Sensitivity Study of Mini-Batch Size on a Long Short-Term Memory Network for Predicting Shell-tocore Ratio of Microencapsulated Phase Change Materials
2022 IEEE International Conference on Prognostics and Health Management (ICPHM)(2022)
摘要
Microencapsulated phase change materials are being studied for applications for thermal energy storage in concentrated solar fields. During fabrication, the thickness of the encapsulation cannot be readily measured for real-time control. Therefore, a machine learning network, specifically a Long Short-Term Memory network, is being developed to estimate the ratio of the shell thickness to core radius based on a one second temperature history. The mini-batch size determines how often the algorithm weights are updated during network training, and shuffle indicates whether the training data is shuffled during training. A general factorial design is used to analyze the effects of varying minibatch size and shuffle, along with the shell-to-core ratio, on the root mean square error (RMSE) of the response from the Long Short-Term Memory network. It was found that the network performed better for smaller shell-to-core ratios (less than 0.6) and had the lowest RMSE when the mini-batch size was 128, but larger mini-batch sizes also performed similarly. The minimum RMSE found was 0.00501.
更多查看译文
关键词
long short-term memory,machine learning,mini-batch size,phase change materials
AI 理解论文
溯源树
样例
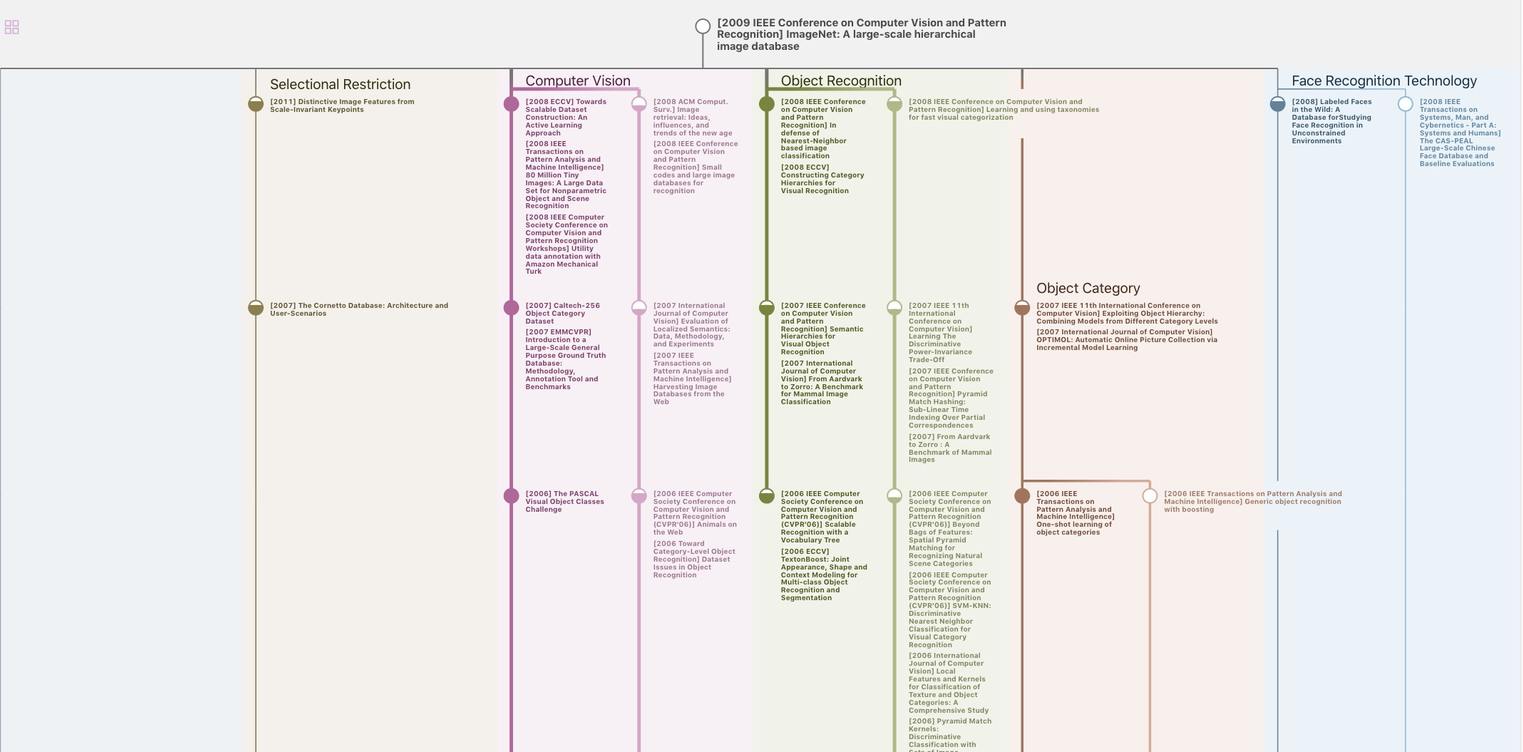
生成溯源树,研究论文发展脉络
Chat Paper
正在生成论文摘要