SparseTFNet: A Physically Informed Autoencoder for Sparse Time-Frequency Analysis of Seismic Data
IEEE TRANSACTIONS ON GEOSCIENCE AND REMOTE SENSING(2022)
摘要
The time-frequency (TF) analysis is an effective tool in seismic signal processing. The sparsity-based TF transforms have been widely used to obtain high localized TF representations in recent past years. These TF transforms formulate a sparse TF representation as an inverse optimization problem using simple mathematical models, which are typically based on a handcrafted prior knowledge. Unlike the traditional sparsity-based TF transforms, the supervised deep learning (DL)-based sparse TF representations do not require this prior knowledge and instead use a large amount of labeled dataset, which is difficult to label for seismic data. In this study, to bridge the gap between the traditional sparsity-based transforms and the supervised DL-based transforms, we propose a DL-based sparse TF analysis approach based on a physically informed autoencoder model, named the SparseTFNet. The proposed SparseTFNet includes two modules: a convolutional neural networks (CNN)-based encoder and a traditional inverse TF representation-based decoder. The CNN-based encoder is implemented by training the inverse optimization problem in the absence of the "ground-truth" TF representation, which can be trained with only seismic traces. The traditional inverse short-time Fourier transform (STFT) is utilized as the decoder module in this study, which is used as a physical constraint to ensure the high accuracy of the calculated TF representation. Finally, after training and validating the proposed model using the noise-free and noisy synthetic seismic traces, the model is applied to 3-D offshore seismic data. The results show that the proposed SparseTFNet model has good performance in the delineation of the depositional fluvial channels.
更多查看译文
关键词
Transforms,Data models,Mathematical models,Time-frequency analysis,Decoding,Analytical models,Three-dimensional displays,Autoencoder,compressed sensing (CS),deep learning (DL),sparse time-frequency (TF) representation
AI 理解论文
溯源树
样例
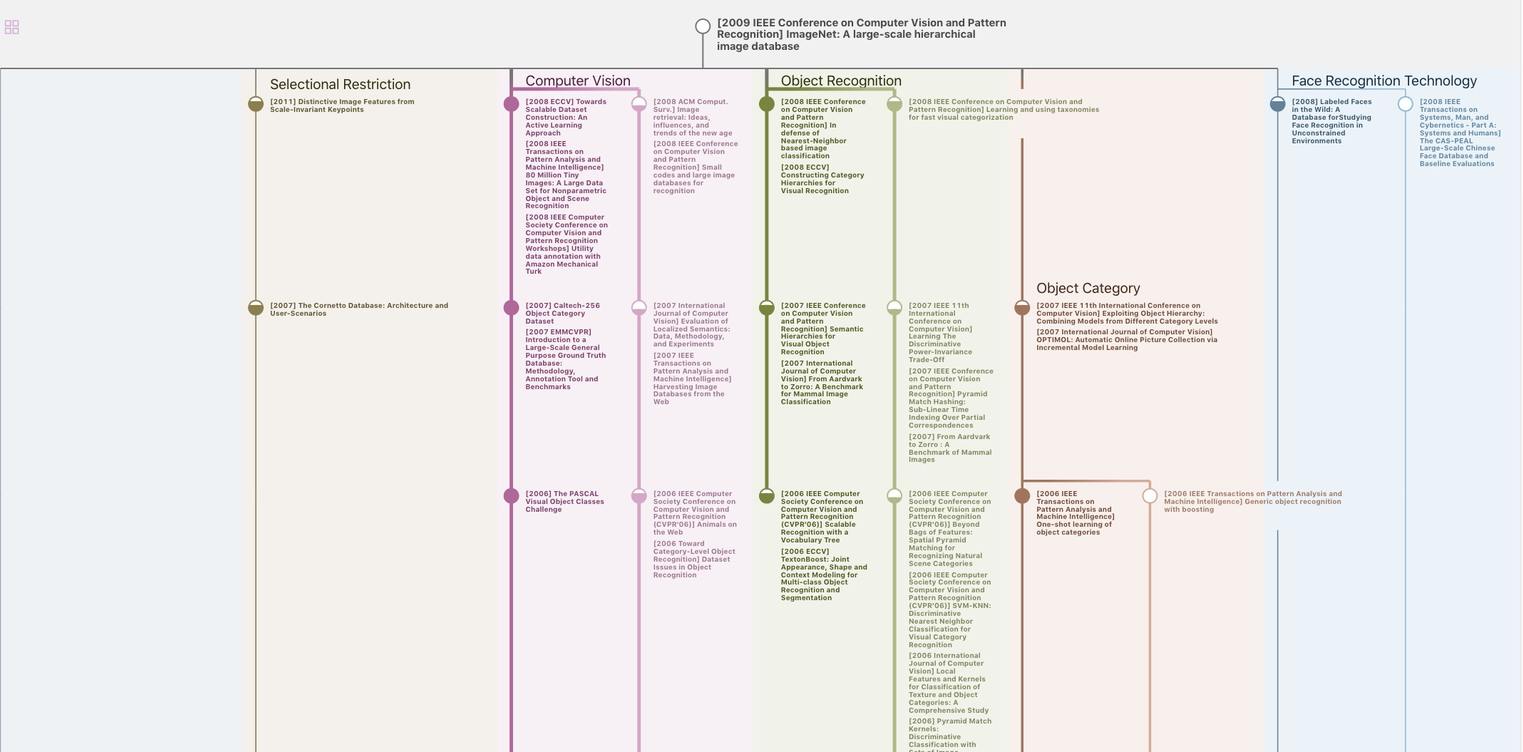
生成溯源树,研究论文发展脉络
Chat Paper
正在生成论文摘要