Prototype augmented network with metric-mixed under limited samples for mechanical intelligent fault recognition.
Appl. Soft Comput.(2022)
摘要
Common deep learning methods can effectively extract fault characteristics and make recognition of mechanical faults. Nevertheless, the results are undesirable when sufficient labeled training data are unavailable. Although metric learning methods can conduct fault recognition with limited samples, they cannot get satisfactory results due to the fixed relation metric function of manually selected. To address this problem we proposed a prototype augmented network with metric-mixed under limited samples (PANM). It combines fixed metric function with the network automatically learning metric, which consists of an embedded module, relation module and metric module, respectively Firstly, the class prototypes are augmented via multilayer residual network in the embedded module. Then, in the relation module, the prototypes are concatenated with training features to automatically learn the corresponding relationship for getting relation scores. Next, the metric module is used to measure the fixed spatial distance and normalize as the weights of each score. Finally, fault identification is taken according to the weighted score. The experiments in two datasets indicate that PANM cannot just identify different fault categories; it may identify new untrained classes without modifying the network parameters as well, which demonstrates practical solving ability and good generalization ability of fault recognition under limited samples.(c) 2022 Elsevier B.V. All rights reserved.
更多查看译文
关键词
Fault recognition,Limited samples,Hybrid metric,Prototype augmentation
AI 理解论文
溯源树
样例
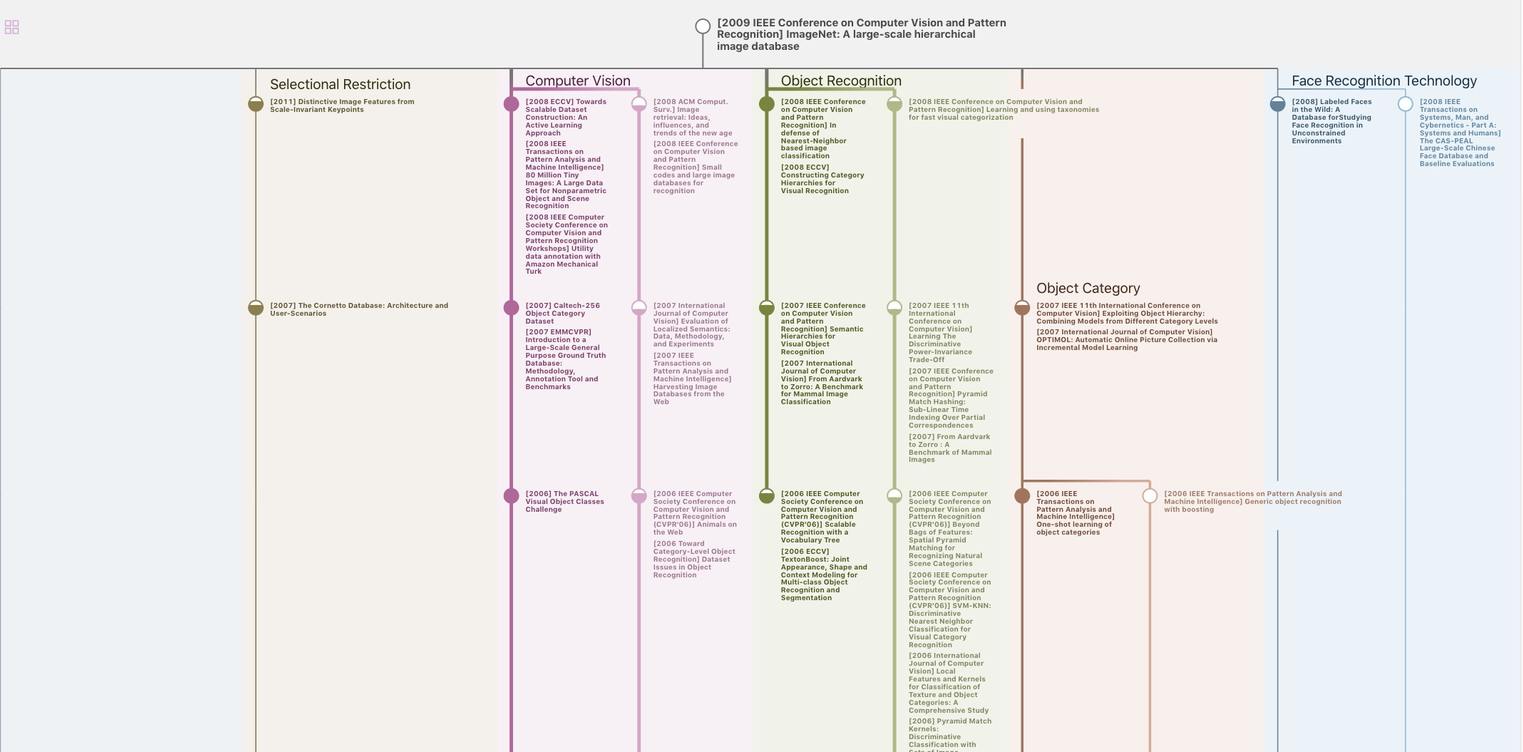
生成溯源树,研究论文发展脉络
Chat Paper
正在生成论文摘要