Personalized Federated Hypernetworks for Privacy Preservation in Multi-Task Reinforcement Learning
arxiv(2022)
摘要
Multi-Agent Reinforcement Learning currently focuses on implementations where all data and training can be centralized to one machine. But what if local agents are split across multiple tasks, and need to keep data private between each? We develop the first application of Personalized Federated Hypernetworks (PFH) to Reinforcement Learning (RL). We then present a novel application of PFH to few-shot transfer, and demonstrate significant initial increases in learning. PFH has never been demonstrated beyond supervised learning benchmarks, so we apply PFH to an important domain: RL price-setting for energy demand response. We consider a general case across where agents are split across multiple microgrids, wherein energy consumption data must be kept private within each microgrid. Together, our work explores how the fields of personalized federated learning and RL can come together to make learning efficient across multiple tasks while keeping data secure.
更多查看译文
关键词
microgrid clusters,energy demand response,transactive energy control,neural networks,multi-agent reinforcement learning,reinforcement learning,multi-task learning,transfer learning,hypernetworks,federated learning,personalized federated learning,microgrids
AI 理解论文
溯源树
样例
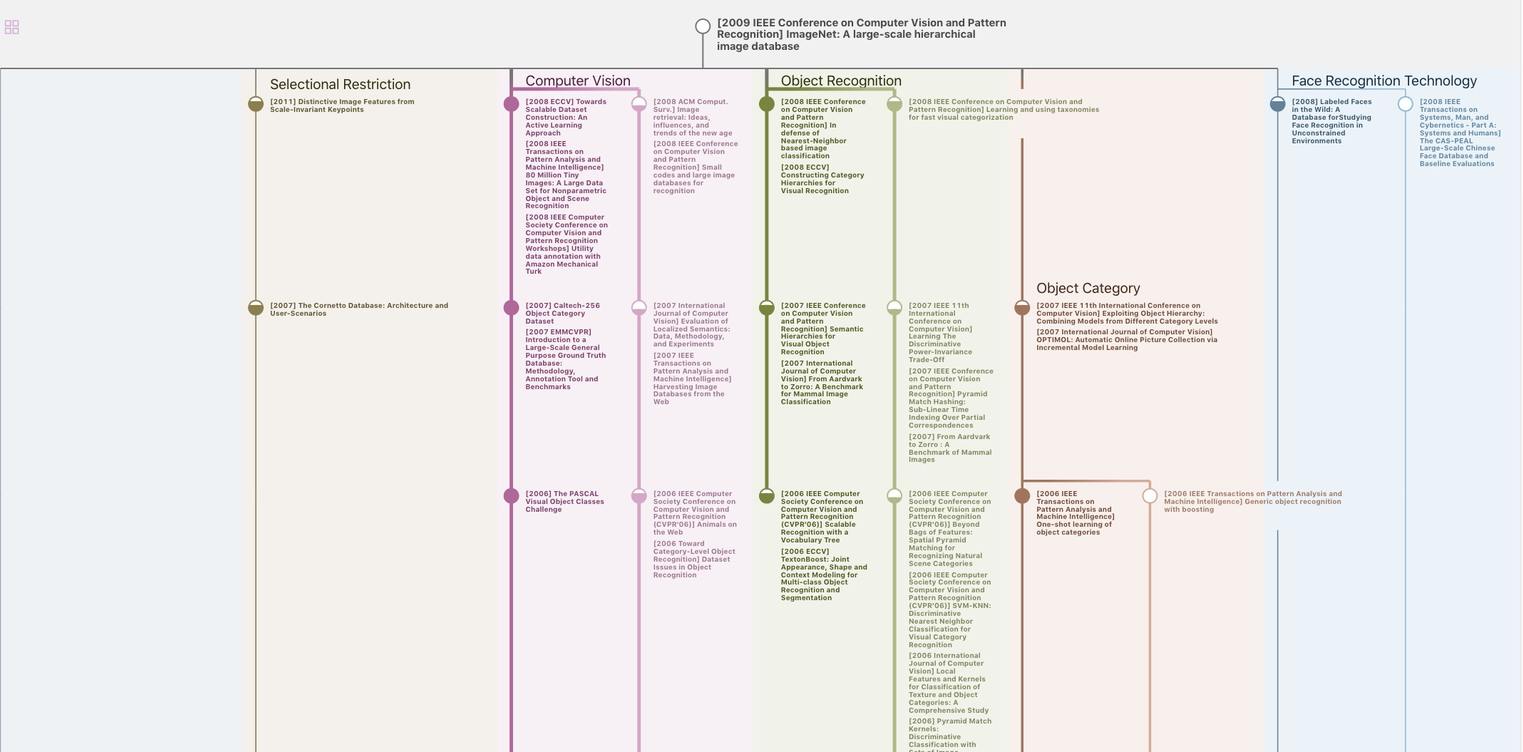
生成溯源树,研究论文发展脉络
Chat Paper
正在生成论文摘要