Localizing seizure onset zones in surgical epilepsy with neurostimulation deep learning
Journal of Neurosurgery(2022)
摘要
OBJECTIVE In drug-resistant temporal lobe epilepsy, automated tools for seizure onset zone (SOZ) localization that use brief interictal recordings could supplement presurgical evaluations and improve care. Thus, the authors sought to localize SOZs by training a multichannel convolutional neural network on stereoelectroencephalography (SEEG) cortico-cortical evoked potentials.METHODS The authors performed single-pulse electrical stimulation in 10 drug-resistant temporal lobe epilepsy patients implanted with SEEG. Using 500,000 unique poststimulation SEEG epochs, the authors trained a multichannel 1-dimensional convolutional neural network to determine whether an SOZ had been stimulated. RESULTS SOZs were classified with mean sensitivity of 78.1% and specificity of 74.6% according to leave-one-patient -out testing. To achieve maximum accuracy, the model required a 0-to 350-msec poststimulation time period. Post hoc analysis revealed that the model accurately classified unilateral versus bilateral mesial temporal lobe seizure onset, as well as neocortical SOZs. CONCLUSIONS This was the first demonstration, to the authors' knowledge, that a deep learning framework can be used to accurately classify SOZs with single-pulse electrical stimulation-evoked responses. These findings suggest that ac-curate classification of SOZs relies on a complex temporal evolution of evoked responses within 350 msec of stimulation. Validation in a larger data set could provide a practical clinical tool for the presurgical evaluation of drug-resistant epilepsy. https://thejns.org/doi/abs/10.3171/2022.8.JNS221321
更多查看译文
关键词
seizure onset zones,surgical epilepsy,neurostimulation,deep learning
AI 理解论文
溯源树
样例
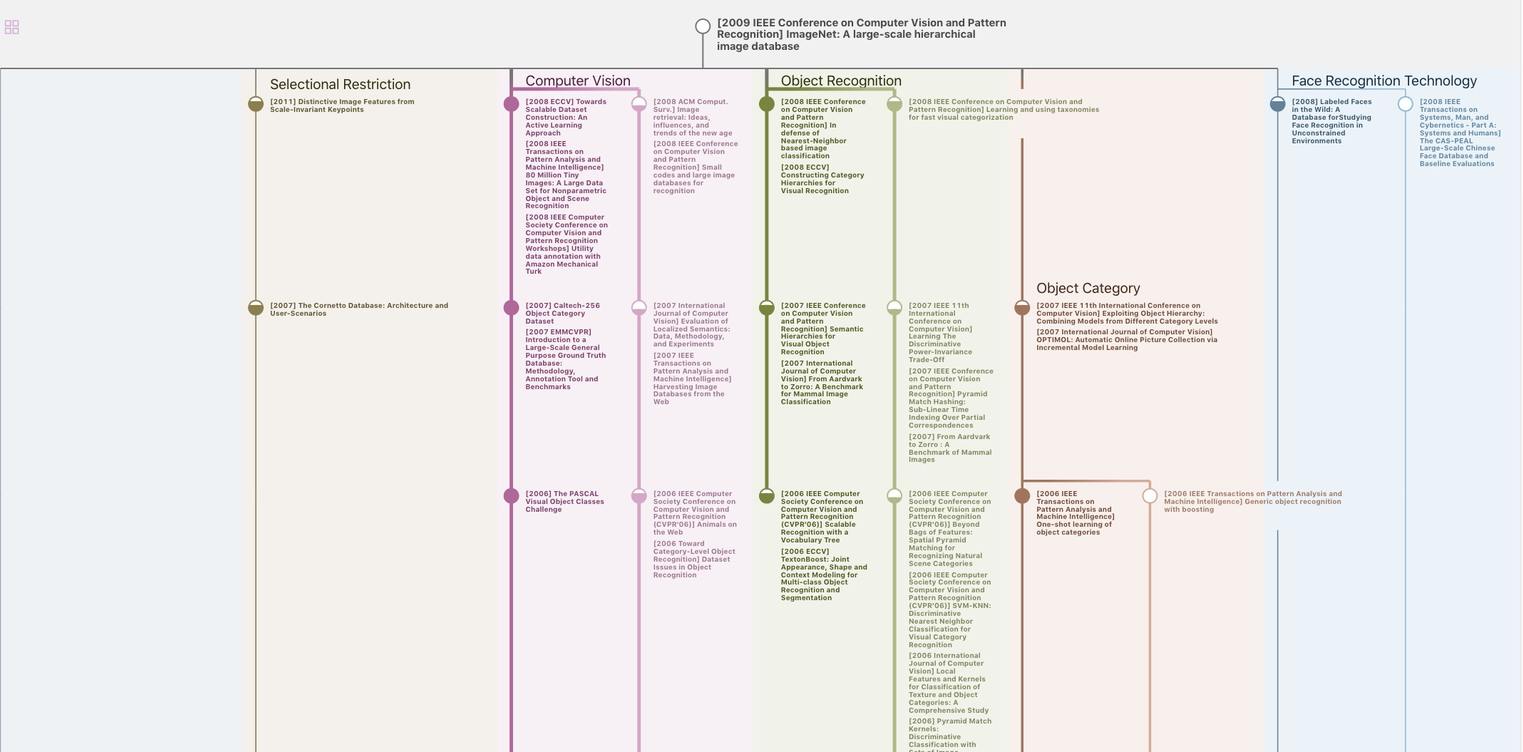
生成溯源树,研究论文发展脉络
Chat Paper
正在生成论文摘要