Data augmentation in extreme ultraviolet lithography simulation using convolutional neural network
Journal of Micro/Nanopatterning, Materials, and Metrology(2022)
摘要
Background: In the previous work, we developed a convolutional neural network (CNN), which reproduces the results of the rigorous electromagnetic (EM) simulations in a small mask area. The prediction time of CNN was 5000 times faster than the calculation time of EM simulation. We trained the CNN using 200,000 data, which were the results of EM simulation. Although the prediction time of CNN was very short, it took a long time to build a huge amount of the training data. Especially when we enlarge the mask area, the calculation time to prepare the training data becomes unacceptably long.Aim: Reducing the calculation time to prepare the training data.Approach: We apply data augmentation technique to increase the number of training data using limited original data. The training data of our CNN are the diffraction amplitudes of mask patterns. Assuming a periodic boundary condition, the diffraction amplitudes of the shifted or flipped mask pattern can be easily calculated using the diffraction amplitudes of the original mask pattern.Results: The number of training data after the data augmentation is multiplied by 200 from 2500 to 500,000. Using a large amount of training data, the validation loss of CNN was reduced. The accuracy of CNN with augmented data is verified by comparing the CNN predictions with the results of EM simulation.Conclusions: Data augmentation technique is applied to the diffraction amplitude of the mask pattern. The data preparation time is reduced by a factor of 200. Our CNN almost reproduces the results of EM simulation. In this work, the mask patterns are restricted to line and space patterns. It is a challenge to build several CNNs for specific mask patterns or ultimately a single CNN for arbitrary mask patterns.
更多查看译文
关键词
lithography simulation,neural network,extremely ultraviolet mask
AI 理解论文
溯源树
样例
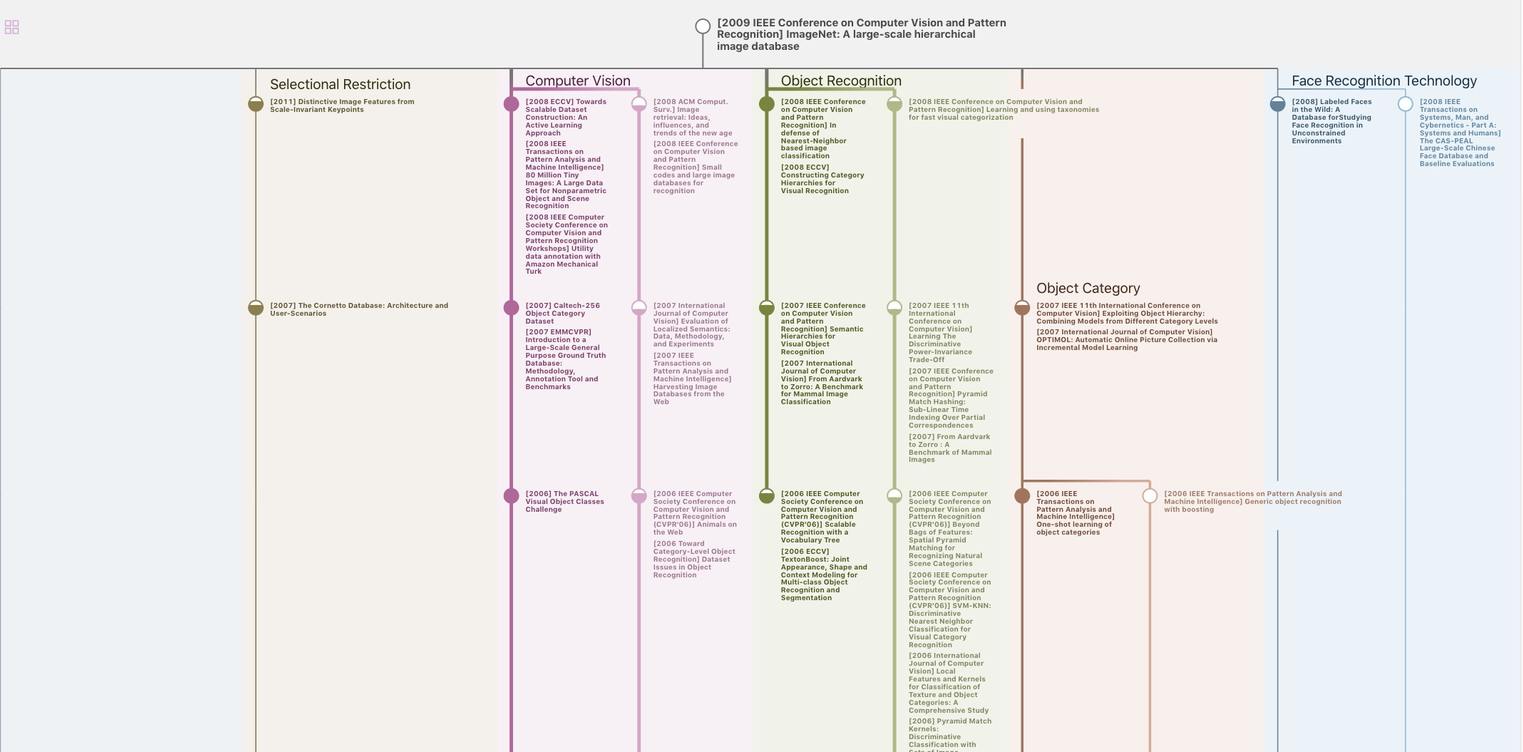
生成溯源树,研究论文发展脉络
Chat Paper
正在生成论文摘要