Enhance COVID-19 Mortality Prediction with Human Mobility Trend and Medical Information.
IEEE International Conference on High Performance Computing and Communications(2021)
摘要
In this work, we study national and state-level COVID-19 pandemic data in the United States with the help of human mobility trend data and auxiliary medical information. We analyze and compare various state-of-the-art time-series prediction techniques. We assess a spatio-temporal graph neural network model which forecasts the pandemic course by utilizing a hybrid deep learning architecture and human mobility data. Nodes in the graph represent the state-level deaths due to COVID-19 at any particular time point, edges represent the human mobility trend and temporal edges correspond to node attributes across time. We also study statistical modeling and machine learning techniques for mortality prediction in the United States. We evaluate these techniques on both state and national level COVID-19 data in the United States and claim that the SARIMAX and GCN-LSTM model generated forecast values using exogenous hospital information variables can enrich the underlying model to improve the prediction accuracy at both levels. Our best machine learning models perform 50% and 60% better than the baseline on an average on the national level and state-level data, respectively, while the statistical models perform 63% and 42% better.
更多查看译文
关键词
COVID-19,Time-Series Analysis,Graph Neural Networks,SARIMAX,Medical Information
AI 理解论文
溯源树
样例
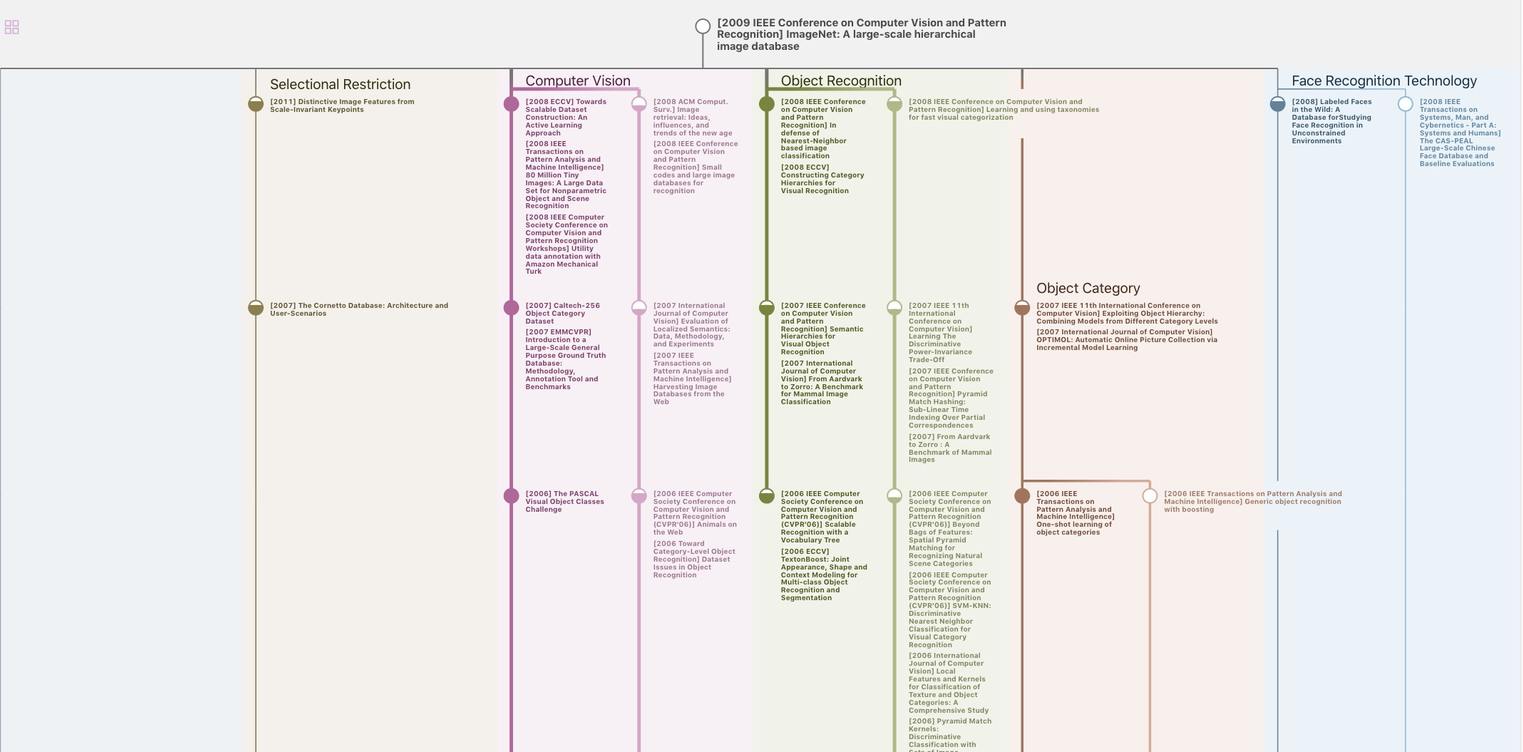
生成溯源树,研究论文发展脉络
Chat Paper
正在生成论文摘要