The computational and learning benefits of Daleian neural networks
NeurIPS 2022(2022)
摘要
Dale's principle implies that biological neural networks are composed of neurons that are either excitatory or inhibitory. While the number of possible architectures of such Daleian networks is exponentially smaller than non-Daleian ones, the computational and functional implications of using Daleian networks by the brain are mostly unknown. Here, we use models of recurrent spiking neural networks and rate-based networks to show, surprisingly, that despite the structural limitations on Daleian networks, they can approximate the computation performed by non-Daleian networks to a very high degree of accuracy. Moreover, we find that Daleian networks are more functionally robust to synaptic noise. We then show that unlike non-Daleian networks, Daleian ones can learn efficiently by tuning single neuron features, nearly as well as learning by tuning individual synaptic weights - suggesting a simpler and more biologically plausible learning mechanism. We thus suggest that in addition to architectural simplicity, Dale's principle confers computational and learning benefits for biological networks, and offers new directions for constructing and training biologically-inspired artificial neural networks
更多查看译文
关键词
neural coding,dale’s principle,spiking neural networks,recurrent neural networks,neural architecture,learning,information coding
AI 理解论文
溯源树
样例
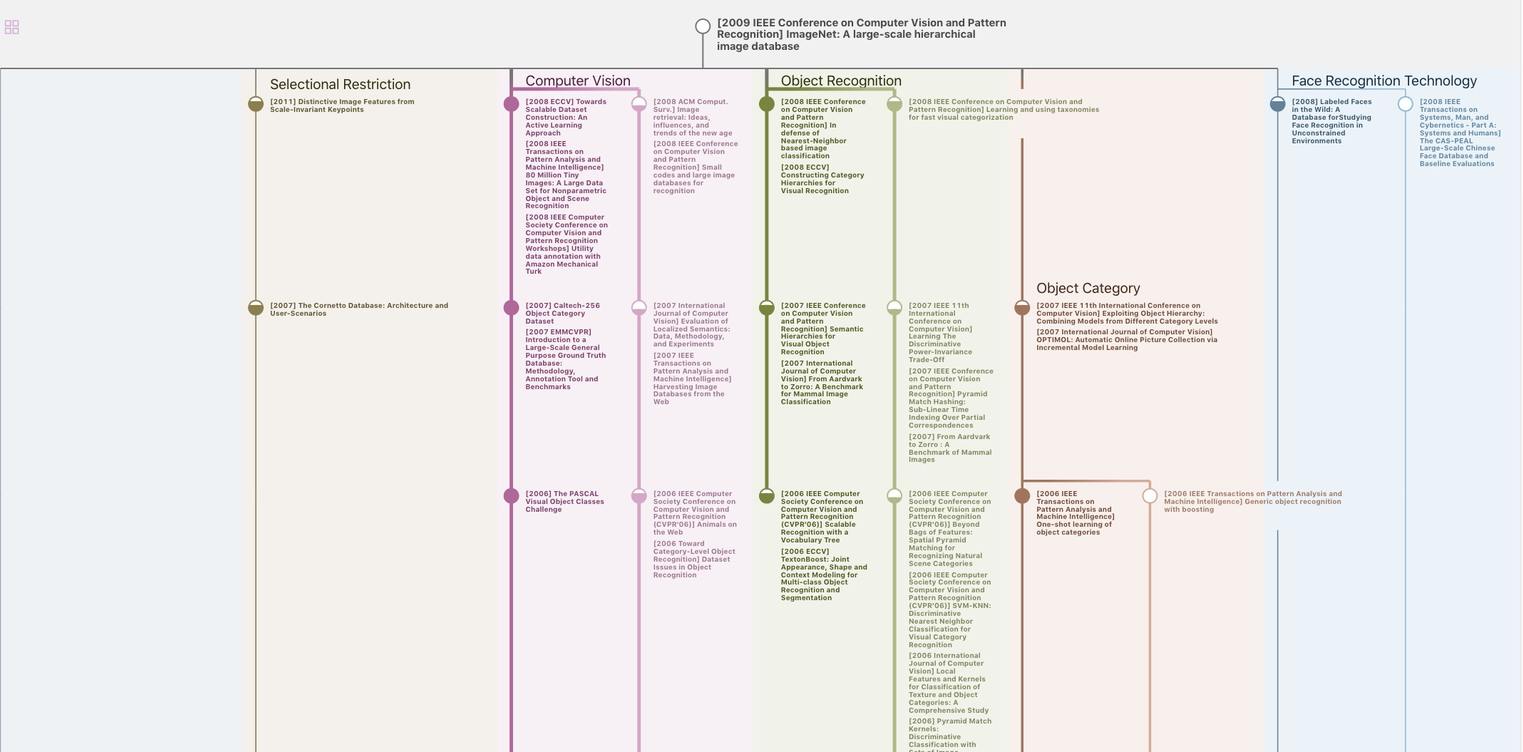
生成溯源树,研究论文发展脉络
Chat Paper
正在生成论文摘要