A novel image-based approach for soybean seed phenotyping using machine learning techniques
biorxiv(2022)
摘要
Soybean is one of the most important sources of protein and vegetable oil in the world. Given its increasing demand, the increment in yield has only been possible due to investments in research and production technology, especially in breeding programs. One of the main factors influencing soybean yield is the seed morphology; however, its analyses are hampered by the lack of efficient computational approaches with not only accurate results, but also a high flexibility to user preferences and needs. In this context, the present work provides a methodological framework for: (i) seed segmentation in soybean images; (ii) seed morphological evaluation; and (iii) image-based prediction of the hundred-seed weight trait. We used genotypes from a partial diallel cross design, which aimed at obtaining genotypes with high agronomic performance. In addition to the measurement of the hundred-seed weight, we collected RGB images of seeds of each plot. For image segmentation, we created an in-house image processing pipeline, which enabled a full morphological seed evaluation. For predicting the hundred-seed weight, we compared different machine learning algorithms using as input the morphological characteristics obtained, and also features from state-of-the-art convolutional neural network (CNN) architectures. The image segmentation methodology showed to be highly efficient, as more than 98% of the seeds in the images were correctly identified. Even if the seeds were close, the segmentation strategy could separate them into independent image components. In addition to supplying a highly accurate decision support system for soybean breeders, we verified the morphological phenotyping adaptability in other plant species, fully assessing the pipeline generalization. We consider the use of this methodology highly advantageous, as the method is entirely based on widely used morphological operations, which results in an easy implementation and low computational costs. Using these morphological measures, we could estimate machine learning models for predicting the hundred-seed weight, achieving considerable predictive accuracy. The same results were observed for CNN-obtained features, showing the efficiency of the morphological measurements as feature extractors. The possibility of obtaining seed morphological characteristics provides a valuable tool for the continuous and efficient development of new soybean cultivars in breeding programs aimed at long-term genetic gain. Additionally, through a faster seed image acquisition workflow, with less chance of errors and low cost, it is also possible to make predictions of important soybean characteristics. The work conducted has the potential to help future research and the industry to develop automated phenotyping tools, incorporating the proposed analytical workflows.
### Competing Interest Statement
The authors have declared no competing interest.
更多查看译文
AI 理解论文
溯源树
样例
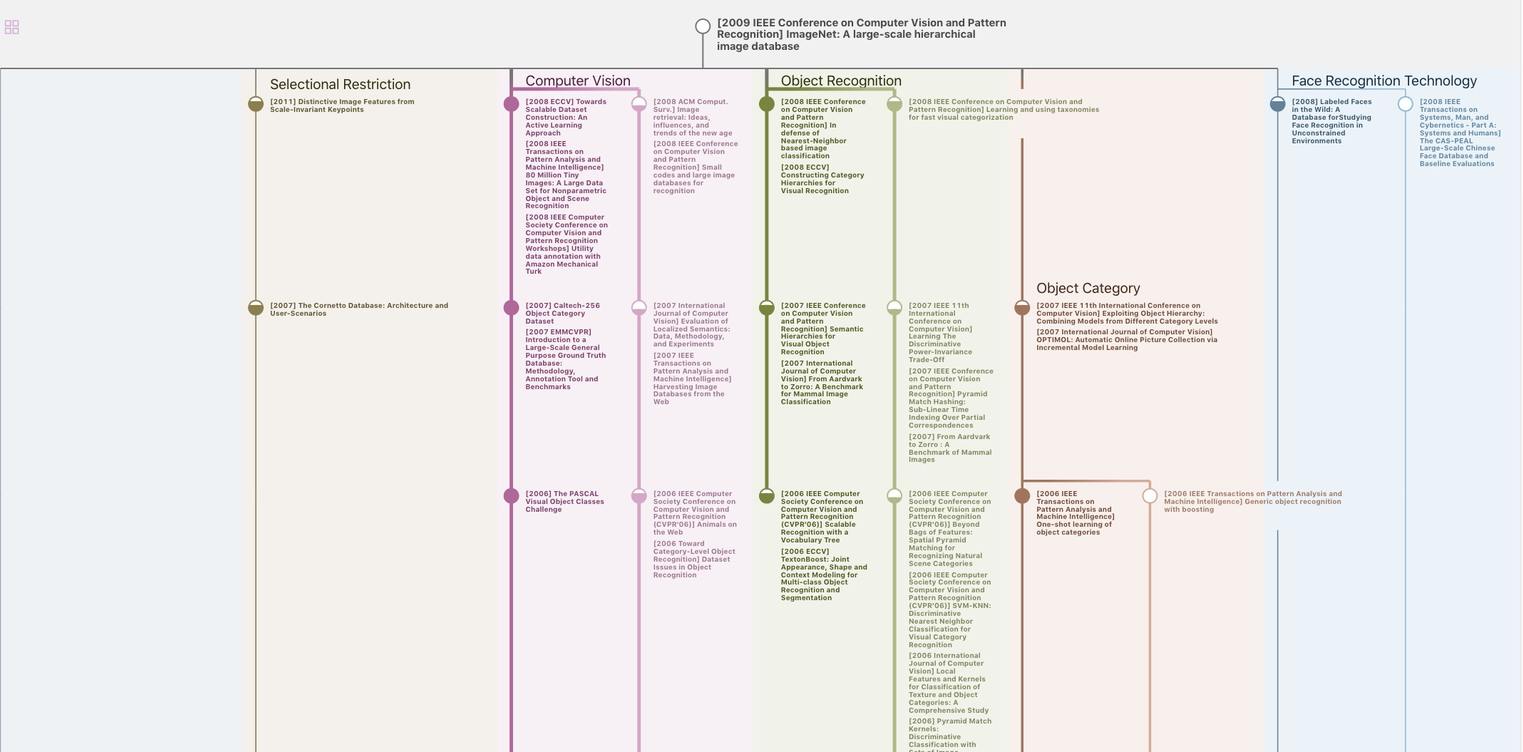
生成溯源树,研究论文发展脉络
Chat Paper
正在生成论文摘要