Towards Consistency and Complementarity: A Multiview Graph Information Bottleneck Approach
arxiv(2022)
摘要
The empirical studies of Graph Neural Networks (GNNs) broadly take the original node feature and adjacency relationship as singleview input, ignoring the rich information of multiple graph views. To circumvent this issue, the multiview graph analysis framework has been developed to fuse graph information across views. How to model and integrate shared (i.e. consistency) and view-specific (i.e. complementarity) information is a key issue in multiview graph analysis. In this paper, we propose a novel Multiview Variational Graph Information Bottleneck (MVGIB) principle to maximize the agreement for common representations and the disagreement for view-specific representations. Under this principle, we formulate the common and view-specific information bottleneck objectives across multiviews by using constraints from mutual information. However, these objectives are hard to directly optimize since the mutual information is computationally intractable. To tackle this challenge, we derive variational lower and upper bounds of mutual information terms, and then instead optimize variational bounds to find the approximate solutions for the information objectives. Extensive experiments on graph benchmark datasets demonstrate the superior effectiveness of the proposed method.
更多查看译文
关键词
multiview graph information
AI 理解论文
溯源树
样例
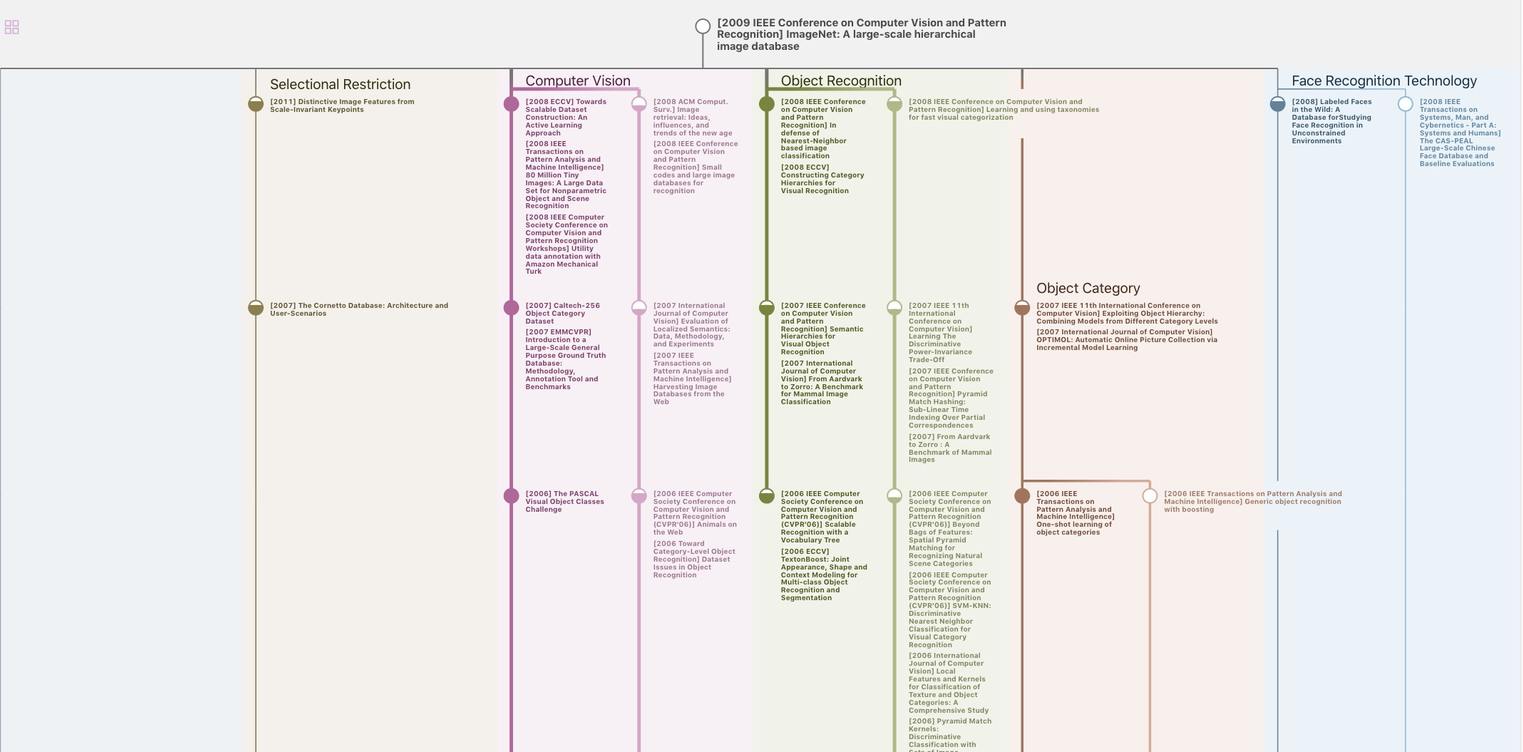
生成溯源树,研究论文发展脉络
Chat Paper
正在生成论文摘要