Multiagent Online Learning in Time-Varying Games
MATHEMATICS OF OPERATIONS RESEARCH(2023)
摘要
We examine the long-run behavior of multiagent online learning in games that evolve over time. Specifically, we focus on a wide class of policies based on mirror descent, and we show that the induced sequence of play (a) converges to a Nash equilibrium in time-varying games that stabilize in the long run to a strictly monotone limit, and (b) it stays asymptotically close to the evolving equilibrium of the sequence of stage games (assuming they are strongly monotone). Our results apply to both gradient- and payoff-based feedback-that is, when players only get to observe the payoffs of their chosen actions.
更多查看译文
关键词
dynamic regret,Nash equilibrium,mirror descent,time-varying games
AI 理解论文
溯源树
样例
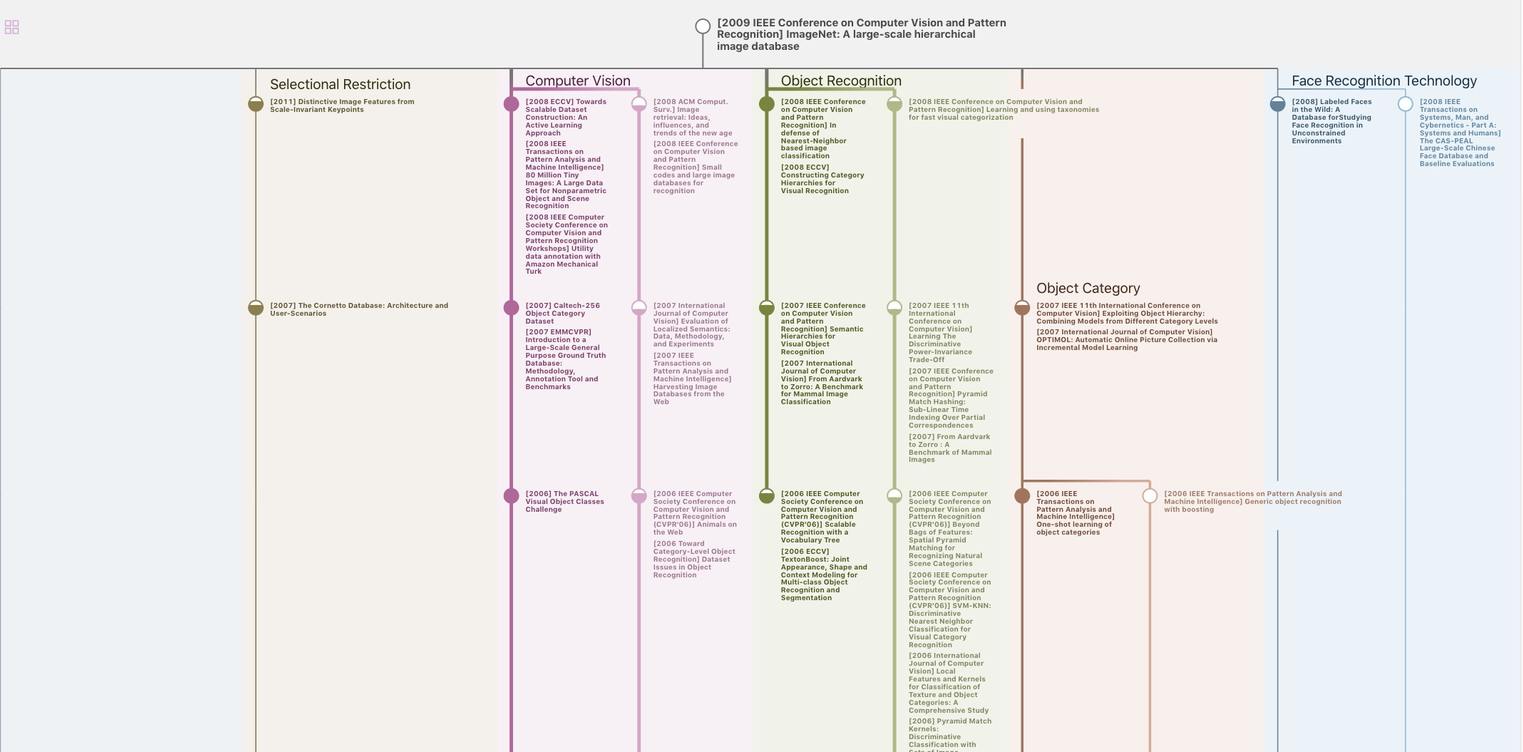
生成溯源树,研究论文发展脉络
Chat Paper
正在生成论文摘要