Unsupervised Change Detection in Very High Resolution Multi-Spectral Images
2021 IEEE International India Geoscience and Remote Sensing Symposium (InGARSS)(2021)
摘要
Change detection for remote sensing images is a critical task especially for the applications including monitoring the environment, urban planning, investigating agriculture, disaster management, etc. Although most of the exiting deep models for change detection are supervised in nature, unsupervised learning-based models are also gaining popularity because of the scarcity of the annotated training examples. In this paper, we have pro-posed an unsupervised change detection algorithm for VHR (Very High Resolution) multispectral images. It uses a deep CNN model [6] to generate the deep change vector which is then subjected to Slow Feature Analysis (SFA) module [2] to suppress the unchanged components and highlight the transformed features’ changed components. An appropriate thresholding technique is then applied to generate the required binary changed map. Experimental observation reveals that the proposed unsupervised model shows competitive performance with the state-of-the-art methods. Our proposed approach has achieved fifth place
1
in Dynamic Earth Net Challenge Track 1 of EARTHVISION 2021.
更多查看译文
关键词
remote sensing images,very high resolution multispectral images,urban planning,disaster management,unsupervised learning,annotated training examples,unsupervised change detection,VHR multispectral images,deep CNN model,deep change vector,feature analysis
AI 理解论文
溯源树
样例
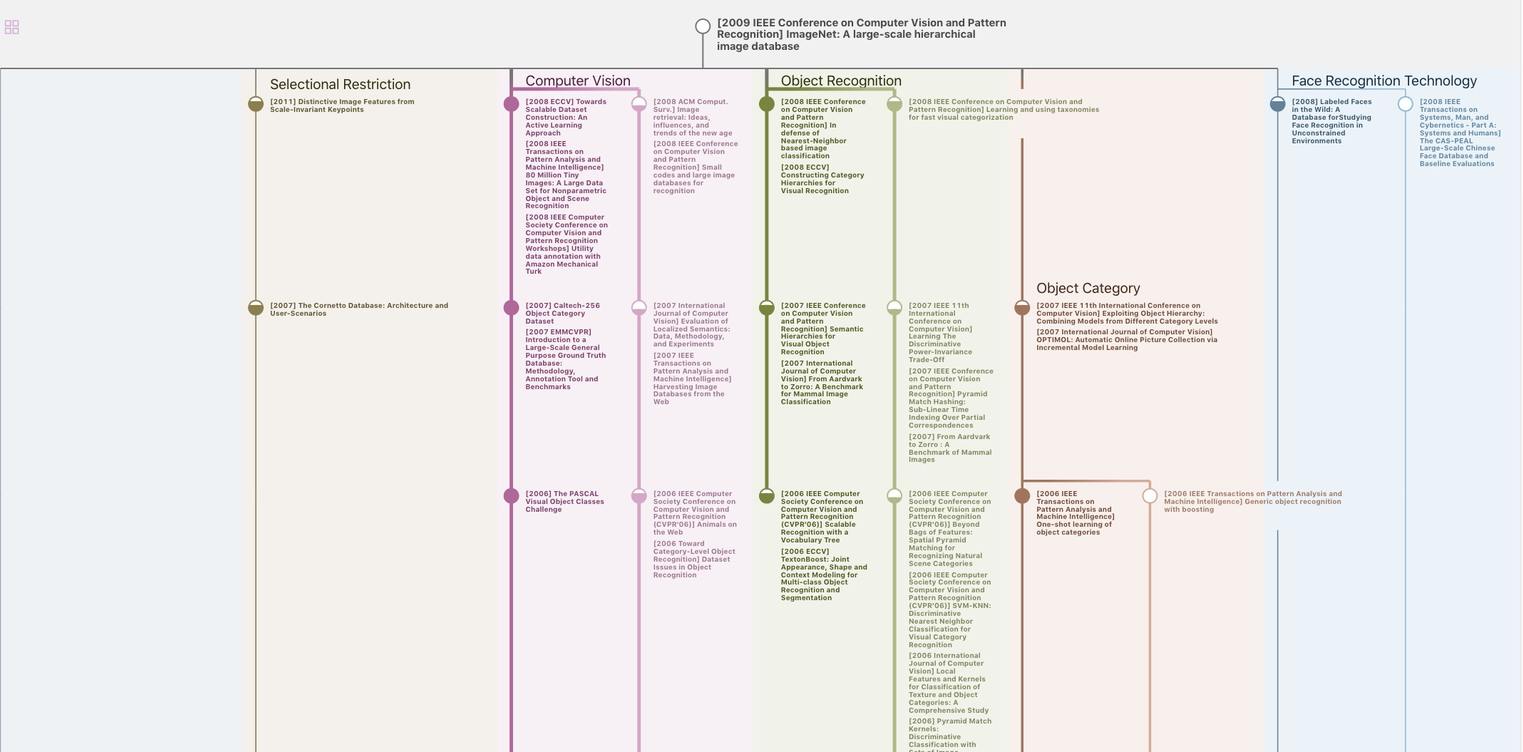
生成溯源树,研究论文发展脉络
Chat Paper
正在生成论文摘要