O-173 Artificial intelligence blastocyst ploidy distinction through morphokinetics data
Human Reproduction(2022)
摘要
Abstract Study question Is it possible to estimate blastocyst ploidy with an artificial intelligence (AI) algorithm built using embryo morphokinetics data? Summary answer AI was able to estimate blastocysts status in euploid or aneuploid with an accuracy of 0.99 (training) and 0.71 and 0.70 AUC (blind-test), respectively. What is known already Morphokinetic parameters are well associated with implantation rates, thus ensuring time-lapse as a useful tool to enhance embryo ranking and selection. However, regarding embryo ploidy status, clinics still rely exclusively on molecular/genetic testing. Our previous studies has indicated that euploid blastocysts are faster at time of pronucleous fading (tPNf) and time to blastulation (tB). Considering the amount of data derived from the morphokinetic annotations and the promising results that AI approach is bringing to the field, this study aims to verify AI accuracy to distinguish euploid and aneuploidy blastocysts using 17 morphokinetics parameters and prospectively compared to embryo biopsy results. Study design, size, duration This is a prospective cohort study including 402 embryos (cultured in a time-lapse incubator, EmbryoscopePlus, Vitrolife) from 140 patients undergoing IVF treatment with preimplantation genetic testing for aneuploidy (NGS platform) after inform consent form signature between July 2019 and September 2021. Morphokinetics annotations were analyzed by AI in an association of the technique of artificial neural networks (ANN) and genetic algorithms (GA) for ploidy assessment. Participants/materials, setting, methods Morphokinetic parameters were manually annotated up to full-expanded blastocyst time. Time intervals and time ratios were calculated, resulting in 17 variables for the IA analysis. Of the 402 embryos data, 252 were randomly divided into training, validating and testing (70%, 15% and 15%, respectively) of the ANN. The remaining 150 data were used for the blind test. The area under the curve (AUC) of the receiver operating characteristic curve was measured to obtain predictive power. Main results and the role of chance From 402 blastocysts biopsied, 185 were euploid and 217 aneuploid. The AI algorithm was trained with 176 embryos (AUC for both euploid and aneuploid= 0.99), tested with 38 embryos (AUC for euploid=0.62 and aneuploid=0.61), validated with 38 embryos (AUC for euploid=0.82 and aneuploid=0.83). For the blind-test 150 embryos were used (AUC for euploid=0.70 and aneuploid=0.71). Blind-test database was checked only after AI algorithm was tested. Limitations, reasons for caution The development of this AI algorithm was built from a single IVF center database. The absence of an extent dataset for the blind-test does not allow us to transpose this algorithm for clinical use at this moment. Wider implications of the findings The use of artificial intelligence for embryo assessment is a promising tool in IVF laboratories. In our model, using only morphokinetics, a considerable predictive power to evaluate euploid (0.71 AUC) and aneuploid (0.70 AUC) embryos was achieved, indicating a potential use as a non-invasive approach for embryo ranking and selection. Trial registration number Not applicable
更多查看译文
AI 理解论文
溯源树
样例
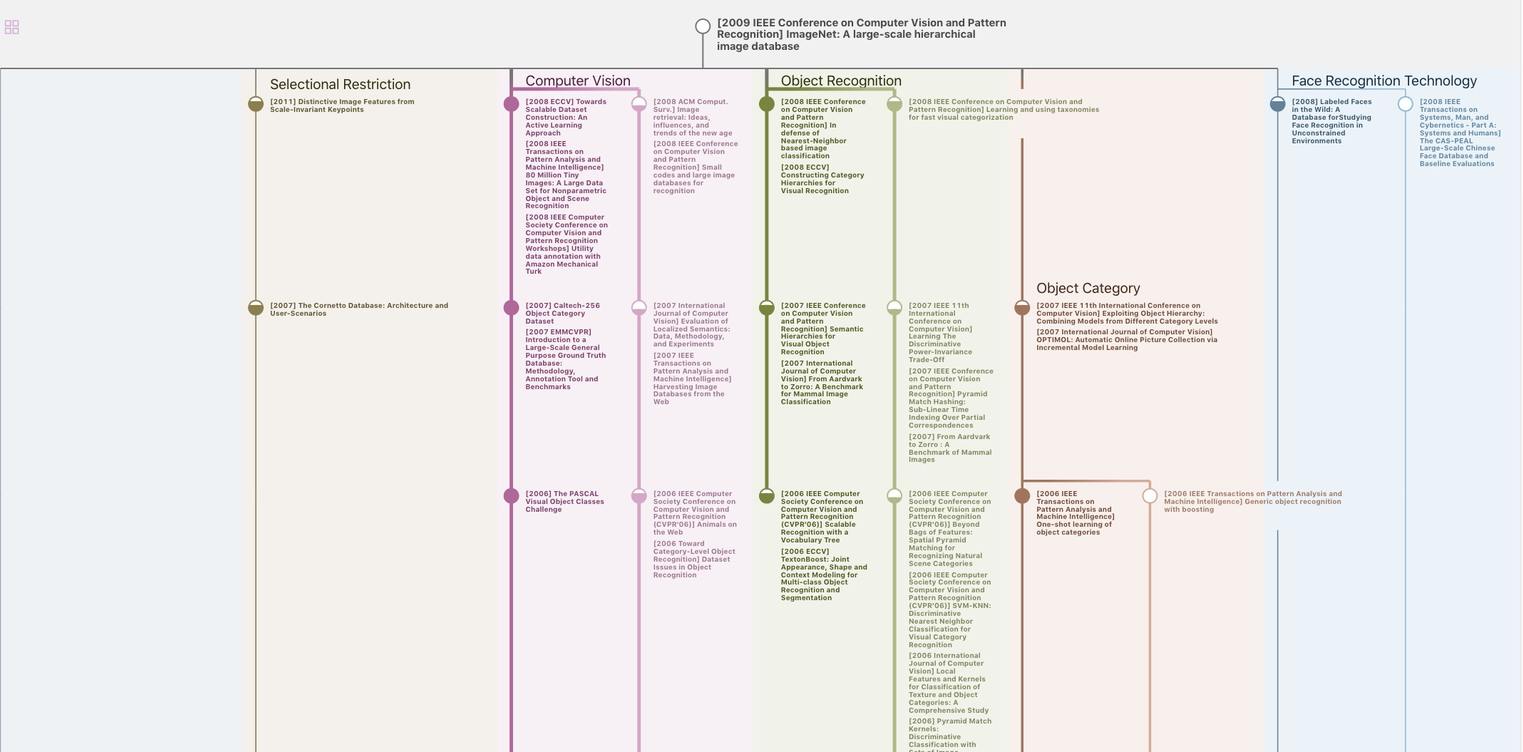
生成溯源树,研究论文发展脉络
Chat Paper
正在生成论文摘要