Machine learning assisted prediction of charge transfer properties in organic solar cells by using morphology-related descriptors
Nano Research(2022)
摘要
Charge transfer and transport properties are crucial in the photophysical process of exciton dissociation and recombination at the donor/acceptor (D/A) interface. Herein, machine learning (ML) is applied to predict the charge transfer state energy ( E CT ) and identify the relationship between E CT and intermolecular packing structures sampled from molecular dynamics (MD) simulations on fullerene- and non-fullerene-based systems with different D/A ratios ( R DA ), oligomer sizes, and D/A pairs. The gradient boosting regression (GBR) exhibits satisfactory performance ( r = 0.96) in predicting E CT with π-packing related features, aggregation extent, backbone of donor, and energy levels of frontier molecular orbitals. The charge transport property affected by π-packing with different R DA has also been investigated by space-charge-limited current (SCLC) measurement and MD simulations. The SCLC results indicate an improved hole transport of non-fullerene system PM6/Y6 with R DA of 1.2:1 in comparison with the 1:1 counterpart, which is mainly attributed to the bridge role of donor unit in Y6. The reduced energetic disorder is correlated with the improved miscibility of polymer with R DA increased from 1:1 to 1.2:1. The morphology-related features are also applicable to other complicated systems, such as perovskite solar cells, to bridge the gap between device performance and microscopic packing structures.
更多查看译文
关键词
charge transfer,charge transport,packing modes,machine learning,organic solar cells
AI 理解论文
溯源树
样例
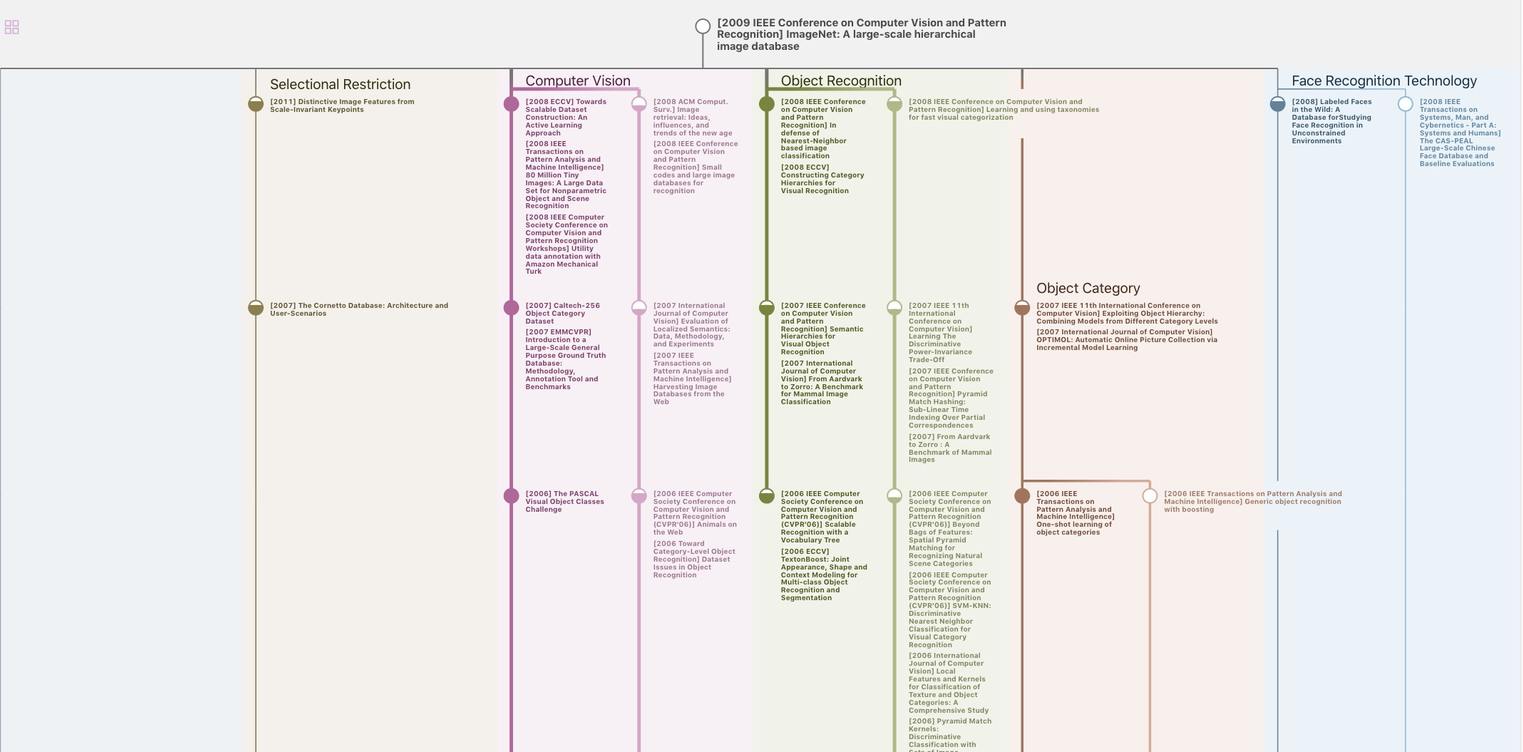
生成溯源树,研究论文发展脉络
Chat Paper
正在生成论文摘要