Probabilistic Inverse Modeling: An Application in Hydrology
arxiv(2022)
摘要
The astounding success of these methods has made it imperative to obtain more explainable and trustworthy estimates from these models. In hydrology, basin characteristics can be noisy or missing, impacting streamflow prediction. For solving inverse problems in such applications, ensuring explainability is pivotal for tackling issues relating to data bias and large search space. We propose a probabilistic inverse model framework that can reconstruct robust hydrology basin characteristics from dynamic input weather driver and streamflow response data. We address two aspects of building more explainable inverse models, uncertainty estimation and robustness. This can help improve the trust of water managers, handling of noisy data and reduce costs. We propose uncertainty based learning method that offers 6\% improvement in $R^2$ for streamflow prediction (forward modeling) from inverse model inferred basin characteristic estimates, 17\% reduction in uncertainty (40\% in presence of noise) and 4\% higher coverage rate for basin characteristics.
更多查看译文
关键词
probabilistic inverse modeling,hydrology
AI 理解论文
溯源树
样例
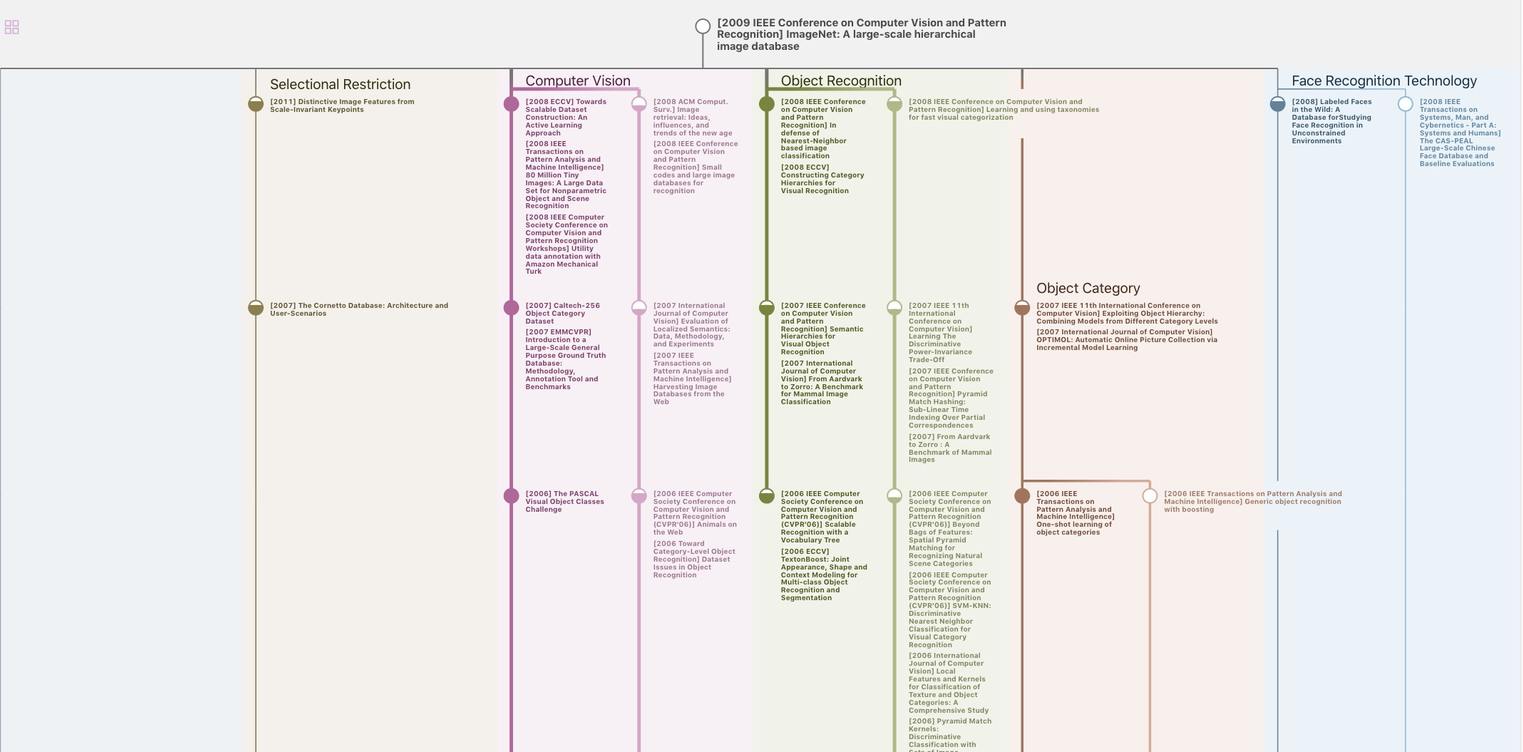
生成溯源树,研究论文发展脉络
Chat Paper
正在生成论文摘要