The application of neural networks to classify dolphin echolocation clicks
biorxiv(2022)
摘要
Passive acoustic monitoring (PAM) is a common approach to monitor marine mammal populations, for species of dolphins, porpoises and whales that use sound for navigation, feeding and communication. PAM produces large datasets which benefit from the application of machine learning algorithms to automatically detect and classify the vocalisations of these animals. We present a deep learning approach for the classification of dolphins’ echolocation clicks into two species groups in an environment with high background noise. We compare the use of Convolutional Neural Networks (CNN) and Recurrent Neural Network (RNN), in which we feed the models the raw waveform data and spectrograms. We show that both models perform well, with the highest performance achieved by a CNN fed with spectrograms (F1 score 97 %) and an RNN fed with raw data (F1 score 96%) fitted with Gated Recurrent Units (GRU). We recommend the use of such models to classify echolocation clicks in marine environments where background noise levels exhibit high spatial and temporal variance. In particular, the RNN showed excellent performance, while being fed with raw data, in terms of reduced processing time and storage. Deep learning automatically extracts effective features from the raw waveform in the training process through multiple layers of the model, without the need to rely on feature extraction in a separate pre-processing step.
### Competing Interest Statement
The authors have declared no competing interest.
更多查看译文
关键词
dolphin,neural networks
AI 理解论文
溯源树
样例
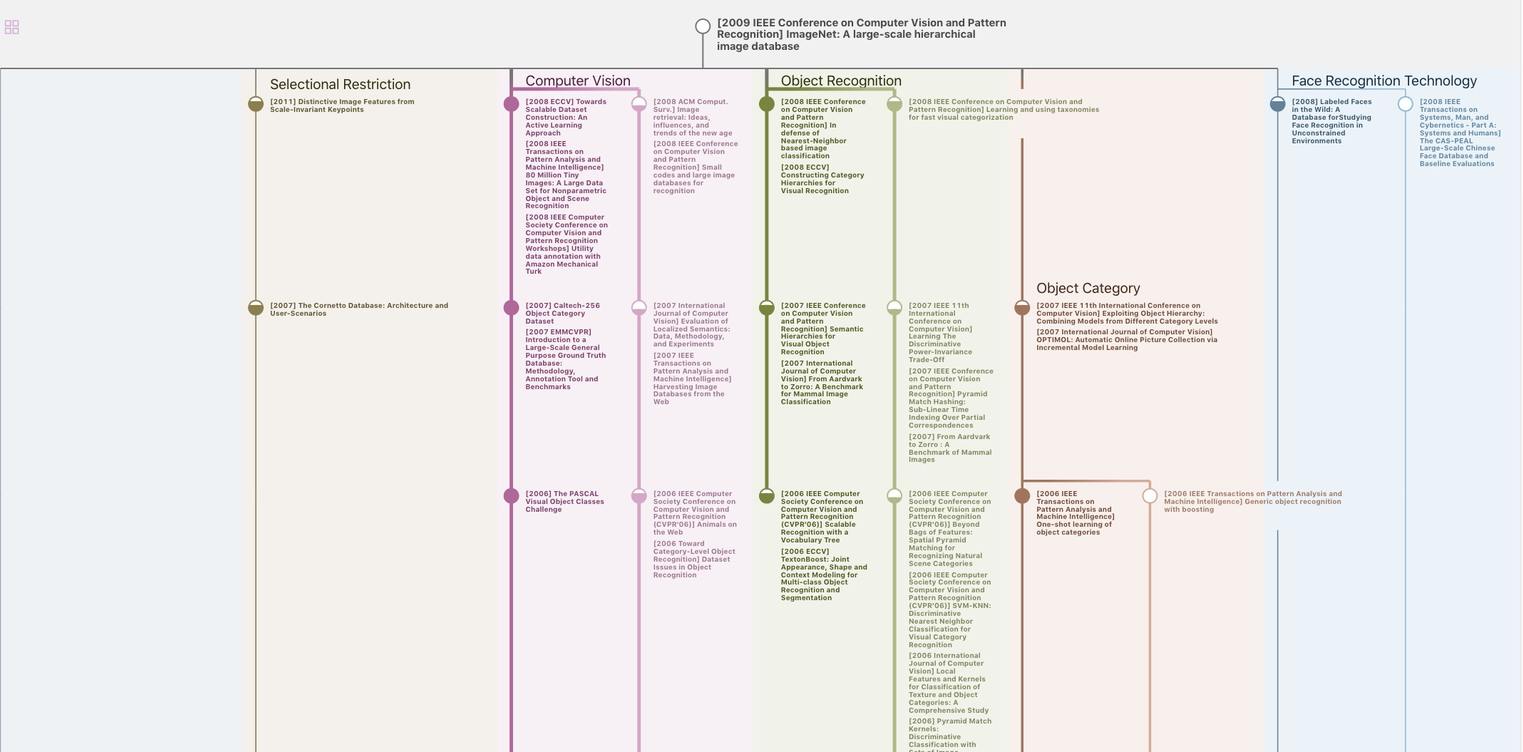
生成溯源树,研究论文发展脉络
Chat Paper
正在生成论文摘要