Self-supervised video pretraining yields strong image representations
ICLR 2023(2022)
摘要
Videos contain far more information than still images and hold the potential for learning rich representations of the visual world. Yet, pretraining on image datasets has remained the dominant paradigm for learning representations that capture spatial information, and previous attempts at video pretraining have fallen short on image understanding tasks. In this work we revisit self-supervised learning of image representations from the dynamic evolution of video frames. To that end, we propose a dataset curation procedure that addresses the domain mismatch between video and image datasets, and develop a contrastive learning framework which handles the complex transformations present in natural videos. This simple paradigm for distilling knowledge from videos to image representations, called VITO, performs surprisingly well on a variety of image-based transfer learning tasks. For the first time, our video-pretrained model closes the gap with ImageNet pretraining on semantic segmentation on PASCAL and ADE20K and object detection on COCO and LVIS, suggesting that video-pretraining could become the new default for learning image representations.
更多查看译文
关键词
self-supervised,contrastive,video,representation learning,image segmentation,object detection
AI 理解论文
溯源树
样例
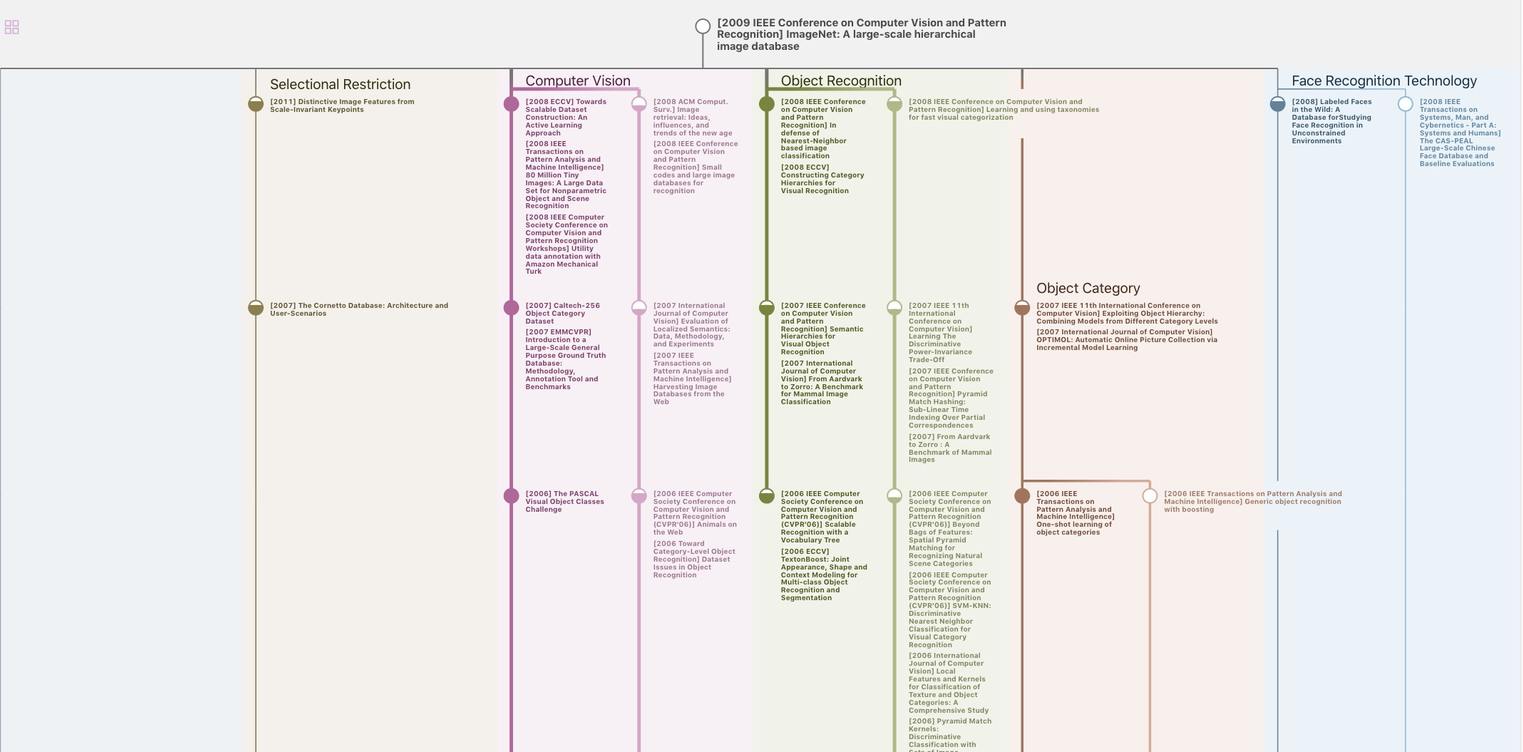
生成溯源树,研究论文发展脉络
Chat Paper
正在生成论文摘要