Smart ensemble machine learner with hyperparameter-free for predicting bond capacity of FRP-to-concrete interface: Multi-national data
Construction and Building Materials(2022)
摘要
Determining the bond capacity of a (fiber-reinforced polymer) FRP-to-concrete interface is important for the design task in repairing or rehabilitating concrete structures in infrastructure. This paper presents a novel framework for creating a hyperparameter-free ensemble model and, using an equilibrium optimizer (EO), demonstrates a radial basis function neural network (RBFNN) and least square support vector regression (LSVR). A new model, the metaheuristic-optimized neuron-vector machine inference model (MNVIM), was used for the accurate estimation of FRP-to-concrete interfacial bond capacity. The MNVIM was constructed using the EO algorithm to blend RBFNN and LSVR adaptively. In the MNVIM framework, the EO is involved in the learning phases of the RBFNN and the LSVR by giving an appropriate set of hyperparameters for the two constituent models, including the number of hidden neurons and the Gaussian impact value of RBFNN, as well as the regularization coefficient and kernel parameter. Simultaneously, EO adaptively adjusts linear combination weight values to blend the prediction values of RBFNN and LSVR. Statistical results from ten runs show that the newly developed model achieved the most desired evaluation criteria, namely, root mean square error (RMSE) (3.989 kN), mean absolute (MAE) (2.632 kN), mean absolute percentage error (MAPE) (15.56%), and R-square (0.839). The t-test statistical measurement further confirms that the prediction accuracy of MNVIM is significantly better than other artificial intelligence (AI) models and sixteen common mathematical approaches. These analytical results indicate that MNVIM is a promising tool for estimating bonding strength; thus, it significantly increases construction safety in concrete element repair/rehabilitation-related engineering design using FRP.
更多查看译文
关键词
Fiber-reinforced polymer,Bonding strength,Structure repair/rehabilitation ensemble model,Neural network,Metaheuristic optimization,Support vector regression
AI 理解论文
溯源树
样例
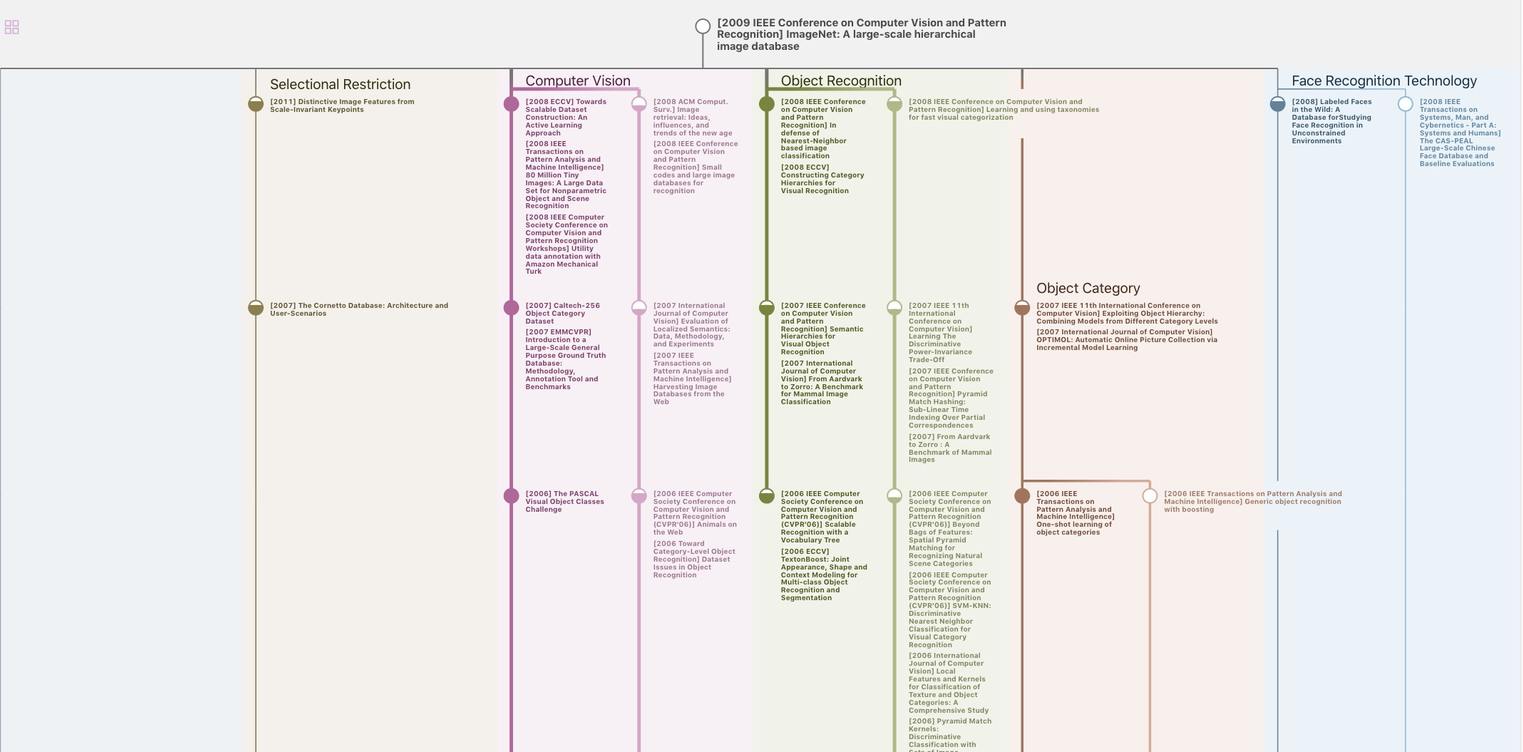
生成溯源树,研究论文发展脉络
Chat Paper
正在生成论文摘要