Hyperspectral Image Denoising With Weighted Nonlocal Low-Rank Model and Adaptive Total Variation Regularization.
IEEE Trans. Geosci. Remote. Sens.(2022)
摘要
Hyperspectral image (HSI) is always corrupted by various types of noises during image capturing, such as Gaussian noise, stripe noise, deadline noise, impulse noise, and more. Such complicated noise significantly degrades imaging quality and thus limits the performance of downstream vision tasks. Current HSI denoising methods tackle this problem by modeling either the spectral-spatial prior of HSI or the noise characteristic of HSI, and few works consider the two aspects simultaneously. In this article, we propose a new HSI denoising method by simultaneously modeling the HSI prior and the HSI noise characteristic. Specifically, we first utilize the nonindependent and identically distributed (non-i.i.d.) mixture of Gaussian (MoG) assumptions to characterize the complex noise, which corresponds to optimizing a weighted fidelity function. Second, we exploit HSI's nonlocal similarity and spatial-spectral correlation priors by applying a nonlocal low-rank model. Third, we design an adaptive edge-preserving total variation (TV) regularization term to characterize the nonlocal smooth property of HSI. Finally, we propose a new denoising model and develop an effective alternating direction method of multipliers (ADMM) algorithm to solve it. Extensive experiments on simulated data and real data substantiate the superiority of the proposed method beyond state-of-the-art.
更多查看译文
关键词
Adaptive spatial-spectral total variation (ASSTV),hyperspectral image (HSI) denoising,non independent and identically distributed (non-i.i.d.) noise modeling,non local low-rank model
AI 理解论文
溯源树
样例
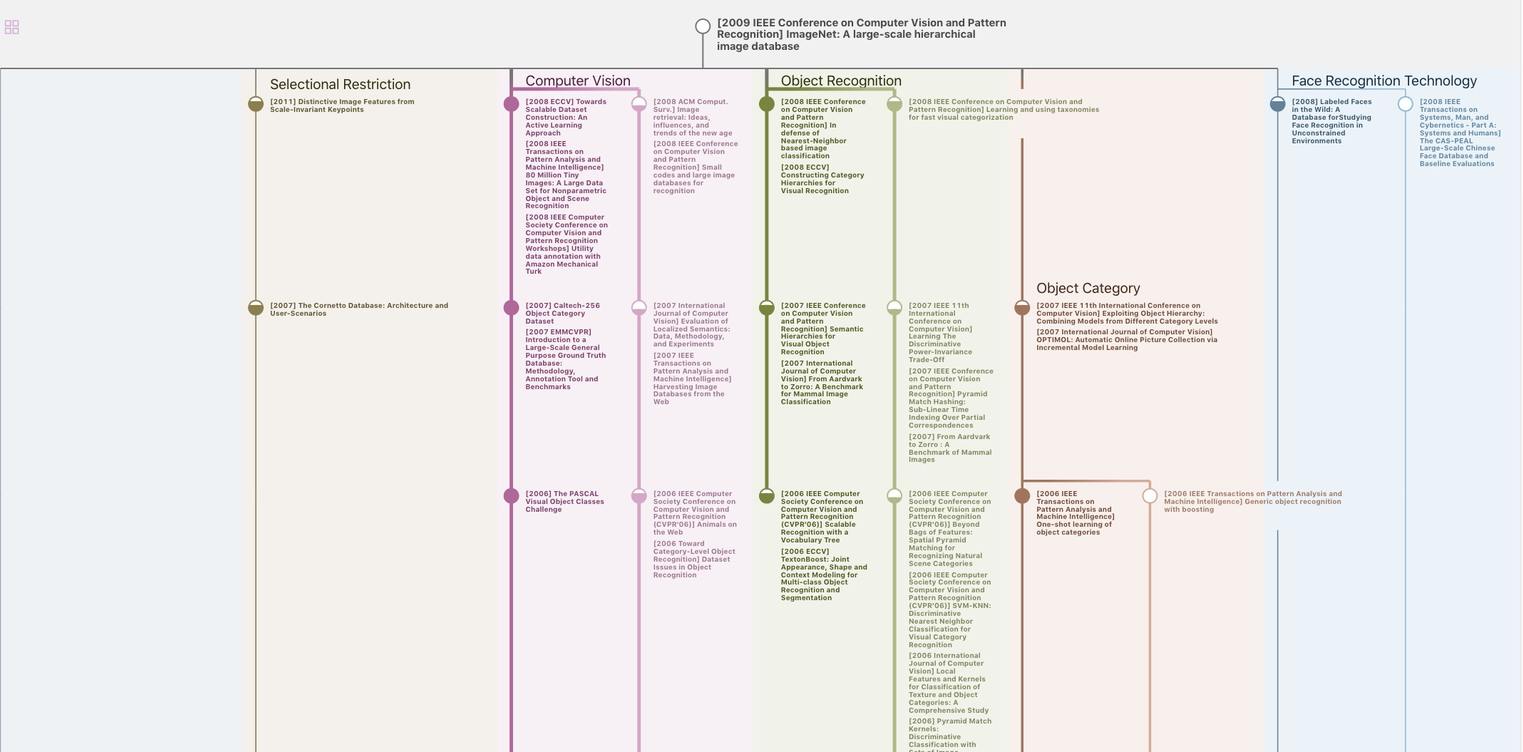
生成溯源树,研究论文发展脉络
Chat Paper
正在生成论文摘要