Shapelet Optimization in Beam Optics Analyzer
2022 IEEE International Conference on Plasma Science (ICOPS)(2022)
摘要
Calabazas Creek Research extends the shapelet method to include optimization of the power density distribution. The shapelet method constructs the surface density from a set of particles impinging on 3D surfaces. This technique allows more accurate representation of the beam power density, which is critical in X-ray target or e-beam lithography. The density is commonly obtained by interpolation of particle position to the mesh. The typical approach is to increase the number of particles and meshes to satisfy the required accuracy. The shapelet method achieves the desired accuracy for a particular spot size by adaptively increasing the order of interpolation without increasing the mesh size or number of particles. Shapelets are constructed from a Gaussian and Hermite polynomials. For a given set of particles, one can increase the order of the interpolation, and the approximation approaches to the series of delta functions, which is not the desired smooth distribution. We can re-simulate with a higher number of particles and denser global mesh, and re-interpolate with shapelets to obtain higher accuracy. However, this will incur extra costly simulations. We propose to optimize on a goal function based on the residual of the power, a scale 2-vector and the orders of the interpolation. We will present optimization results by the Nelder-Mead method.
更多查看译文
关键词
power density distribution,shapelet method,surface density,beam power density,e-beam lithography,interpolation,particle position,particular spot size,mesh size,desired smooth distribution,global mesh,Nelder-Mead method,shapelet optimization,beam optics analyzer,Calabazas Creek research,X-ray target,Gaussian polynomials,Hermite polynomials
AI 理解论文
溯源树
样例
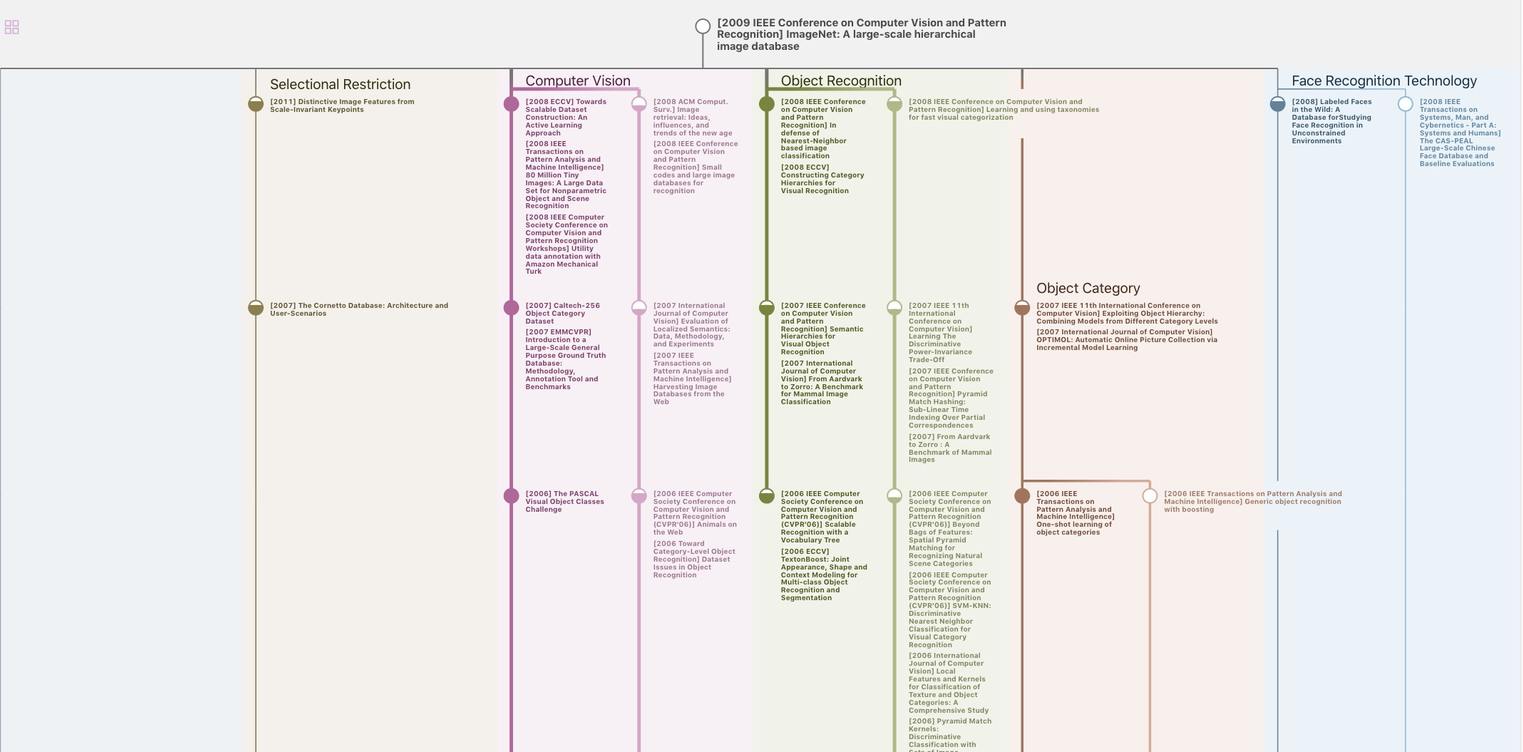
生成溯源树,研究论文发展脉络
Chat Paper
正在生成论文摘要