Tracking influenza a virus infection in the lung from hematological data with machine learning
bioRxiv(2022)
摘要
The tracking of pathogen burden and host responses with minimal-invasive methods during respiratory infections is central for monitoring disease development and guiding treatment decisions. Utilizing a standardized murine model of respiratory Influenza A virus (IAV) infection, we developed and tested different supervised machine learning models to predict viral burden and immune response markers, i . e . cytokines and leukocytes in the lung, from hematological data. We performed independently in vivo infection experiments to acquire extensive data for training and testing purposes of the models. We show here that lung viral load, neutrophil counts, cytokines like IFN-γ and IL-6, and other lung infection markers can be predicted from hematological data. Furthermore, feature analysis of the models shows that blood granulocytes and platelets play a crucial role in prediction and are highly involved in the immune response against IAV. The proposed in silico tools pave the path towards improved tracking and monitoring of influenza infections and possibly other respiratory infections based on minimal-invasively obtained hematological parameters.
### Competing Interest Statement
The authors have declared no competing interest.
更多查看译文
关键词
influenza,machine learning,virus infection,hematological data,lung
AI 理解论文
溯源树
样例
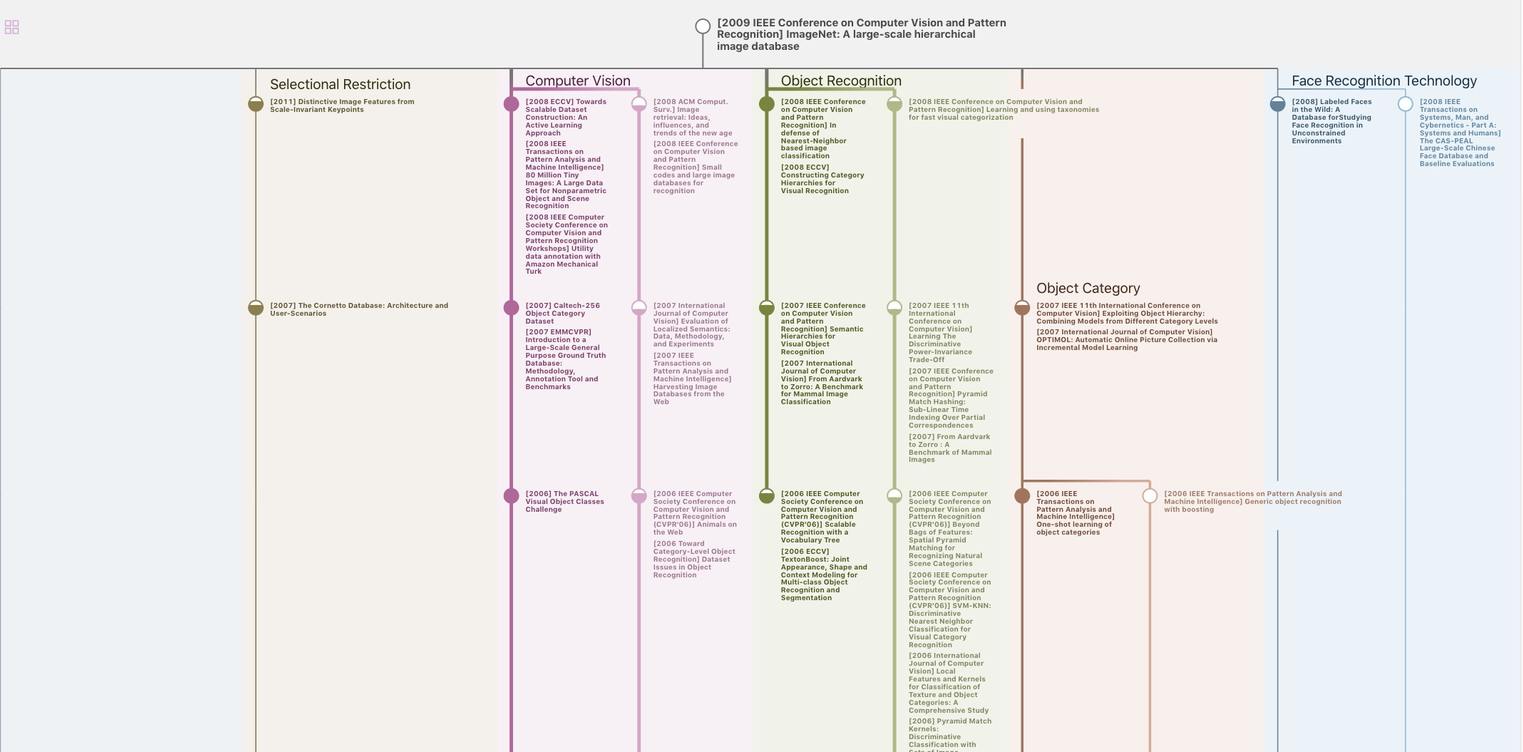
生成溯源树,研究论文发展脉络
Chat Paper
正在生成论文摘要