Rolling Bearing Fault Diagnosis Based on Horizontal Visibility Graph and Graph Neural Networks
2020 International Conference on Sensing, Measurement & Data Analytics in the era of Artificial Intelligence (ICSMD)(2020)
摘要
The automatic extraction and learning features relying on artificial intelligence algorithms replace traditional manual features. More effective feature expression improves the performance of machine fault diagnosis with fewer requirements for labor and expertise. However, the present models only can process the data in Euclidean space. The relations between data points are ignored for a long time, which can play a significant role in distinguishing diverse faults patterns. To combat this issue, a novel model for bearing faults diagnosis is proposed by incorporating the horizontal visibility graph (HVG) and graph neural networks (GNN). In the proposed model, time series is converted to graph retaining invariant dynamic characteristics through the HVG algorithm, and the generated graphs are fed into a designed GNN model for feature learning and faults classification further. Finally, the proposed model is tested on two actual bearing datasets, and it shows state-of-the-art performance in the bearing faults diagnosis. The experimental results demonstrate that extracting relation information using HVG benefits bearing faults diagnosis.
更多查看译文
关键词
rolling bearing,fault diagnosis,horizontal visibility graph (HVG),graph neural networks (GNN)
AI 理解论文
溯源树
样例
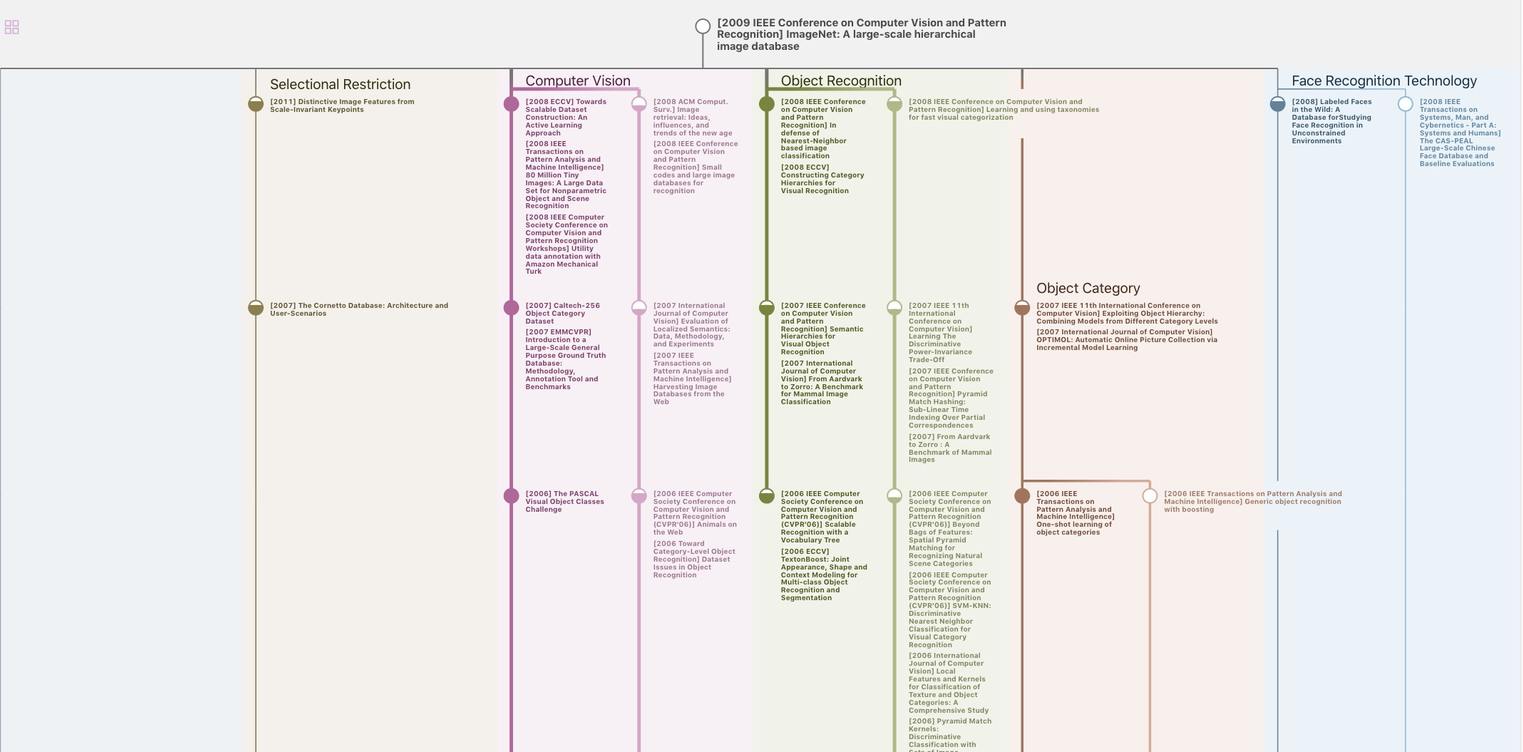
生成溯源树,研究论文发展脉络
Chat Paper
正在生成论文摘要