Short-Term Electric Demand Forecasting for Power Systems using Similar Months Approach based SARIMA
2019 IEEE International Conference on Power, Electrical, and Electronics and Industrial Applications (PEEIACON)(2019)
摘要
Short term load forecasting is essential for energy management of any power system. Over the years, a variety of methods such as exponential smoothing, autoregressive integrated moving average (ARIMA) and Artificial Neural Networks (ANNs) have been proposed in the literature. All of these methods have been used in conjunction with similar days approach to bolster the prediction accuracy. However, there is no general consensus in literature on what is the best approach to group 365 days of a year into different categories. In contrast, similar months approach to forecast electricity demand has received little attention although it alleviates many of the efforts in sorting similar days into separate groups. In this paper, we propose a similar months approach based seasonal ARIMA (SARIMA) to forecast electric demand both on a national and a household scale. Through comparison with similar days based approach on similar tasks, we demonstrate that this method is a viable method for short term electric load forecasting of power systems as well as in building energy management applications.
更多查看译文
关键词
short-term electric demand forecasting,power system,SARIMA,seasonal autoregressive integrated moving average,short term electric load forecasting,building energy management,artificial neural networks
AI 理解论文
溯源树
样例
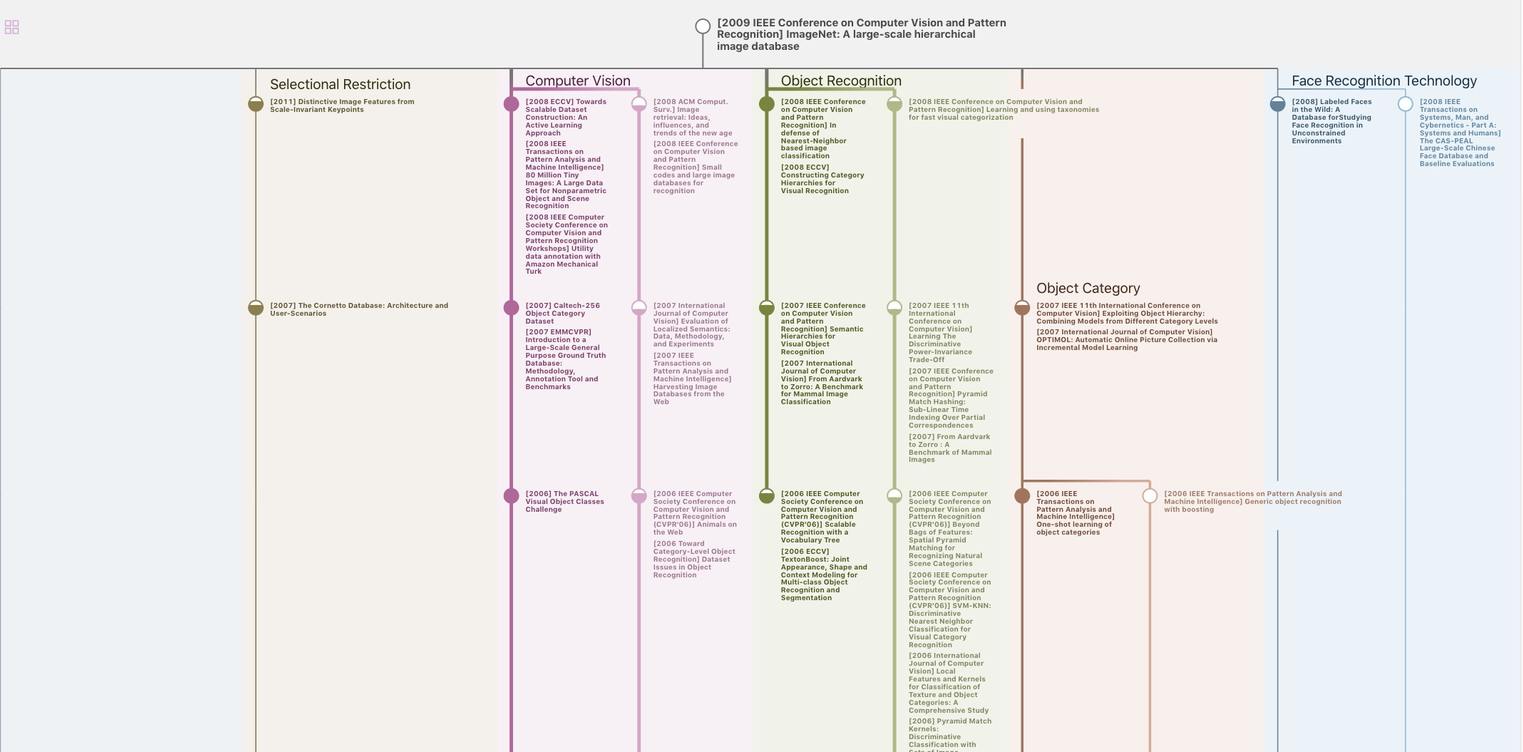
生成溯源树,研究论文发展脉络
Chat Paper
正在生成论文摘要