Regularized nonlinear regression for simultaneously selecting and estimating key model parameters: Application to head-neck position tracking
Engineering Applications of Artificial Intelligence(2022)
摘要
In system identification, estimating parameters of a biomechanical model using limited observations results in poor identifiability. To cope with this issue, we propose a new method to simultaneously select and estimate sensitive parameters as key model parameters while fixing the remaining parameters to a set of typical values. The problem is formulated as a nonlinear least-squares estimator with L1-regularization on the deviation of parameters from a set of typical values. In addition, a modified optimization approach is introduced to find the solution to the formulated problem. As a result, we provided consistency and oracle properties of the proposed estimator as a theoretical foundation. To show the effectiveness of the proposed method, we conducted simulation and experimental studies. In the simulation study, the proposed Lasso performed significantly better than the ordinary L1-regularization methods in terms of the bias and variance of the parameter estimates. The experimental study presented an application identifying a biomechanical parametric model of a head position tracking task for ten human subjects from limited data. Compared with the variance of the parameter estimates from nonlinear ordinary least-squares regression, that of parameter estimates from the proposed Lasso decreased by 96% using the simulated data. Using the real-world data, the variance of estimated parameters decreased by 71%. In addition, the proposed method kept variance accounted for (VAF) at 83% and was 54 times faster than the ordinary Lasso using a standard simplex-based optimization algorithm.
更多查看译文
关键词
System identification,Nonlinear regression,L1-regularization,Identifiability,Head-neck model
AI 理解论文
溯源树
样例
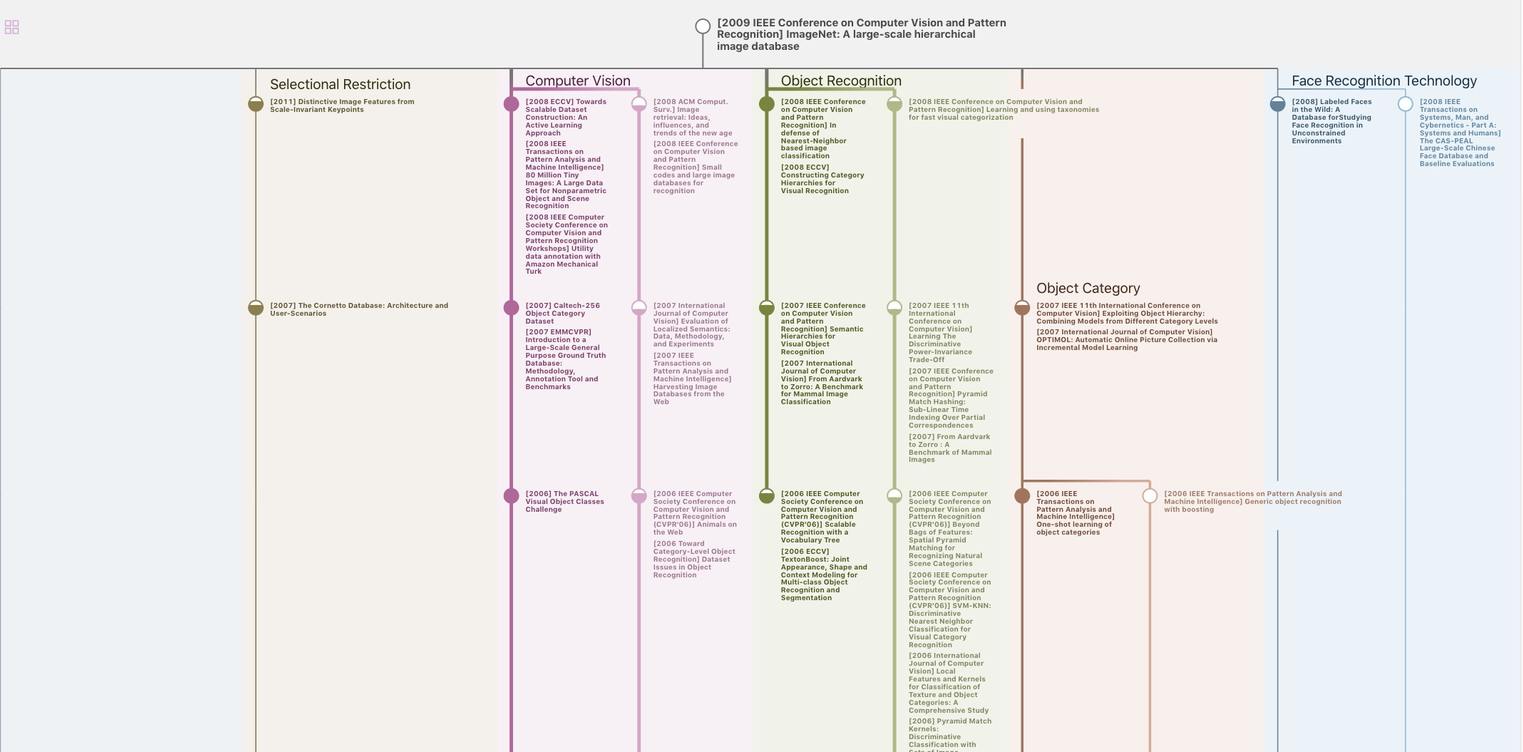
生成溯源树,研究论文发展脉络
Chat Paper
正在生成论文摘要