On the Generalizability of Two-dimensional Convolutional Neural Networks for Fake Speech Detection
International Multimedia Conference(2022)
摘要
BSTRACTThe powerful capabilities of modern text-to-speech methods to produce synthetic computer generated voice, can pose a problem in terms of discerning real from fake audio. In the present work, different pipelines were tested and the best in terms of inference time and audio quality was selected to expand on the real audio of the TIMIT dataset. This led to the creation of a new fake audio detection dataset based on the TIMIT corpus. A range of different audio representations (magnitude spectrogram and energies representations) were studied in terms of performance on both datasets, with the two-dimensional convolutional neural networks trained only on the Fake or Real (FoR) dataset. While there was not a single best representation in terms of performance on both datasets, the Mel spectrogram and Mel energies representations were found to be more robust overall. No performance difference in recognition accuracy was evident during validation, while the two-dimensional convolutional neural network model showed a tendency to under-perform on the test set of the FoR dataset and the synthesized one based on the TIMIT corpus, regardless of the representation used. This fact was corroborated by the data distribution analysis that is presented in the present work.
更多查看译文
AI 理解论文
溯源树
样例
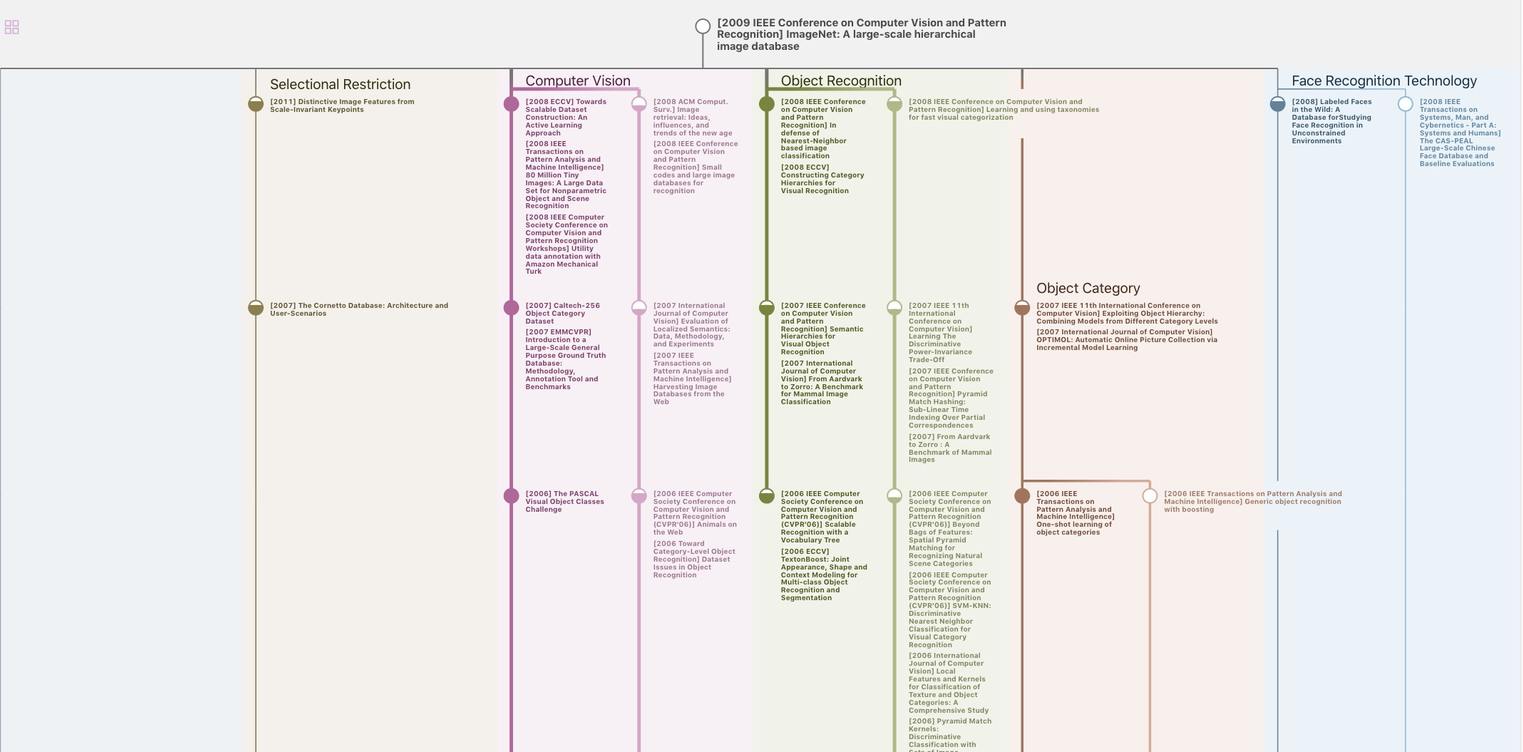
生成溯源树,研究论文发展脉络
Chat Paper
正在生成论文摘要