On RKHS Choices for Assessing Graph Generators via Kernel Stein Statistics
arxiv(2022)
摘要
Score-based kernelised Stein discrepancy (KSD) tests have emerged as a powerful tool for the goodness of fit tests, especially in high dimensions; however, the test performance may depend on the choice of kernels in an underlying reproducing kernel Hilbert space (RKHS). Here we assess the effect of RKHS choice for KSD tests of random networks models, developed for exponential random graph models (ERGMs) in Xu and Reinert (2021)and for synthetic graph generators in Xu and Reinert (2022). We investigate the power performance and the computational runtime of the test in different scenarios, including both dense and sparse graph regimes. Experimental results on kernel performance for model assessment tasks are shown and discussed on synthetic and real-world network applications.
更多查看译文
关键词
graph generators,kernel stein
AI 理解论文
溯源树
样例
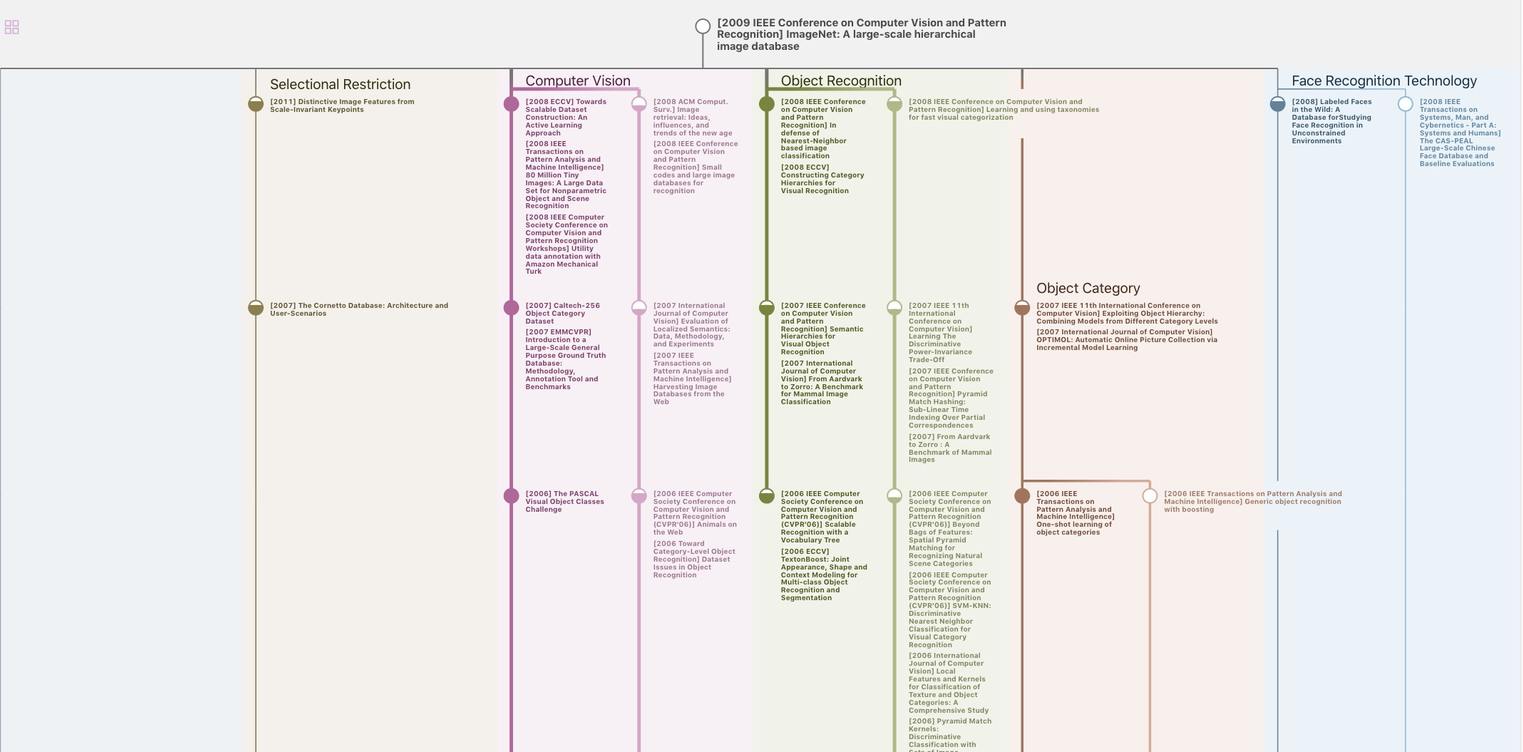
生成溯源树,研究论文发展脉络
Chat Paper
正在生成论文摘要