P3S: A High Accuracy Probabilistic Prediction Processing System for CNN Acceleration
Proceedings of the Great Lakes Symposium on VLSI 2022(2022)
摘要
Convolutional Neural Networks (CNNs) achieve state-of-the-art performance for perception tasks at the cost of billions of computational operations. In this paper, we propose a probabilistic prediction processing system, dubbed P3S, to eliminate redundant compute-heavy convolution operations by predicting whether output activations are zero-valued. By exploiting the probability characteristic of Gaussian-like distributed activations and weights in CNNs, P3S calculates the partial convolution across values greater than a standard deviation-related threshold, to predict the ineffectual output activations. P3S skips remaining convolutions and sets outputs to zero in advance if output activations are predicted to be zero. P3S reduces 67% computations within 0.2% accuracy loss and does not even require retraining or fine-tuning CNNs. We further implement a P3S-based CNN accelerator that achieves 2.02x speedup and 2.23x energy efficiency on average over the traditional accelerator. Compared with the state-of-the-art prediction-based accelerator with 3% accuracy degradation, our P$^3$S yields up to 1.49x speedup and 1.69x energy efficiency.
更多查看译文
关键词
Representation Learning,Transfer Learning,Semi-Supervised Learning,Clustering Analysis
AI 理解论文
溯源树
样例
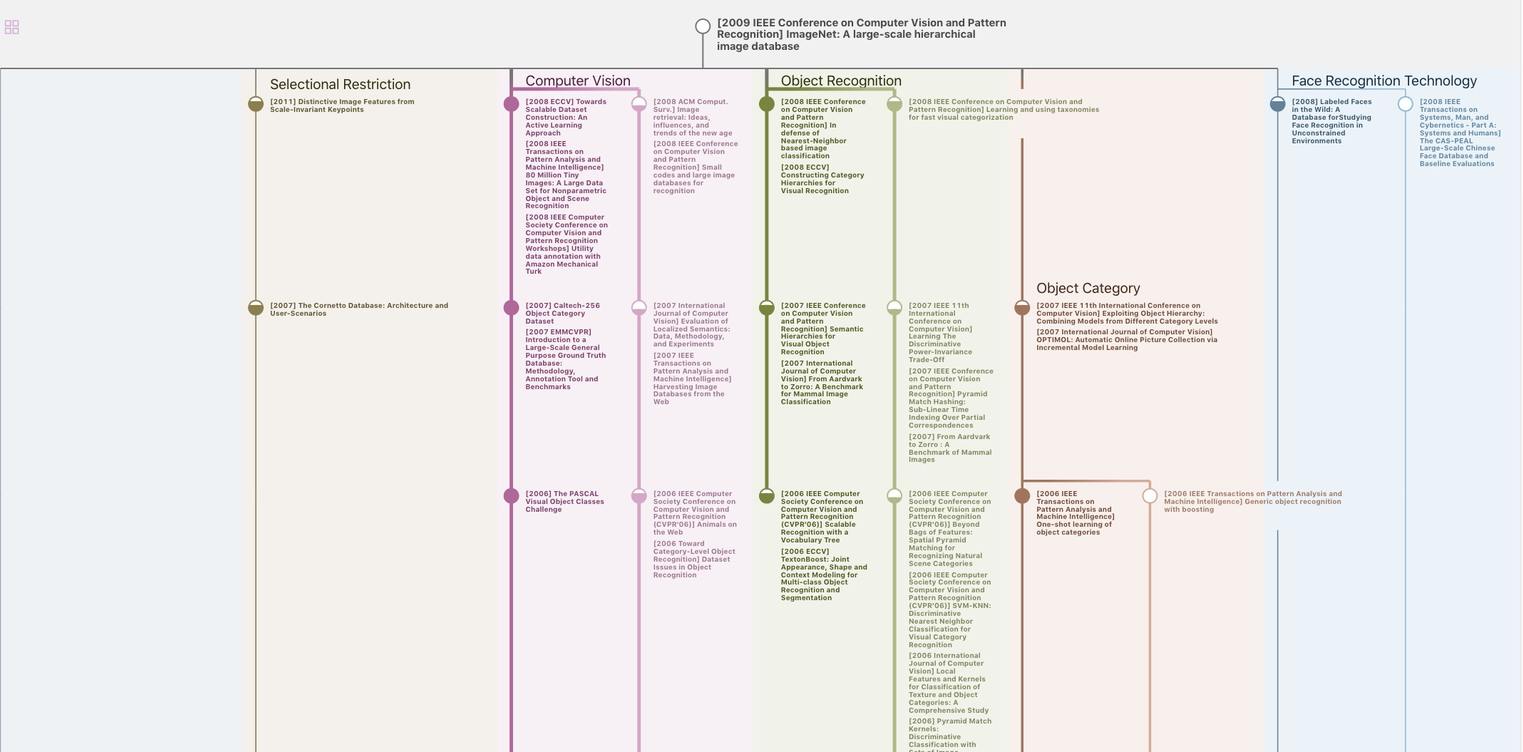
生成溯源树,研究论文发展脉络
Chat Paper
正在生成论文摘要