A Multilevel Hybrid Transmission Network for Infrared and Visible Image Fusion
IEEE TRANSACTIONS ON INSTRUMENTATION AND MEASUREMENT(2022)
摘要
Infrared and visible image fusion aims to generate an image with prominent target information and abundant texture details. Most existing methods generally rely on manually designing complex fusion rules to realize image fusion. Some deep learning fusion networks tend to ignore the correlation between different level features, which may cause loss of intensity information and texture details in the fused image. To overcome these drawbacks, we propose a multilevel hybrid transmission network for infrared and visible image fusion, which mainly contains the multilevel residual encoder module (MREM) and the hybrid transmission decoder module (HTDM). Considering the great difference between infrared and visible images, the MREM with two independent branches is designed to extract abundant features from source images. To avoid complicated fusion strategies, the concatenate convolution is applied to fuse features. Toward utilizing information from source images efficiently, the HTDM is constructed to integrate different level features. Experimental results and analyses on three public datasets demonstrate that our method not only can achieve high-quality image fusion, but also performs better than comparison methods in terms of qualitative and quantitative comparisons. In addition, the proposed method has good real-time performance in infrared and visible image fusion.
更多查看译文
关键词
Image fusion,Feature extraction,Decoding,Transforms,Frequency modulation,Residual neural networks,Learning systems,Decoder module,encoder module,hybrid transmission,image fusion,multilevel
AI 理解论文
溯源树
样例
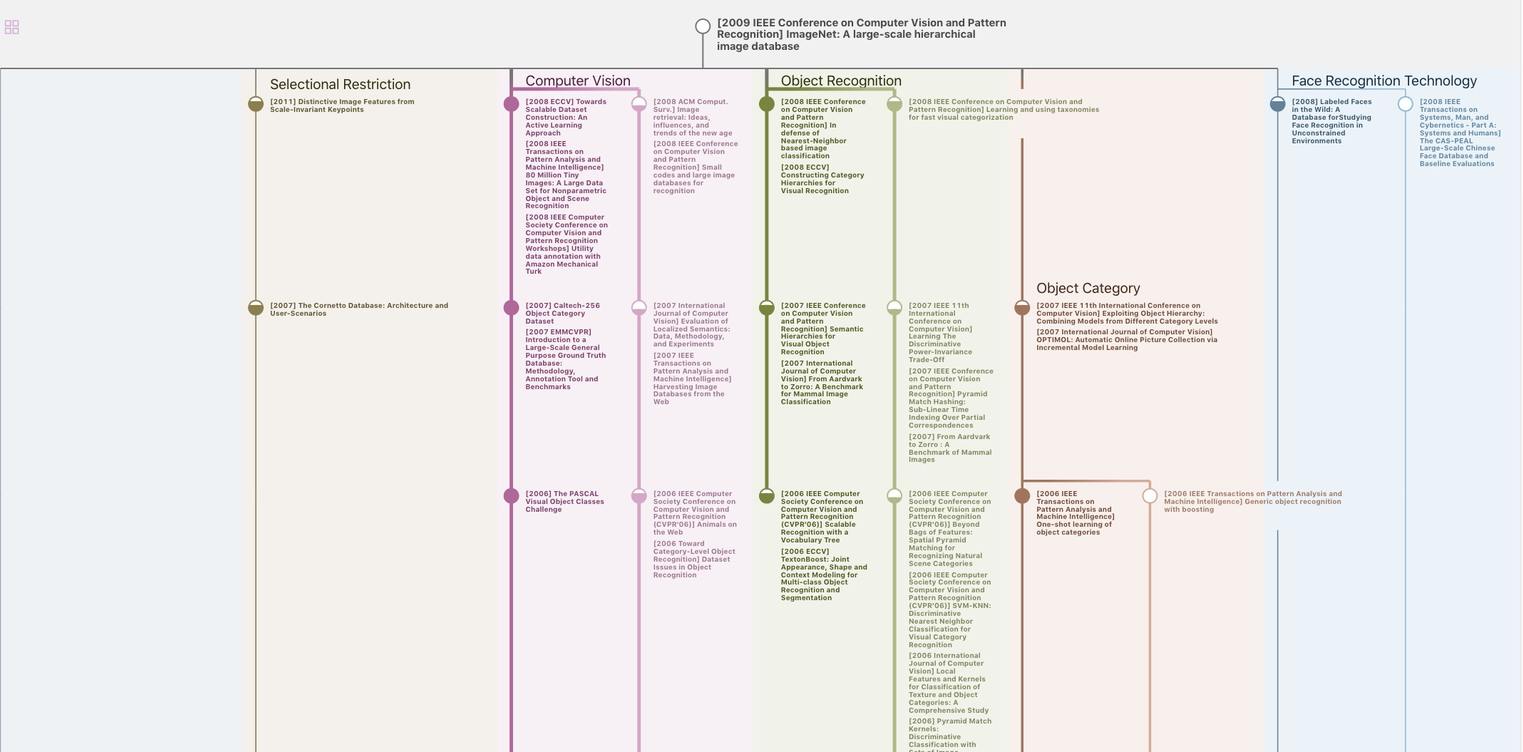
生成溯源树,研究论文发展脉络
Chat Paper
正在生成论文摘要