Unified Regression Model in Fitting Potential Energy Surfaces for Quantum Dynamics
Journal of Mathematical Chemistry(2022)
摘要
In this work, by discussing comparison of regression methods in fitting potential energy surfaces (PESs) for quantum dynamics, a unified regression model is proposed. Starting from the generalized linear regression (GLR), the popular neural-network (NN) approach together with the kernel model (KM) for regression can be derived. The NN approach has nested multi-layer structure of GLR, while methods of KM, such as Gaussian process regression and support vector regression, are also derived by GLR. Other derivative methods from either GLR or KM are also discussed by this formalism. Moreover, numerical comparisons of these methods are performed for fitting the PESs of the H + H _2 , H _2 + H _2 , and OH + HO _2 systems as well as the Hénon–Heiles model. The H + H _2 and H _2 + H _2 systems are constructed with the aid of previously reported BKMP2 (Boothroyd et al. in J Chem Phys 104:7139, 1996) and BMKP (Boothroyd et al. in J Chem Phys 116:666, 2002) PESs, respectively. The PESs of the OH + HO _2 system are constructed by the present ab initio energy calculations. The present numerical implementations clearly show that neither method is superior over the other and all have advantages and disadvantages. One should weight against each other for a specific case. Moreover, the present model might be helpful to inspire an idea for developing a new tool in fitting PES.
更多查看译文
AI 理解论文
溯源树
样例
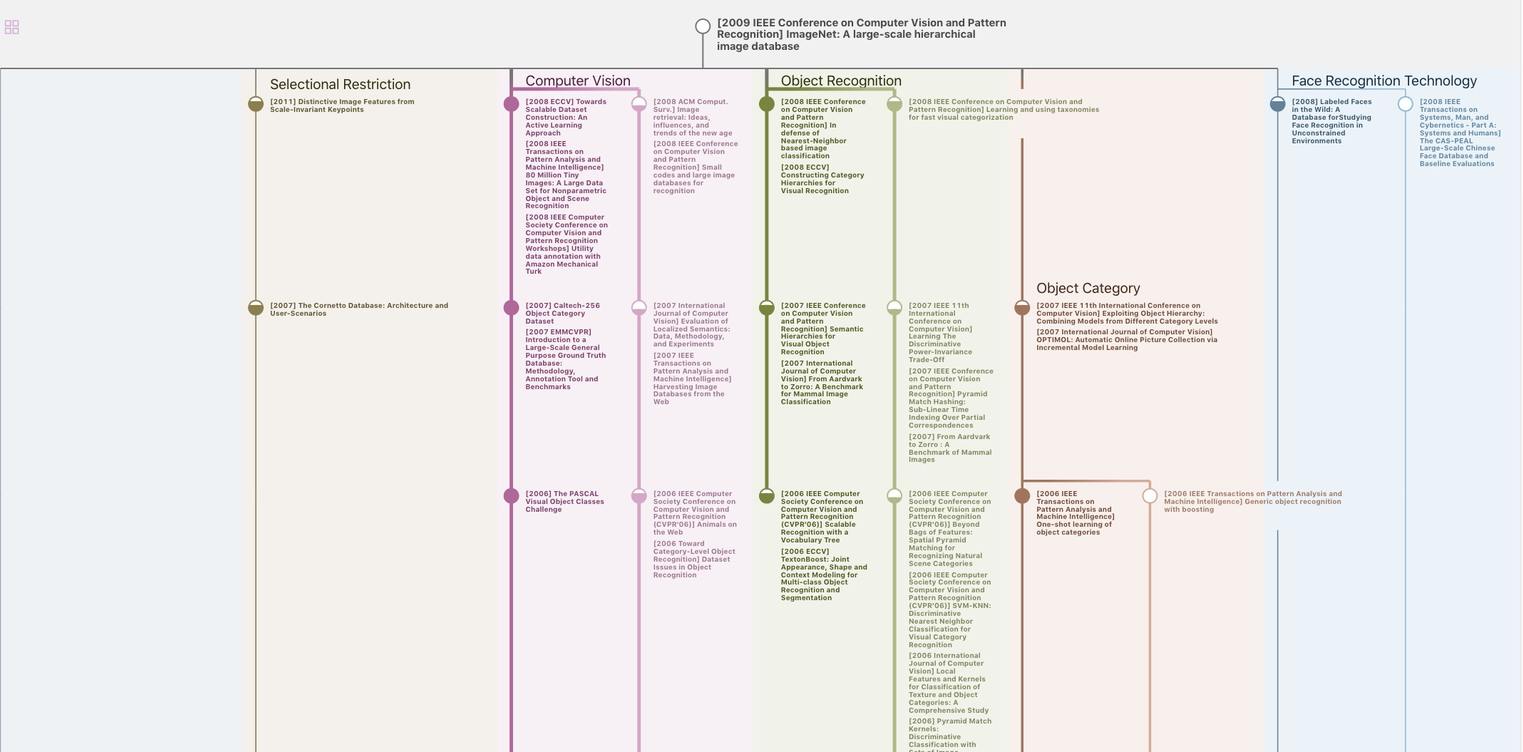
生成溯源树,研究论文发展脉络
Chat Paper
正在生成论文摘要