Real-time deep learning method for automated detection and localization of structural defects in manufactured products
Computers & Industrial Engineering(2022)
摘要
In recent years, artificial intelligence has been applied in the industry to automate various vision-based applications, such as monitoring structural defects in manufactured products. For industrial inspections, the automatic detection and localization of defective parts from product images ensure quality while avoiding waste of labor and materials. To this end, this paper introduces a two-branch neural network architecture that comprises detector and localizer components, where the former identifies the presence of defects, while the latter defines the region of interest for each defective area detected in the product structure. In both cases, the underlying strategy lies in a semi-supervised setting observing only defect-free product images, enabling the learning of the correct product structure that can be used to identify every kind of defect independently from position, color, or shape. The effectiveness of the proposed method is evaluated on the MVTec-AD industrial benchmark comprising different object and texture categories, considering the common state-of-the-art AUROC and SSIM metrics for the evaluation of anomaly detection and localization, respectively. Ablation studies varying the number of layers are performed on all the architecture components, founding that the presented two-branch network is consistently robust among all classes achieving remarkable results, i.e., 98% for AUROC and 94% for SSIM. What is more, measuring the time required to detect and localize the defects, the trained network is run on the RPi4B as an embedded system to simulate a practical industrial setting with limited computational resources, demonstrating the applicability of the presented method in real scenarios.
更多查看译文
关键词
Defect detection,Region of interest,Deep learning,Convolutional auto-encoders,Industrial inspection
AI 理解论文
溯源树
样例
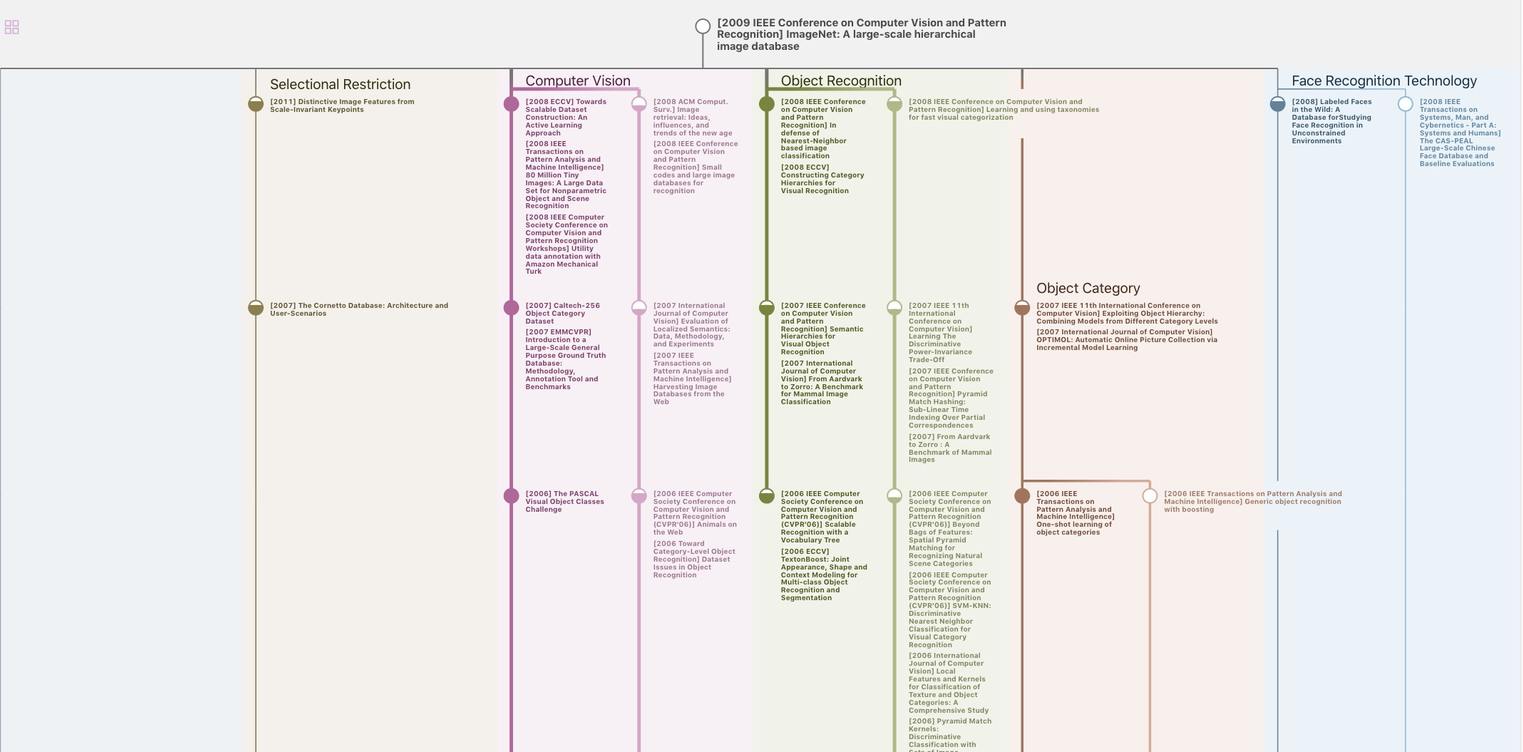
生成溯源树,研究论文发展脉络
Chat Paper
正在生成论文摘要