Discovering clustering patterns from e-counter data streams
ISPRS Annals of the Photogrammetry, Remote Sensing and Spatial Information Sciences(2022)
摘要
Abstract. Clustering data streams has gained popularity in recent years due to their potential of generating relevant information for planning building automation, evaluating energy efficiency scenarios, and simulating emergency protocols in indoor spaces. In this paper, a Data Stream Affinity Propagation (DSAP) clustering algorithm is proposed for analyzing indoor localization data generated from e-counters systems. The data streams are a sequence of potentially infinite and non-stationary data points, arriving continuously where random access to the data is not feasible and storing all the arriving data is impractical. The DSAP model is developed based on a two-phase approach (i.e., online and offline clustering phases) using the landmark time window model. It is non-parametric in the sense of not requiring any prior knowledge about the number of clusters and their respective labels. The validation and performance of the DSAP model is evaluated using real-world data streams from a behavioural experiment aimed at finding new spatio-temporal patterns in stair usage due to an educational intervention campaign.
更多查看译文
关键词
clustering patterns,streams,e-counter
AI 理解论文
溯源树
样例
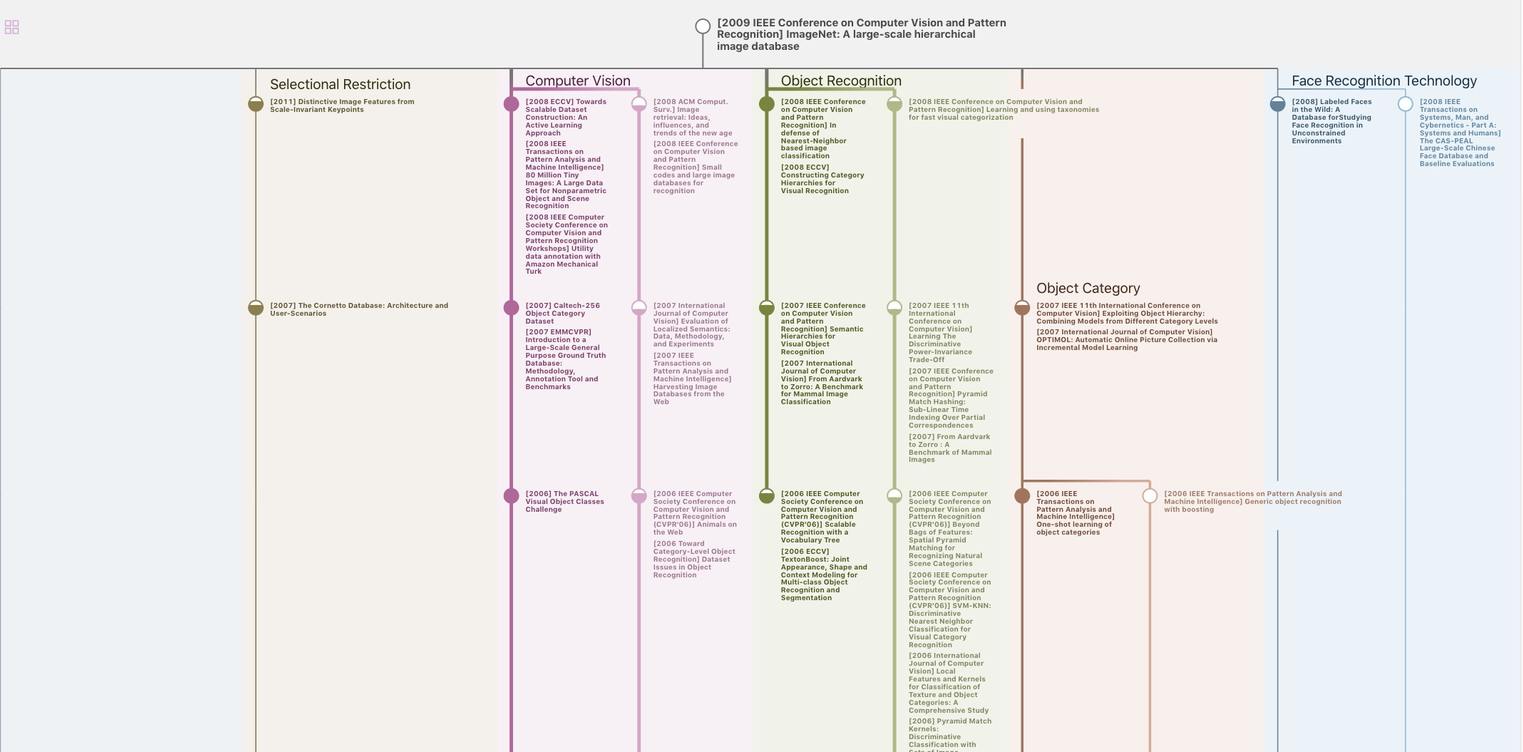
生成溯源树,研究论文发展脉络
Chat Paper
正在生成论文摘要