Testing of a machine learning (ML) model for ability to predict oxaliplatin and bevacizumab (bev) benefit in NRG Oncology/NSABP C-07 and C-08.
Journal of Clinical Oncology(2022)
摘要
3607 Background: Through mining The Cancer Genome Atlas (TCGA) data and a large set of transcriptomes of CRC in GEO, we constructed 15 metagenes reflecting the transcriptomic impact of major driver genes of CRC. Independent of any clinical information, we used metagenes as features to further develop an ML model, then tested it using gene expression (GE) data from C-07 and C-08. Methods: We carried out a prospectively designed and double-blind study to evaluate the clinical utility of the ML model in the adjuvant setting. Samples were classified as Sig+ or Sig-. Association of signatures with recurrence free interval were tested using log-rank test, and significance was set at P<0.05. Cox regression models were used to estimate hazard ratios in univariate and multivariate models and for significance testing in multivariate models. Clinical variables included in multivariate models were nodal status, age, sex, and T stage. Results: We tested the ML model for its ability to predict oxaliplatin benefit in all C-07 pts with available GE data (n=846). Sig+ pts received significant benefit from oxaliplatin (HR=0.68, 95% CI=0.48-0.95, p=0.025) but Sig- did not (Sig- HR=1.05, 95% CI=0.72-1.53, p=0.79), however, the int p value showed only a trend for significance (int p=0.091). Sig+ remained significant for oxaliplatin benefit in multivariate analysis (HR=0.67, 95% CI=0.48-0.95, p=0.024). When we combined all C-07 pts (C-07 FULV-trtd n=298, FLOX n=304) with C-08 FOLFOX-treated pts (n=226) the Sig+ was significantly associated with oxaliplatin benefit (HR=0.65, 95% CI=0.48-0.89, p=0.0065) with a significant int p=0.03. We also tested the signature for association with bev benefit in C-08 (n=438), using a different cut off. Sig+ showed only a trend for an association with bev benefit (HR=0.63, 95% CI=0.35-1.12, p=0.11). To increase the power to detect bev benefit, we also tested the signature for association with bev benefit in all C-08 patients and C-07 pts treated with FLOX. The Sig+ group received significant benefit from bev (HR=0.58, 95% CI=0.36-0.94, p=0.025) but the Sig- group did not (HR=1.02, 95% CI=0.64-1.63, p=0.94), however, the int p was not significant (p=0.101). The model also showed an association with prognosis within the FULV treatment arm in C-07 (HR=1.51, 95% CI=1.07-2.14, p=0.018) and the FOLFOX+bev arm in C-08 (HR=0.55, 95% CI=0.30-1.01, p=0.049). Conclusions: Although our study is not optimally powered, our analyses indicate that the ML model was predictive for oxaliplatin benefit in stage II and III CC and may be useful for detecting bev benefit. Importantly, the Sig- population is candidate for omitting oxaliplatin (de-escalation) in adjuvant setting but will require further validation. Support: PA DOH, U10CA-180868, -180822, -196067, Genentech, Sanofi; NSABP. Clinical trial information: 00096278, 00004931.
更多查看译文
AI 理解论文
溯源树
样例
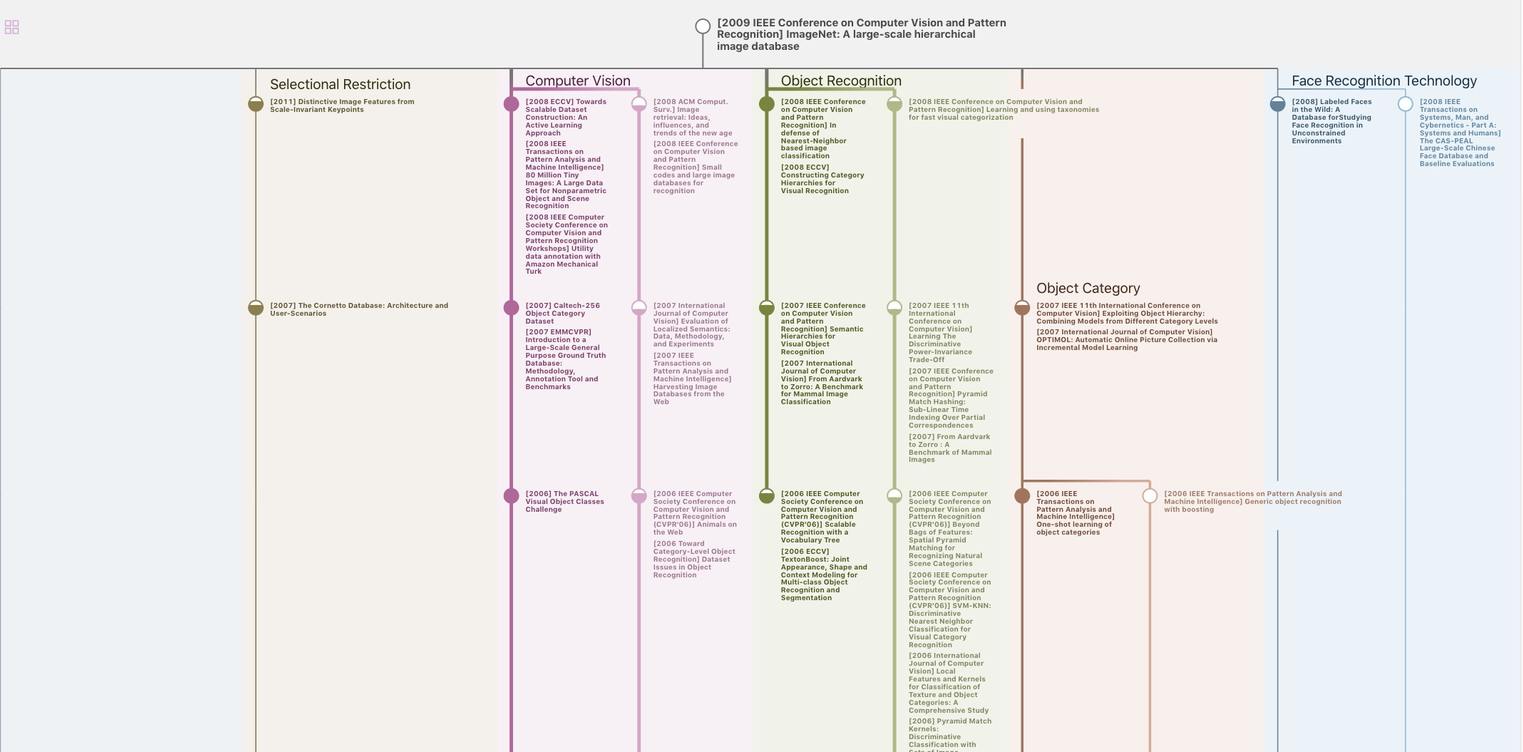
生成溯源树,研究论文发展脉络
Chat Paper
正在生成论文摘要