Fake News Detection Based on Multi-Modal Classifier Ensemble
International Multimedia Conference(2022)
摘要
BSTRACTWith the advent of the era of big data, the ubiquity of multi-modal fake news has increasingly affected information dissemination and consumption. Measurements should be taken to identify multimodal fake news for improving the credibility of news. However, existing single-modal fake news detection models fail to detect fake news based on complete multi-modal information, while multimodal models are often difficult to fully utilize the original information of each single modality to obtain the ultimate accuracy. To tackle above problems, we propose a novel multi-modal fake news detection method, called fake news detection based on multi-modal classifier ensemble, which takes into account the advantages of both single-modal and multi-modal models. Specifically, we design two single-modal classifiers for text and image inputs respectively. We then establish a similarity classifier to calculate the feature similarity over the modalities. We also build an integrity classifier that utilizes integral multi-modal information. Finally, all classifier outputs are integrated with an ensemble learning to increase the classification accuracy. Furthermore, we introduce the center loss, to reduce intra-class variance, which is helpful to achieve higher detection accuracy. The cross-entropy loss is used to maximize the inter-class variations while the center loss is used to minimize the intra-class variations so that the discriminate ability of the learned news features can be enhanced. Experimental results on both Chinese and English datasets demonstrate that the proposed method outperforms the baseline fake news detection approaches.
更多查看译文
AI 理解论文
溯源树
样例
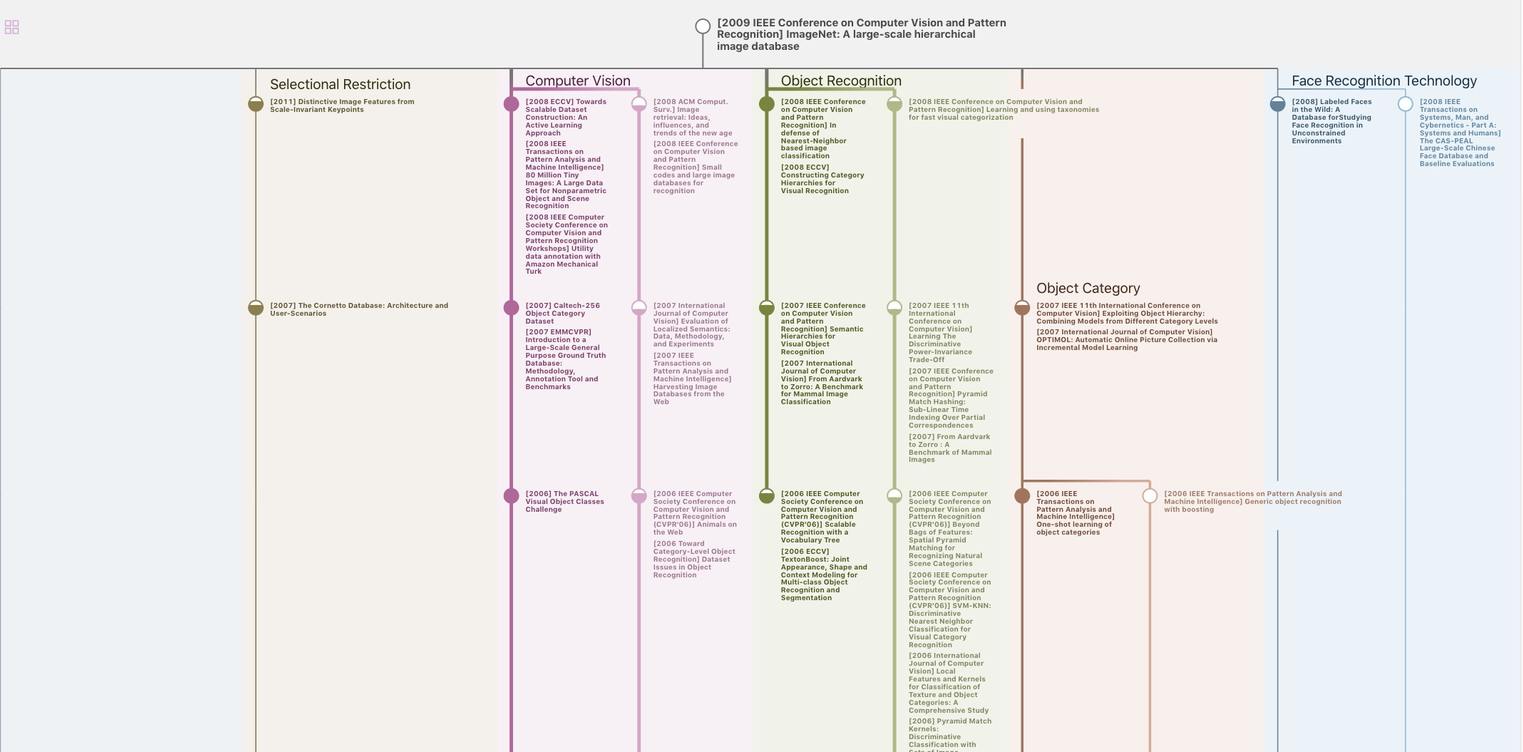
生成溯源树,研究论文发展脉络
Chat Paper
正在生成论文摘要