EEG Feature Significance Analysis.
International Conference on Neural Information Processing(2021)
摘要
Electroencephalography (EEG), a recording of brain activities, is usually feature fused. Although such feature fusion characteristic makes many brain-computer interfaces (BCI) possible, it makes it hard to distinguish task-specific features. As a result, current works usually use the whole EEG signal or features for a specific task like classification, regardless of the fact that many of the features are not task-related. In this paper, we aim to analyze the task-specific significance of EEG features. Specifically, we extract the frequency domain features and perform classification on them. To ensure a generalized conclusion, we use various classification architectures like Multilayer Perceptron (MLP) and 2D convolutional neural network (Conv2D). Extensive experiments are conducted on the UCI EEG dataset. We find that the front part of the brain, namely channel Fpz, AFz, Fp1, and Fp2 contains the general distinct features. Besides, the beta frequency band of the EEG signal is the most significant in the alcoholism classification task.
更多查看译文
关键词
significance,feature,analysis
AI 理解论文
溯源树
样例
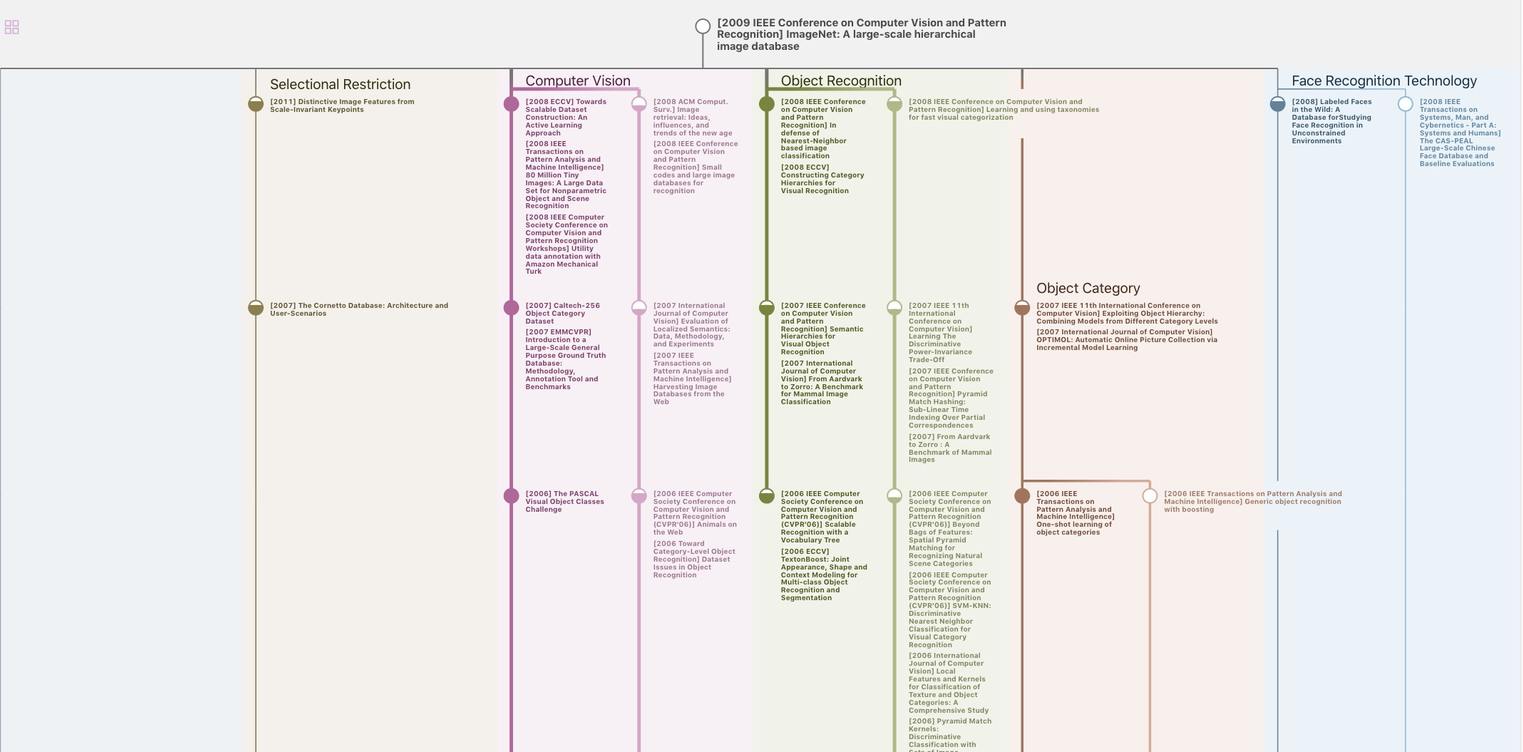
生成溯源树,研究论文发展脉络
Chat Paper
正在生成论文摘要