Explanations from Large Language Models Make Small Reasoners Better
arxiv(2022)
摘要
Integrating free-text explanations to in-context learning of large language models (LLM) is shown to elicit strong reasoning capabilities along with reasonable explanations. In this paper, we consider the problem of leveraging the explanations generated by LLM to improve the training of small reasoners, which are more favorable in real-production deployment due to their low cost. We systematically explore three explanation generation approaches from LLM and utilize a multi-task learning framework to facilitate small models to acquire strong reasoning power together with explanation generation capabilities. Experiments on multiple reasoning tasks show that our method can consistently and significantly outperform finetuning baselines across different settings, and even perform better than finetuning/prompting a 60x larger GPT-3 (175B) model by up to 9.5% in accuracy. As a side benefit, human evaluation further shows that our method can generate high-quality explanations to justify its predictions, moving towards the goal of explainable AI.
更多查看译文
关键词
small reasoners,large language models
AI 理解论文
溯源树
样例
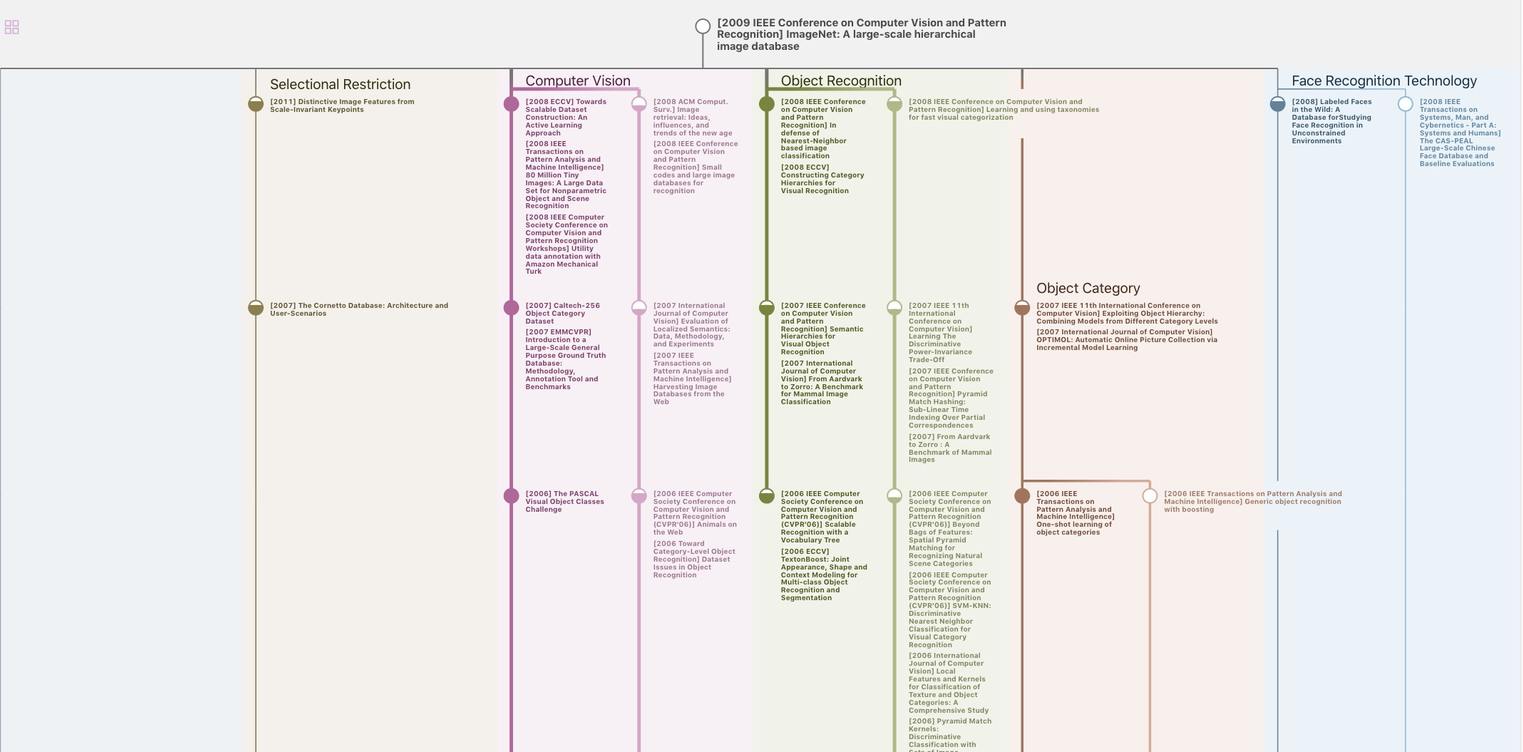
生成溯源树,研究论文发展脉络
Chat Paper
正在生成论文摘要