Seeking patterns in rms voltage variations at the sub-10-minute scale from multiple locations via unsupervised learning and patterns? post-processing
INTERNATIONAL JOURNAL OF ELECTRICAL POWER & ENERGY SYSTEMS(2022)
摘要
This paper addresses the issue of seeking sub-10-min patterns in fast rms voltage variations from time-limited measurement data at multiple locations worldwide. This is a rarely considered time scale in studies that could be important for the incorrect operation of end-user equipment. Moreover, measurements from multiple loca-tions could be significant from the view of seeking pattern methods. To learn more about this time scale, we propose an unsupervised learning method that employs a Kernel Principal Component Analysis (KPCA) with a Cosine kernel to extract principal features from 10-min time series of voltage variations with a 1-s resolution followed by a k-means clustering to group the features. The scheme is applied to measurements from 57 low-voltage locations in 19 countries from 2009 to 2018. Fifteen initial clusters/patterns are then extracted and converted to ten new (general) patterns using a clusters' merging strategy with highly similar patterns employed in a new post-processing approach useful for multiple locations. Utilizing data from multiple locations in mul-tiple countries ensures a level of generality of the patterns. It also allows comparing the locations. Next to the ten general patterns, some typical patterns are extracted separately for every location. A statistical indices analysis confirms that a complete picture of sub-10-min oscillations needs both statistical indices (quantifying level and variations) and the proposed framework (quantifying patterns). The extracted patterns could be used as a reference for testing/putting requirements on the grid-connected equipment and quantifying the grid's hosting capacity for different types of new distributed generations connected to the grid. The framework is scalable and computationally cheap, making it appropriate for seeking typical patterns in the big data domain. Applying the framework to the much less understood phenomenon will result in providing general knowledge in the field of power quality.
更多查看译文
关键词
Power quality,Fast voltage variations,Seeking patterns,Unsupervised learning,Kernel PCA (KPCA),Time series clustering,Post-processing
AI 理解论文
溯源树
样例
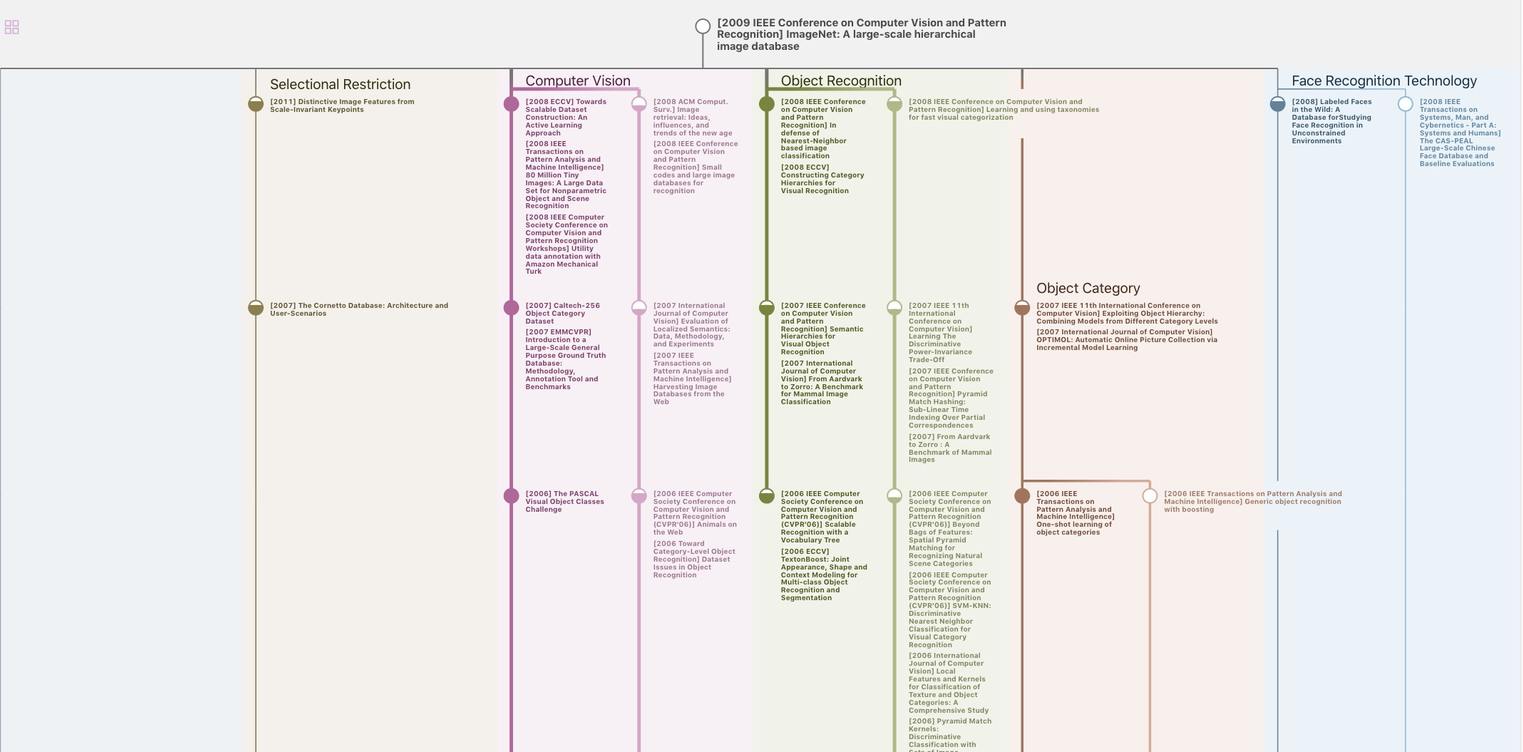
生成溯源树,研究论文发展脉络
Chat Paper
正在生成论文摘要