Uplift and Upsample: Efficient 3D Human Pose Estimation with Uplifting Transformers
WACV(2023)
摘要
The state-of-the-art for monocular 3D human pose estimation in videos is dominated by the paradigm of 2D-to-3D pose uplifting. While the uplifting methods themselves are rather efficient, the true computational complexity depends on the per-frame 2D pose estimation. In this paper, we present a Transformer-based pose uplifting scheme that can operate on temporally sparse 2D pose sequences but still produce temporally dense 3D pose estimates. We show how masked token modeling can be utilized for temporal upsampling within Transformer blocks. This allows to decouple the sampling rate of input 2D poses and the target frame rate of the video and drastically decreases the total computational complexity. Additionally, we explore the option of pre-training on large motion capture archives, which has been largely neglected so far. We evaluate our method on two popular benchmark datasets: Human3.6M and MPI-INF-3DHP. With an MPJPE of 45:0 mm and 46:9 mm, respectively, our proposed method can compete with the state-of-the-art while reducing inference time by a factor of 12. This enables real-time throughput with variable consumer hardware in stationary and mobile applications. We release our code and models at https://github.com/ goldbricklemon/uplift-upsample-3dhpe
更多查看译文
关键词
Algorithms: 3D computer vision,Biometrics,face,gesture,body pose,Video recognition and understanding (tracking,action recognition,etc.)
AI 理解论文
溯源树
样例
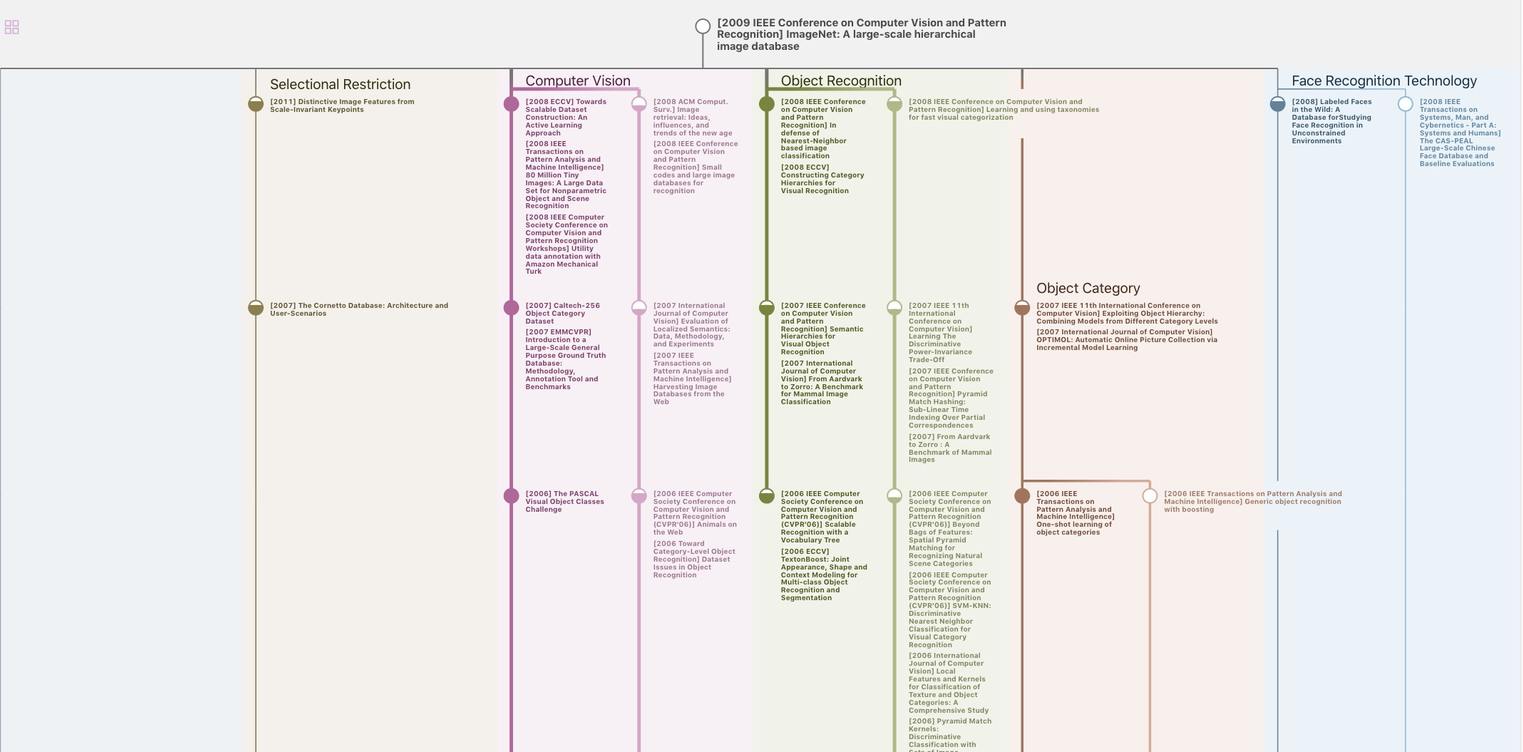
生成溯源树,研究论文发展脉络
Chat Paper
正在生成论文摘要