Early prediction of student knowledge in game-based learning with distributed representations of assessment questions
Br. J. Educ. Technol.(2023)
摘要
Game-based learning environments hold significant promise for facilitating learning experiences that are both effective and engaging. To support individualised learning and support proactive scaffolding when students are struggling, game-based learning environments should be able to accurately predict student knowledge at early points in students' gameplay. Student knowledge is traditionally assessed prior to and after each student interacts with the learning environment with conventional methods, such as multiple choice content knowledge assessments. While previous student modelling approaches have leveraged machine learning to automatically infer students' knowledge, there is limited work that incorporates the fine-grained content from each question in these types of tests into student models that predict student performance at early junctures in gameplay episodes. This work investigates a predictive student modelling approach that leverages the natural language text of the post-gameplay content knowledge questions and the text of the possible answer choices for early prediction of fine-grained individual student performance in game-based learning environments. With data from a study involving 66 undergraduate students from a large public university interacting with a game-based learning environment for microbiology, Crystal Island, we investigate the accuracy and early prediction capacity of student models that use a combination of gameplay features extracted from student log files as well as distributed representations of post-test content assessment questions. The results demonstrate that by incorporating knowledge about assessment questions, early prediction models are able to outperform competing baselines that only use student game trace data with no question-related information. Furthermore, this approach achieves high generalisation, including predicting the performance of students on unseen questions. Practitioner notesWhat is already known about this topic A distinctive characteristic of game-based learning environments is their capacity to enable fine-grained student assessment.Adaptive game-based learning environments offer individualisation based on specific student needs and should be able to assess student competencies using early prediction models of those competencies.Word embedding approaches from the field of natural language processing show great promise in the ability to encode semantic information that can be leveraged by predictive student models.What this paper adds Investigates word embeddings of assessment question content for reliable early prediction of student performance.Demonstrates the efficacy of distributed word embeddings of assessment questions when used by early prediction models compared to models that use either no assessment information or discrete representations of the questions.Demonstrates the efficacy and generalisability of word embeddings of assessment questions for predicting the performance of both new students on existing questions and existing students on new questions.Implications for practice and/or policy Word embeddings of assessment questions can enhance early prediction models of student knowledge, which can drive adaptive feedback to students who interact with game-based learning environments. Practitioners should determine if new assessment questions will be developed for their game-based learning environment, and if so, consider using our student modelling framework that incorporates early prediction models pretrained with existing student responses to previous assessment questions and is generalisable to the new assessment questions by leveraging distributed word embedding techniques.Researchers should consider the most appropriate way to encode the assessment questions in ways that early prediction models are able to infer relationships between the questions and gameplay behaviour to make accurate predictions of student competencies.
更多查看译文
关键词
game-based learning,natural language processing,predictive student modelling
AI 理解论文
溯源树
样例
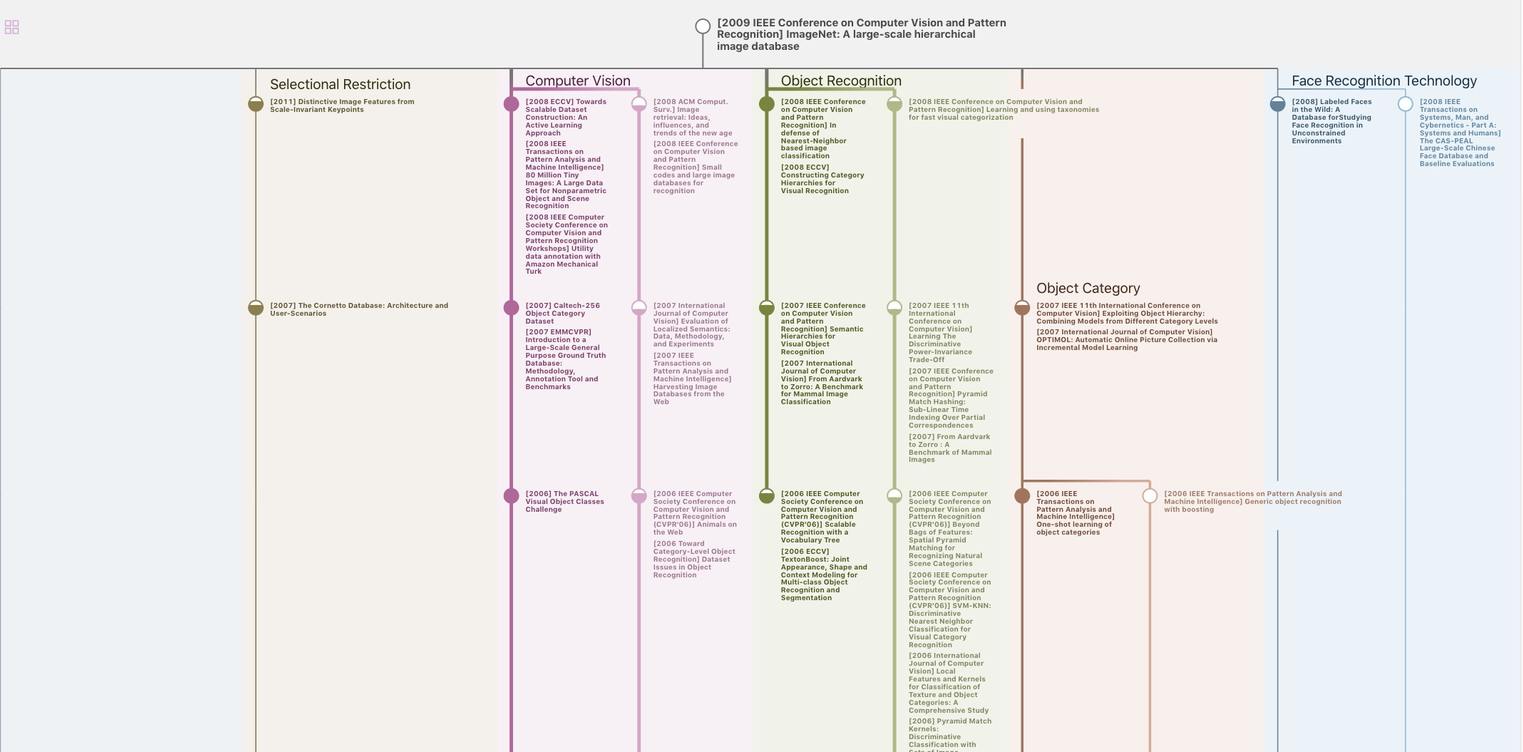
生成溯源树,研究论文发展脉络
Chat Paper
正在生成论文摘要