their assemble centroid is also important to the learning. In this paper, we propose a novel model based on orthogonal non-negative matrix factorization, which allows exploring both the consensus relations between samples and between samples and their assemble centroid. Since this model utilizes more consensus information to guide the multi-view learning, it can lead to better performance. Meanwhile, we theoretically derive a proposition about the equivalency between the partial orthogonality and the full orthogonality. Based on this proposition, the orthogonality constraint and the label constraint are simultaneously implemented in the proposed model. Experimental evaluations on five real-world datasets show that our approach outperforms the state-of-the-art methods, where the improvement is 6% average in terms of ARI index.
更多查看译文
关键词
Multi-view learning,Semi-supervised learning,Orthogonal constraint,Orthogonal non-negative matrix factorization,Consensus
AI 理解论文
溯源树
样例
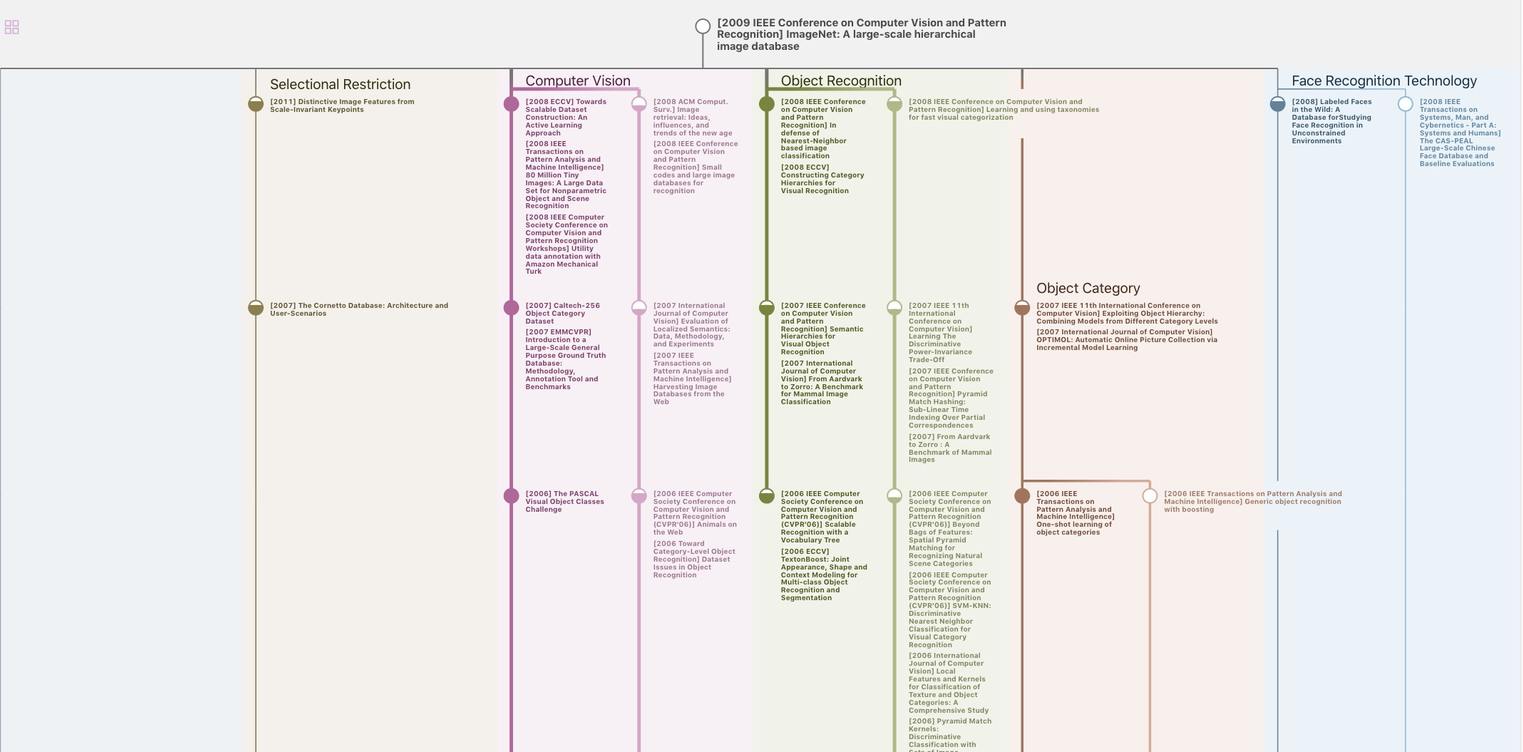
生成溯源树,研究论文发展脉络
Chat Paper
正在生成论文摘要