Neighborhood Structure Configuration Models
WWW 2023(2022)
摘要
We develop a new method to efficiently sample synthetic networks that preserve the d-hop neighborhood structure of a given network for any given d. The proposed algorithm trades off the diversity in network samples against the depth of the neighborhood structure that is preserved. Our key innovation is to employ a colored Configuration Model with colors derived from iterations of the so-called Color Refinement algorithm. We prove that with increasing iterations the preserved structural information increases: the generated synthetic networks and the original network become more and more similar, and are eventually indistinguishable in terms of centrality measures such as PageRank, HITS, Katz centrality and eigenvector centrality. Our work enables to efficiently generate samples with a precisely controlled similarity to the original network, especially for large networks.
更多查看译文
关键词
models,structure
AI 理解论文
溯源树
样例
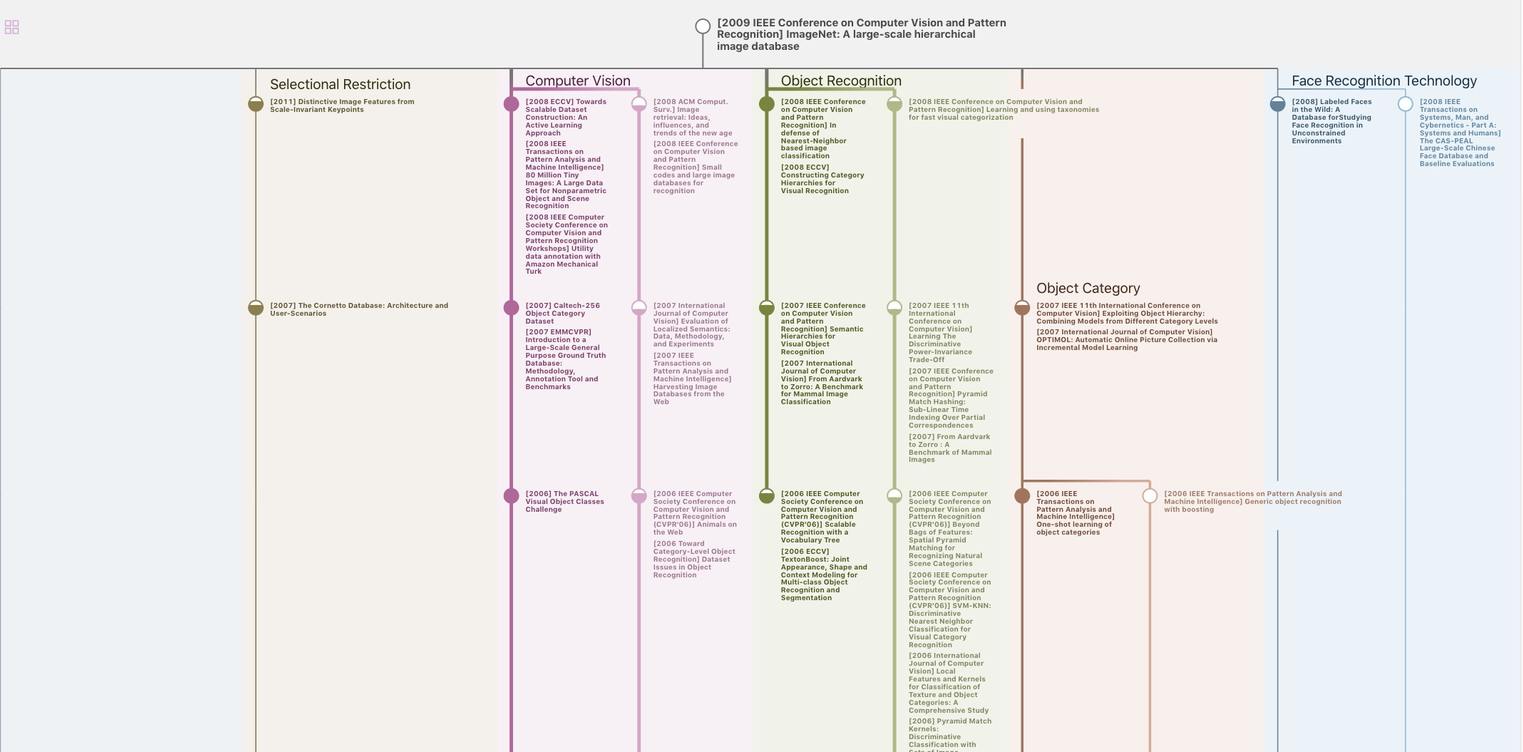
生成溯源树,研究论文发展脉络
Chat Paper
正在生成论文摘要