A deep partial adversarial transfer learning network for cross-domain fault diagnosis of machinery
2022 Prognostics and Health Management Conference (PHM-2022 London)(2022)
摘要
Recently, the deep transfer learning-based methods have been widely applied in intelligent fault diagnosis of modern manufacturing equipment in real-industrial scenarios, which are capable of identifying the health conditions of unlabeled target samples under various working conditions. In transfer learning- based intelligent fault diagnosis, the source diagnostic knowledge, which is usually extracted by supervised learning approaches, is transferred and reused in related target fault identification tasks. However, the tremendous success of these transfer learning methods is mainly achieved in the field of close-set cross-domain fault diagnosis. But in practical applications, a partial cross-domain scenario is more common and difficult, where the health conditions of the target domain are less than that of the source domain. To address this issue, a deep partial adversarial transfer learning network (PATLN) based on convolutional neural networks and adversarial training is proposed. Experiments on a public rolling element bearing dataset verify the effectiveness of the PATLN method.
更多查看译文
关键词
adversarial training,cross-domain fault diagnosis,partial transfer learning
AI 理解论文
溯源树
样例
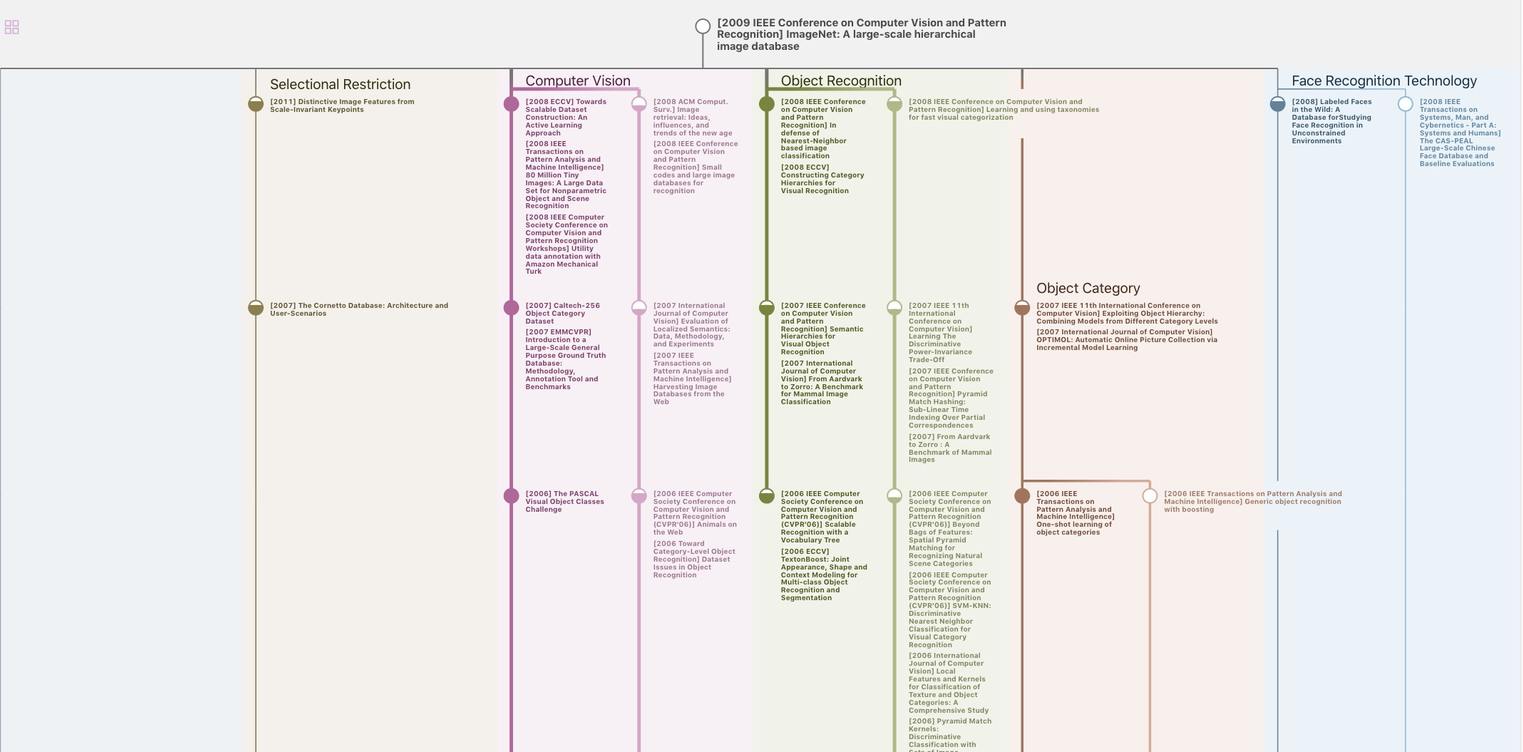
生成溯源树,研究论文发展脉络
Chat Paper
正在生成论文摘要