A sparse matrix formulation of model-based ensemble Kalman filter
arxiv(2023)
摘要
We introduce a computationally efficient variant of the model-based ensemble Kalman filter (EnKF). We propose two changes to the original formulation. First, we phrase the setup in terms of precision matrices instead of covariance matrices, and introduce a new prior for the precision matrix which ensures it to be sparse. Second, we propose to split the state vector into several blocks and formulate an approximate updating procedure for each of these blocks. We study in a simulation example the computational speedup and the approximation error resulting from using the proposed approach. The speedup is substantial for high dimensional state vectors, allowing the proposed filter to be run on much larger problems than can be done with the original formulation. In the simulation example the approximation error resulting from using the introduced block updating is negligible compared to the Monte Carlo variability inherent in both the original and the proposed procedures.
更多查看译文
关键词
Bayesian inference,Data assimilation,Ensemble Kalman filter,Gaussian Markov random field,Hidden Markov model,Spatial statistics
AI 理解论文
溯源树
样例
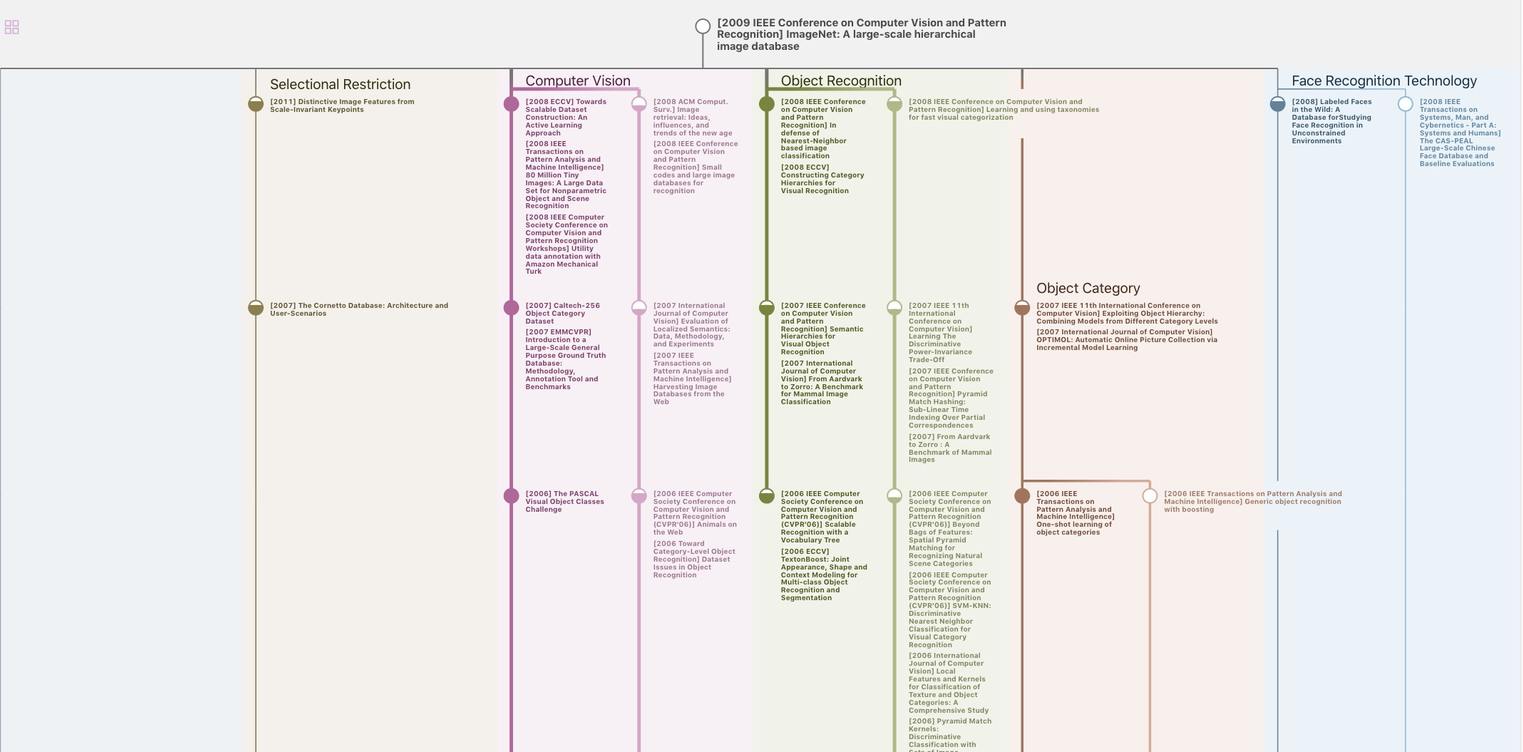
生成溯源树,研究论文发展脉络
Chat Paper
正在生成论文摘要