A Probabilistic Model Checking Approach to Self-adapting Machine Learning Systems
SOFTWARE ENGINEERING AND FORMAL METHODS: SEFM 2021 COLLOCATED WORKSHOPS(2022)
摘要
Machine Learning (ML) is increasingly used in domains such as cyber-physical systems and enterprise systems. These systems typically operate in non-static environments, prone to unpredictable changes that can adversely impact the accuracy of the ML models, which are usually in the critical path of the systems. Mispredictions of ML components can thus affect other components in the system, and ultimately impact overall system utility in non-trivial ways. From this perspective, self-adaptation techniques appear as a natural solution to reason about how to react to environment changes via adaptation tactics that can potentially improve the quality of ML models (e.g., model retrain), and ultimately maximize system utility. However, adapting ML components is non-trivial, since adaptation tactics have costs and it may not be clear in a given context whether the benefits of ML adaptation outweigh its costs. In this paper, we present a formal probabilistic framework, based on model checking, that incorporates the essential governing factors for reasoning at an architectural level about adapting ML classifiers in a system context. The proposed framework can be used in a selfadaptive system to create adaptation strategies that maximize rewards of a multi-dimensional utility space. Resorting to a running example from the enterprise systems domain, we show how the proposed framework can be employed to determine the gains achievable via ML adaptation and to find the boundary that renders adaptation worthwhile.
更多查看译文
关键词
Machine-learning based systems, Self-adaptation, Probabilistic model checking, Architectural framework
AI 理解论文
溯源树
样例
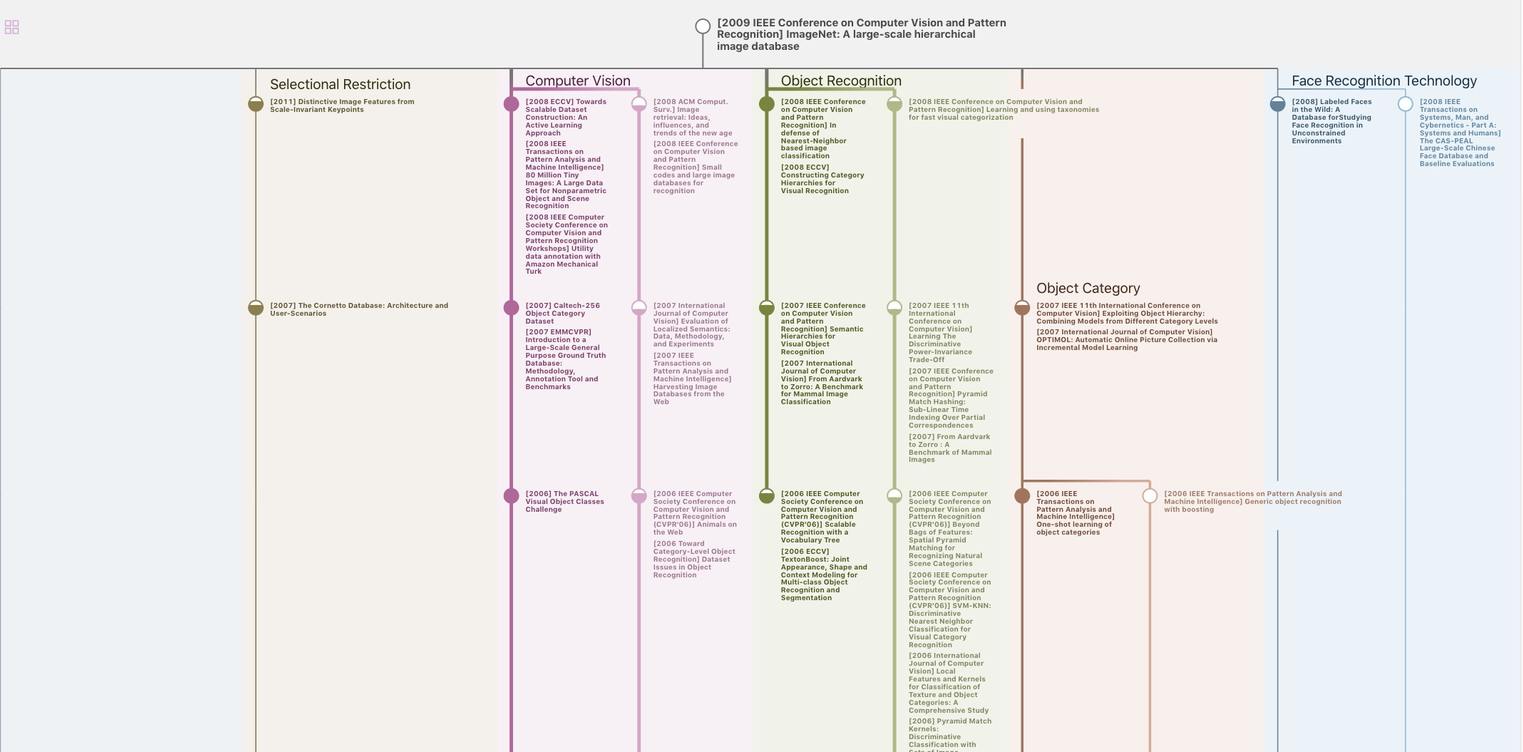
生成溯源树,研究论文发展脉络
Chat Paper
正在生成论文摘要