Sequential Submodular Maximization and Applications to Ranking an Assortment of Products
Economics and Computation(2022)
摘要
ABSTRACTWe introduce and study a variation of the submodular maximization problem motivated by applications in online retail. A platform displays a list of products to a user in response to a search query. The user inspects the first k items in the list for a k chosen at random from a given distribution, and decides whether to purchase an item from that set based on a choice model. The goal of the platform is to maximize the engagement of the shopper defined as the probability of purchase. This problem gives rise to a less-studied variation of submodular maximization in which we are asked to choose an ordering of a set of elements to maximize a linear combination of different submodular functions. First, using a reduction to maximizing submodular functions over matroids, we give an optimal (1-1/e)-approximation for this problem. We then consider a variant in which the platform cares not only about user engagement, but also about diversification across various groups of users, that is, guaranteeing a certain probability of purchase in each group. We characterize the polytope of feasible solutions and give a bi-criteria ((1-1/e)2,(1-1/e)2)-approximation for this problem by rounding an approximate solution of a linear programming relaxation. For rounding, we rely on our reduction and the particular rounding techniques for matroid polytopes. For the special case in which underlying submodular functions are coverage functions -- which is practically relevant in online retail -- we propose an alternative LP relaxation and a simpler randomized rounding for the problem. This approach yields to an optimal bi-criteria (1-1/e,1-1/e)-approximation algorithm for the special case of the problem with coverage functions.
更多查看译文
AI 理解论文
溯源树
样例
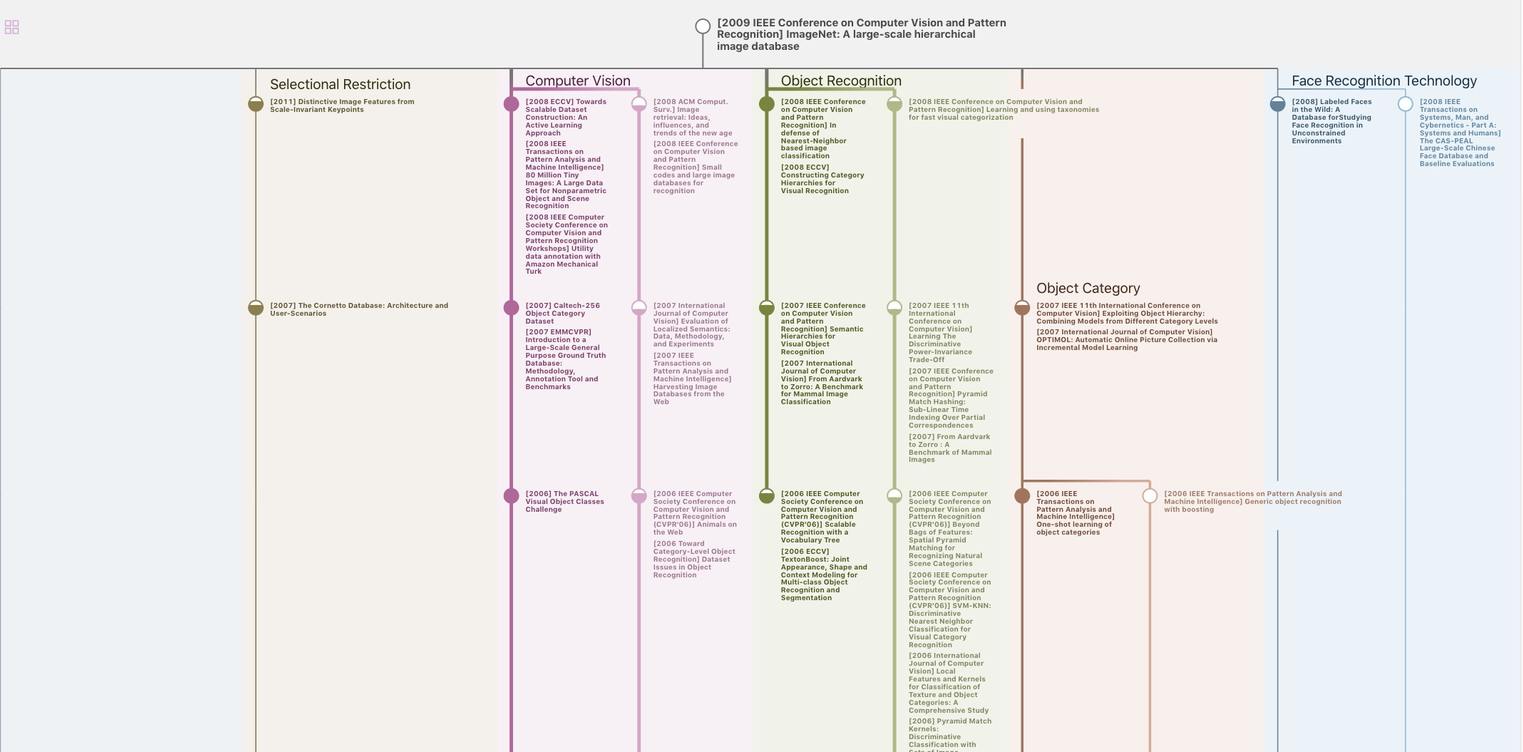
生成溯源树,研究论文发展脉络
Chat Paper
正在生成论文摘要