Multi-Agent Reinforcement Learning-Based Decentralized Spectrum Access in Vehicular Networks With Emergent Communication
IEEE Communications Letters(2023)
摘要
In this letter, we propose a novel decentralized spectrum access algorithm based on the multi-agent reinforcement learning (MARL) for cellular vehicle-to-everything (C-V2X) networks. The agents make decisions independently with the global objective of maximizing vehicle-to-infrastructure (V2I) users' sum throughput while meeting vehicle-to-vehicle (V2V) users' latency and reliability requirements. Specifically, to achieve better collaboration among agents we introduce the inter-agent communication mechanism into MARL. So, in the proposed algorithm each agent consists of an action selector module and a message generator module, i.e., the agents learn emergent communication via an additional dedicated channel to enable explicit collaboration, which helps with learning effective policies for channel access. Simulation results show the effectiveness of the proposed algorithm, in improving both V2I users' throughput and V2V users' packet delivery ratio.
更多查看译文
关键词
\boldsymbol C-V2X,decentralized spectrum access,multi-agent reinforcement learning,emergent communication
AI 理解论文
溯源树
样例
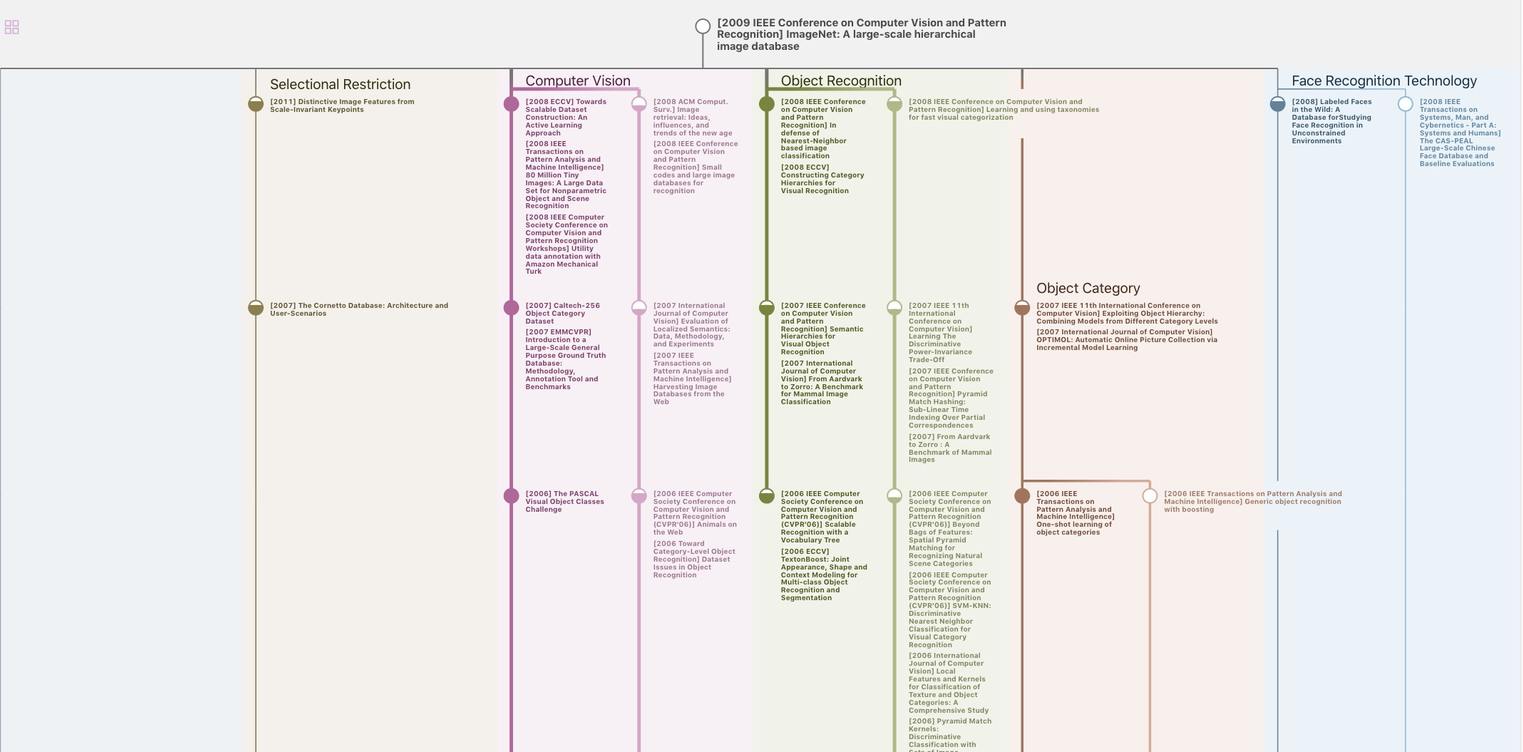
生成溯源树,研究论文发展脉络
Chat Paper
正在生成论文摘要